Comparative analysis of early versus late feeding after an esophagogastroduodenoscopic intervention: Meta-analysis
iGIE(2024)
Abstract
Background & Aims
The right time to re-start feeding after esophagogastroduodenoscopic (EGD interventions is not well established. However, impaired nutrition from prolonged fasting may lead to unfavorable clinical outcomes. Herein, we performed a systematic review and meta-analysis to study the impact of early feeding (within 24 hours) vs. late feeding (more than 24 hours) in patients undergoing acute therapeutic EGD interventions.
Methods
Multiple databases, including Medline, Scopus, and Embase, were searched (in May 2022) using specific terms for studies evaluating the outcomes of early vs. late feeding after EGD interventions. Outcomes of interest were early rebleed (< 7 days), late rebleed (> 7 days), mortality rates, length of hospital stay, and rate of blood transfusion. Standard meta-analysis methods were employed using the random-effects model. I2% heterogeneity was used to assess the heterogeneity. The GRADE working group approach was used to assess the certainty of evidence.
Results
Eight studies (813 patients) were included in the final analysis. The ‘Early feeding’ cohort included 411 patients, 31% females, with a mean age of 58 years, and the ‘late feeding’ cohort included 402 patients, 26.4% females, with a mean age of 57 years. Four studies (283 patients) evaluated patients with band ligation/sclerotherapy in acute variceal bleeding (224 patients), two studies (309 patients) with endoscopic treatment of peptic ulcer bleeding, and two studies in endoscopic mucosal dissection for gastric mucosal epithelial neoplasia. The pooled risk ratio (RR) of early rebleed in ‘early feeding’ vs. ‘late feeding’ was 1.6 (95% confidence interval (CI) [0.7-3.7; I2=0%, p=0.2]. Similarly, the RR of late rebleed was 0.9 [0.4-2.3; I2=0%, p= 0.9]. The pooled RR of total rebleed was 1.2 [0.7-2.2; I2=0%, p=0.3]. The pooled RR of mortality between the ‘early feeding’ and ‘late feeding’ groups was 0.6 [0.3-1.2; I2=0%, p=0.16]. The Pooled rate of standard difference of mean length of hospital stay was -1.184 (-1.5 - -0.81, I2 = 92%, p=0.00) between early and late feeding groups. The Pooled rate of mean difference in blood transfusion between early and late feeding groups was 0.1 [-0.4 - 0.41; I2= 77.6%, p=0.96].
Conclusion
Our meta-analysis demonstrates early enteral feeding within 24 hours does not appear to have a higher risk of rebleeding and mortality than delayed enteral feeding in patients undergoing EGD therapeutic interventions. However, early feeding is associated with a shorter length of hospital stay compared to late feeding.
MoreTranslated text
Key words
Early feed,late feed,endoscopic intervention,post-procedural bleeding,endoscopic mucosal dissection
AI Read Science
Must-Reading Tree
Example
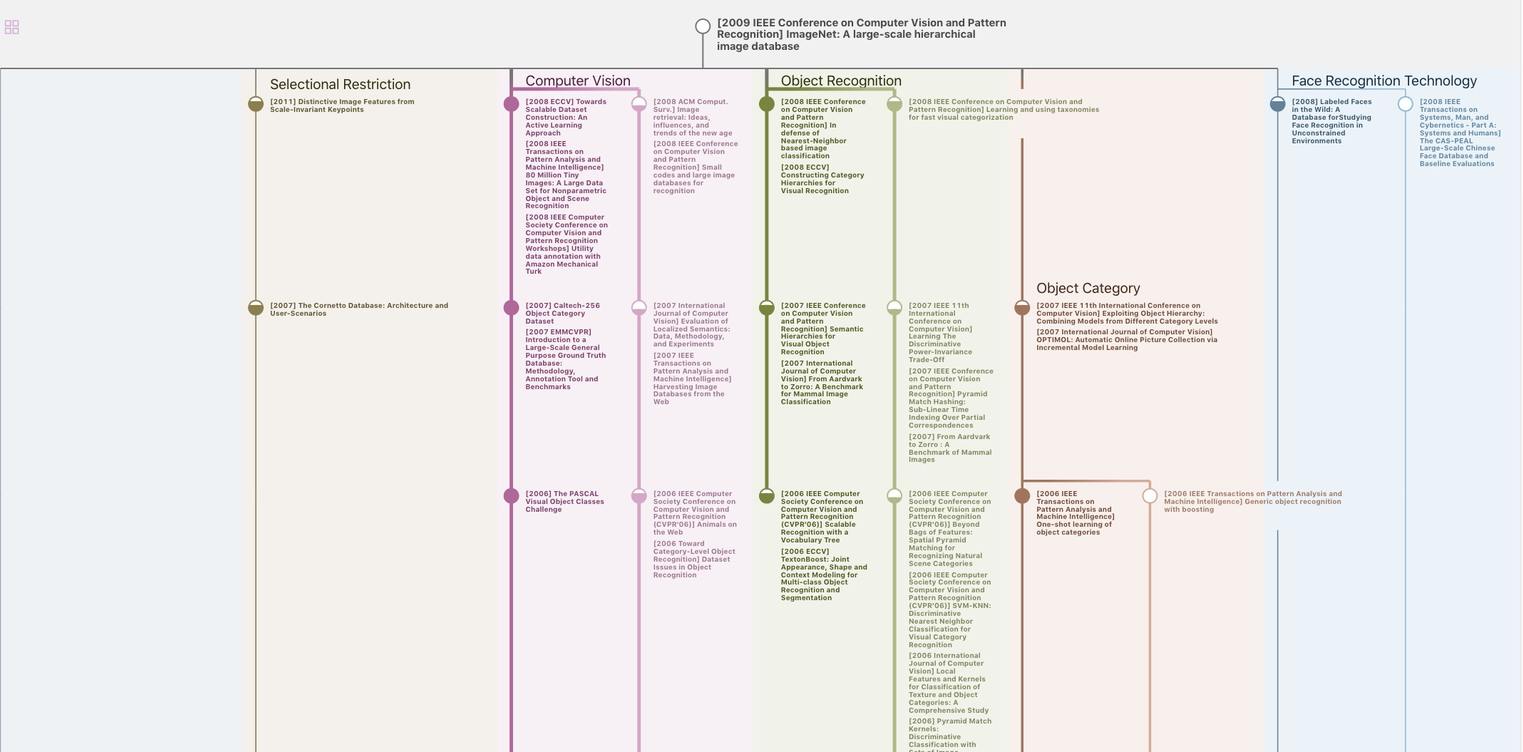
Generate MRT to find the research sequence of this paper
Chat Paper
Summary is being generated by the instructions you defined