Deep ensemble transfer learning framework for COVID-19 Arabic text identification via deep active learning and text data augmentation
Multimedia Tools and Applications(2024)
摘要
Since the declaration of COVID-19 as an epidemic by the World Health Organization in September 2019, the task of monitoring and managing the spread of misinformation related to COVID-19 on social media has become increasingly challenging. Particularly, when it comes to Arabic text recognition, tracking and identifying misleading information regarding COVID-19 on social media platforms presents significant difficulties. The detection of such text is crucial in order to safeguard our communities from the dissemination of false rumors and to establish a reliable framework for text detection. This research paper introduces a novel deep ensemble learning framework that aims to recognize ten distinct categories of Arabic text related to COVID-19, including rumors, restrictions, celebrity news, informational news, plans, requests, advice, personal anecdotes, and others. To build our framework, we leverage a dataset called ArCOVID-19Vac (Dataset1), which consists of 10,000 text samples. In addition, the DAL technique is employed to automatically annotate new text samples acquired for Dataset2. To further expand our datasets, we employ back translation and random insertion augmentation strategies, resulting in Datasets3 and Datasets4, each containing 24,000 text samples. By merging the original and augmented datasets, we create Dataset5, which comprises a total of 39,000 text samples. The final text prediction is carried out using three transformer-based BERT models through ensemble transfer learning. Our proposed ensemble framework is evaluated using each dataset independently, and it demonstrates promising results, particularly when utilizing the largest dataset (Dataset5), achieving an accuracy of 93%, precision of 92%, recall of 93%, and an F1-score of 91%. Furthermore, our proposed model exhibits performance improvements of 27%, 18%, 2%, and 1% when utilizing Datasets2, 3, 4, and 5, respectively. The comprehensive experimental results demonstrate that our ensemble framework outperforms other state-of-the-art AI-based models. The encouraging performance of our framework in accurately identifying Arabic text has the potential to enhance decision-making processes regarding the identification of misleading information and to facilitate the development of strategies to combat such issues in the future.
更多查看译文
关键词
COVID-19,Arabic text identification,Ensemble transfer learning,Text data augmentation,Deep active learning (DAL), Data mining
AI 理解论文
溯源树
样例
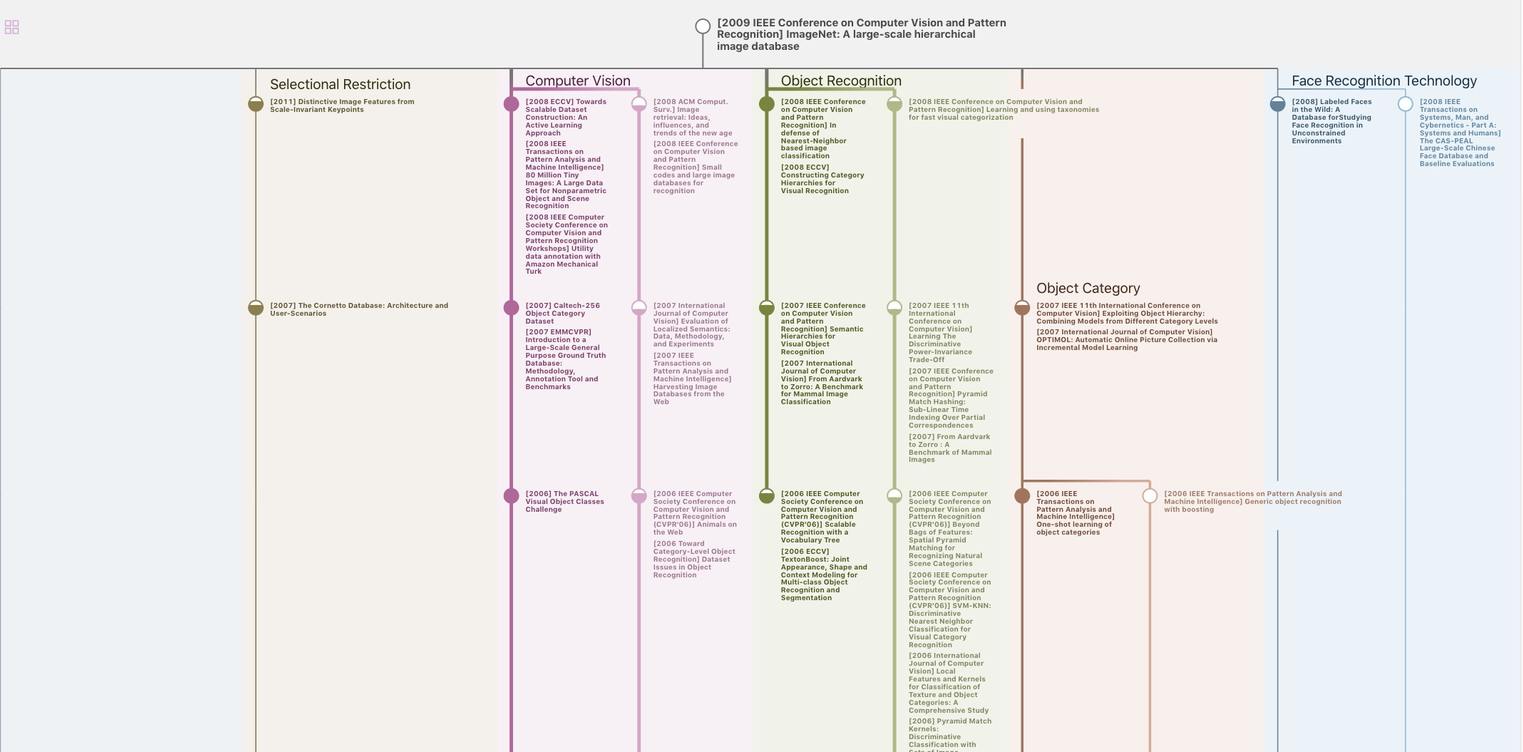
生成溯源树,研究论文发展脉络
Chat Paper
正在生成论文摘要