Machine Learning-Based Discrimination of Cardiovascular Outcomes in Patients With Hypertrophic Cardiomyopathy
JACC: Asia(2024)
摘要
Background
Current risk stratification strategies for patients with hypertrophic cardiomyopathy (HCM) are limited to traditional methodologies.
Objectives
The authors aimed to establish machine learning (ML)-based models to discriminate major cardiovascular events in patients with HCM.
Methods
We enrolled consecutive HCM patients from 2 tertiary referral centers and used 25 clinical and echocardiographic features to discriminate major adverse cardiovascular events (MACE), including all-cause death, admission for heart failure (HF-adm), and stroke. The best model was selected for each outcome using the area under the receiver operating characteristic curve (AUROC) with 20-fold cross-validation. After testing in the external validation cohort, the relative importance of features in discriminating each outcome was determined using the SHapley Additive exPlanations (SHAP) method.
Results
In total, 2,111 patients with HCM (age 61.4 ± 13.6 years; 67.6% men) were analyzed. During the median 4.0 years of follow-up, MACE occurred in 341 patients (16.2%). Among the 4 ML models, the logistic regression model achieved the best AUROC of 0.800 (95% CI: 0.760-0.841) for MACE, 0.789 (95% CI: 0.736-0.841) for all-cause death, 0.798 (95% CI: 0.736-0.860) for HF-adm, and 0.807 (95% CI: 0.754-0.859) for stroke. The discriminant ability of the logistic regression model remained excellent when applied to the external validation cohort for MACE (AUROC = 0.768), all-cause death (AUROC = 0.750), and HF-adm (AUROC = 0.806). The SHAP analysis identified left atrial diameter and hypertension as important variables for all outcomes of interest.
Conclusions
The proposed ML models incorporating various phenotypes from patients with HCM accurately discriminated adverse cardiovascular events and provided variables with high importance for each outcome.
更多查看译文
关键词
heart failure,hypertrophic cardiomyopathy,machine learning,prediction model,prognosis
AI 理解论文
溯源树
样例
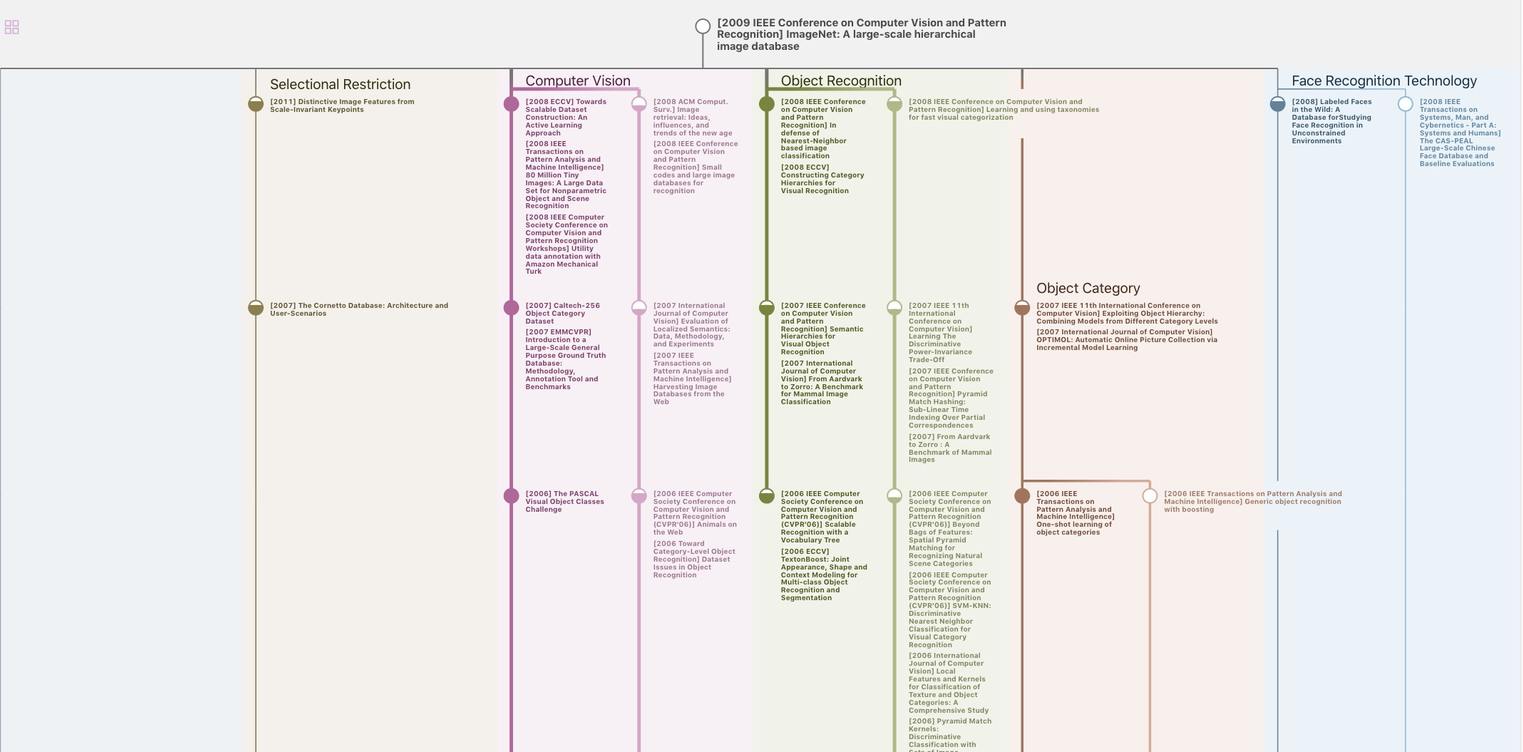
生成溯源树,研究论文发展脉络
Chat Paper
正在生成论文摘要