Tolerant Self-Distillation for image classification
Neural Networks(2024)
摘要
Deep neural networks tend to suffer from the overfitting issue when the training data are not enough. In this paper, we introduce two metrics from the intra-class distribution of correct-predicted and incorrect-predicted samples to provide a new perspective on the overfitting issue. Based on it, we propose a knowledge distillation approach without pretraining a teacher model in advance named Tolerant Self-Distillation (TSD) for alleviating the overfitting issue. It introduces an online updating memory and selectively stores the class predictions of the samples from the past iterations, making it possible to distill knowledge across the iterations. Specifically, the class predictions stored in the memory bank serve as the soft labels for supervising the samples from the same class for the current iteration in a reverse way, i.e. the correct-predicted samples are supervised with the incorrect predictions while the incorrect-predicted samples are supervised with the correct predictions. Consequently, the premature convergence issue caused by the over-confident samples would be mitigated, which helps the model to converge to a better local optimum. Extensive experimental results on several image classification benchmarks, including small-scale, large-scale, and fine-grained datasets, demonstrate the superiority of the proposed TSD.
更多查看译文
关键词
Overfitting,Self-Distillation,Tolerant,Deep Learning
AI 理解论文
溯源树
样例
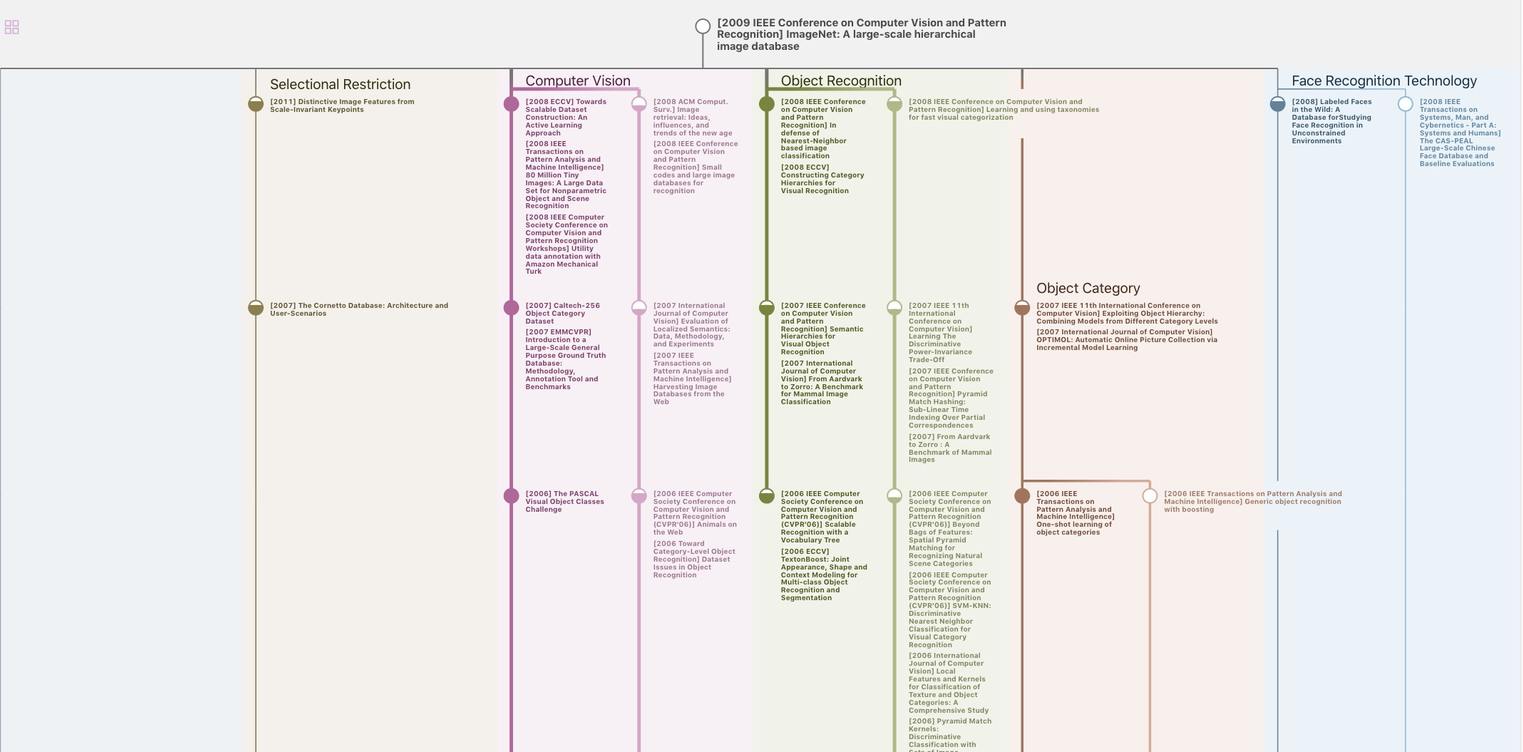
生成溯源树,研究论文发展脉络
Chat Paper
正在生成论文摘要