A variance deconvolution estimator for efficient uncertainty quantification in Monte Carlo radiation transport applications
Journal of Quantitative Spectroscopy and Radiative Transfer(2024)
摘要
Monte Carlo simulations are at the heart of many high-fidelity simulations and analyses for radiation transport systems. As is the case with any complex computational model, it is important to propagate sources of input uncertainty and characterize how they affect model output. Unfortunately, uncertainty quantification (UQ) is made difficult by the stochastic variability that Monte Carlo transport solvers introduce. The standard method to avoid corrupting the UQ statistics with the transport solver noise is to increase the number of particle histories, resulting in very high computational costs. In this contribution, we propose and analyze a sampling estimator based on the law of total variance to compute UQ variance even in the presence of residual noise from Monte Carlo transport calculations. We rigorously derive the statistical properties of the new variance estimator, compare its performance to that of the standard method, and demonstrate its use on neutral particle transport model problems involving both attenuation and scattering physics. We illustrate, both analytically and numerically, the estimator’s statistical performance as a function of available computational budget and the distribution of that budget between UQ samples and particle histories. We show analytically and corroborate numerically that the new estimator is unbiased, unlike the standard approach, and is more accurate and precise than the standard estimator for the same computational budget.
更多查看译文
关键词
Uncertainty quantification,Monte Carlo radiation transport,Stochastic solvers
AI 理解论文
溯源树
样例
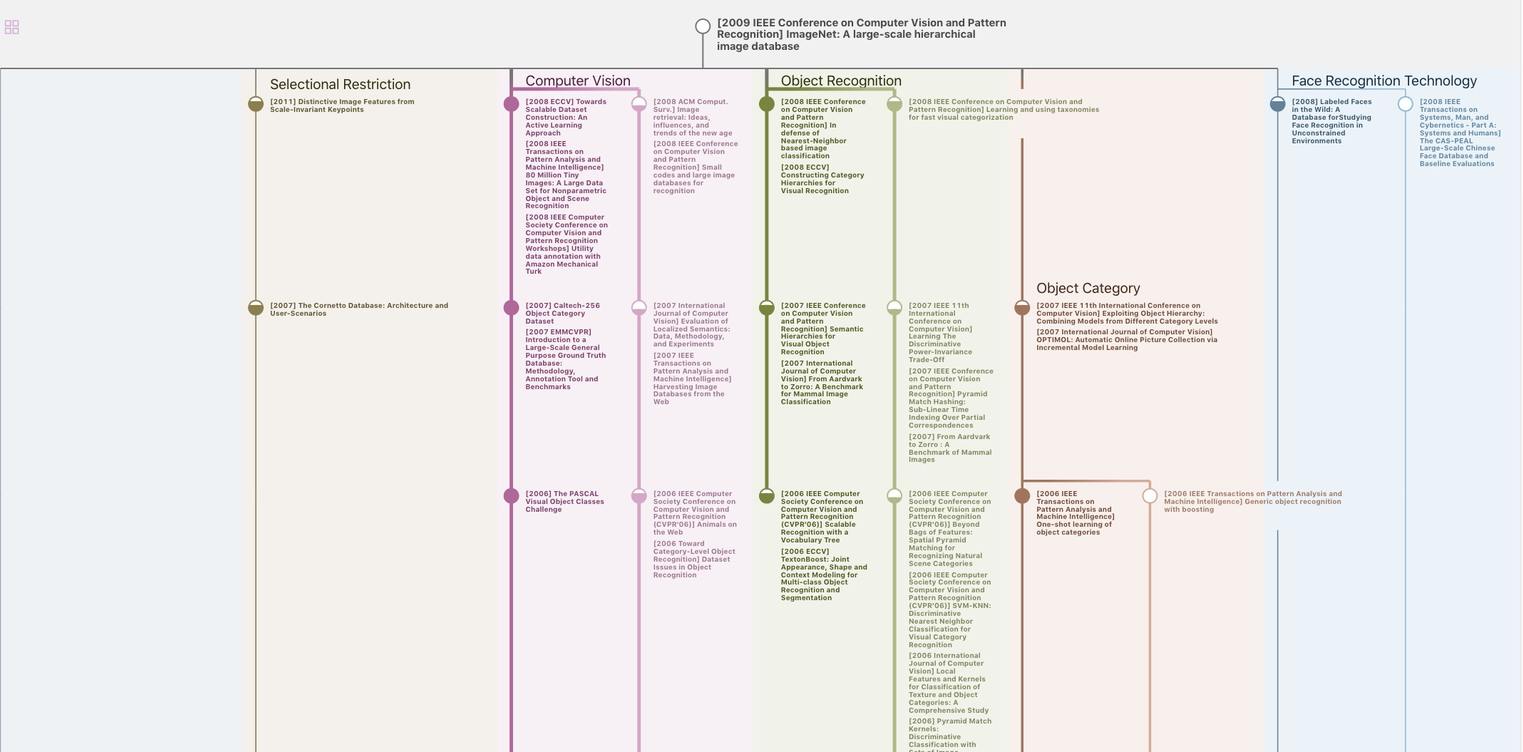
生成溯源树,研究论文发展脉络
Chat Paper
正在生成论文摘要