Predicting ride-hailing passenger demand: A POI-based adaptive clustering federated learning approach
FUTURE GENERATION COMPUTER SYSTEMS-THE INTERNATIONAL JOURNAL OF ESCIENCE(2024)
摘要
Passenger demand prediction is a key task for online ride -hailing platforms to optimize their resource allocation and service quality. However, centralized data collection and mining of massive passengers' travel data expose serious privacy and security risks. To address this challenge, we propose a POI -based Adaptive Clustering Federated Learning with Spatio-Temporal Graph Attention Gate Recurrent Unit Networks (CFL-STG) algorithm that achieves accurate passenger demand prediction while protecting passenger privacy. Firstly, we propose a localized data storage mechanism for preserving data privacy and facilitating federated learning among different regions. Then, to tackle the statistical heterogeneity of data in federated learning, we introduce a clustering strategy that groups the regions based on their Point of Interest (POI) features, which reflect their demand patterns. For each group, we design a Spatio-Temporal Graph Attention Gate Recurrent Unit Network (STG) as a local model that can capture the spatio-temporal dynamics and inter -regional influence of POI features on passenger demand. Experiments on real data show that the proposed framework outperforms the existing federated learning models and common centralized data mining methods in terms of demand prediction performance and learning efficiency.
更多查看译文
关键词
Demand prediction,Clustering federated learning,Spatial -temporal correlations,POI features,Graph attention
AI 理解论文
溯源树
样例
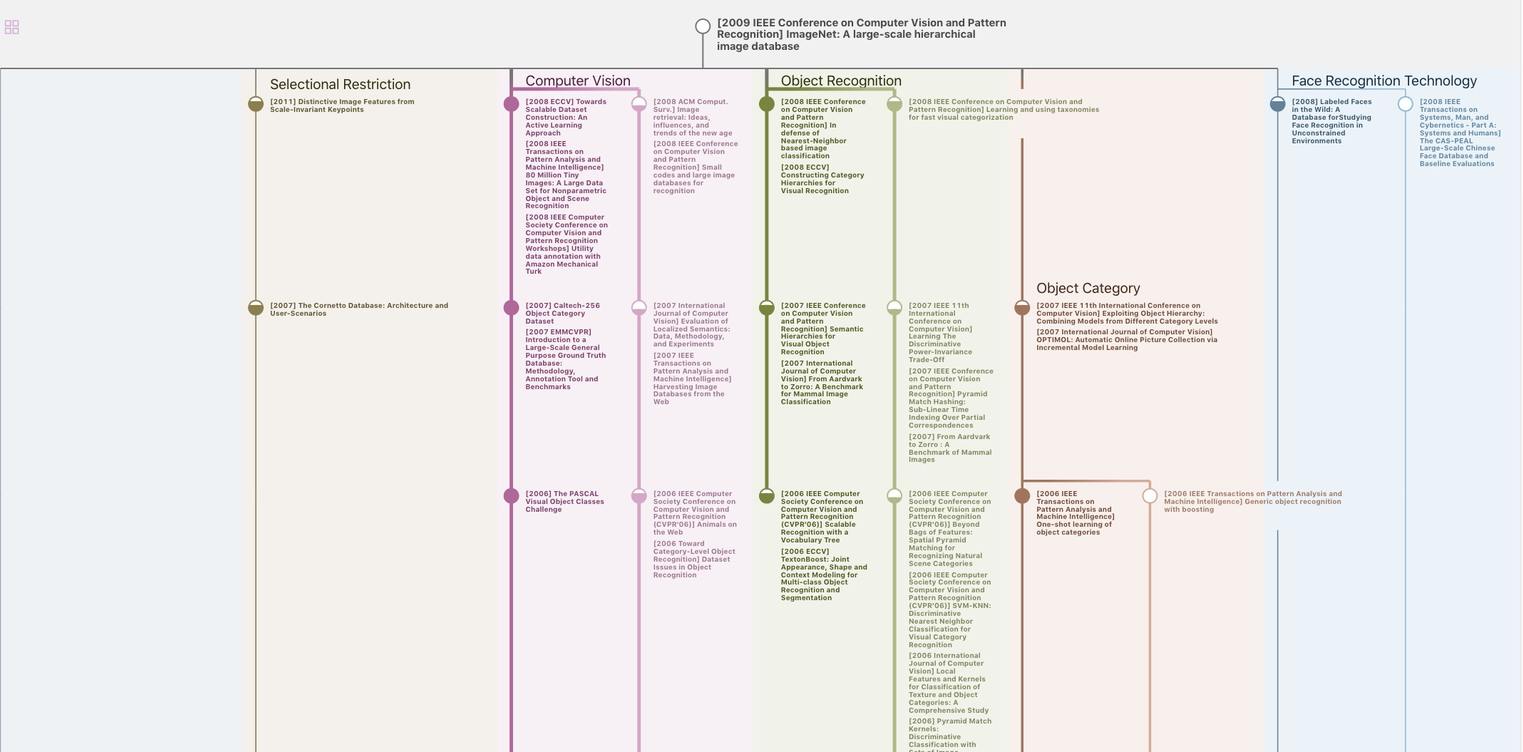
生成溯源树,研究论文发展脉络
Chat Paper
正在生成论文摘要