Robust multi-view learning via M-estimator joint sparse representation
PATTERN RECOGNITION(2024)
摘要
Recently, multi-view learning has achieved extraordinary success in many research areas such as pattern recognition and data mining. Most existing multi-view methods mainly focus on exploring the correlation information between different views and their performance may severely degrade in the presence of heavy noises and outliers. In this paper, we put forward a robust multi-view joint sparse representation (RMJSR) method for multi-view learning. Firstly, we design a novel multi-view Cauchy estimator based loss function originating from robust statistics to address complex noises and outliers in reality. Based on this, we leverage the l(1,q) norm to enhance our model by encouraging the learned representation of multiple views to share the same sparsity pattern. Secondly, to explore the optimal solution for the RMJSR model, we devise an effective optimization algorithm based on the half-quadratic (HQ) theory and the alternating direction method of multipliers (ADMM) framework. Thirdly, we provide the theoretical guarantee for revealing the theoretical condition for the success of the proposed method. Further, we have also provided extensive analysis of the proposed method, including the optimality condition, convergence analysis, and complexity analysis. Extensive experimental results validate the effectiveness and robustness of the proposed method in comparison with state-of-the-art competitors. The source code is available at https://github.com/Huyutao7/RMJSRC.
更多查看译文
关键词
Robust multi-view learning,Joint sparse representation,M-estimator
AI 理解论文
溯源树
样例
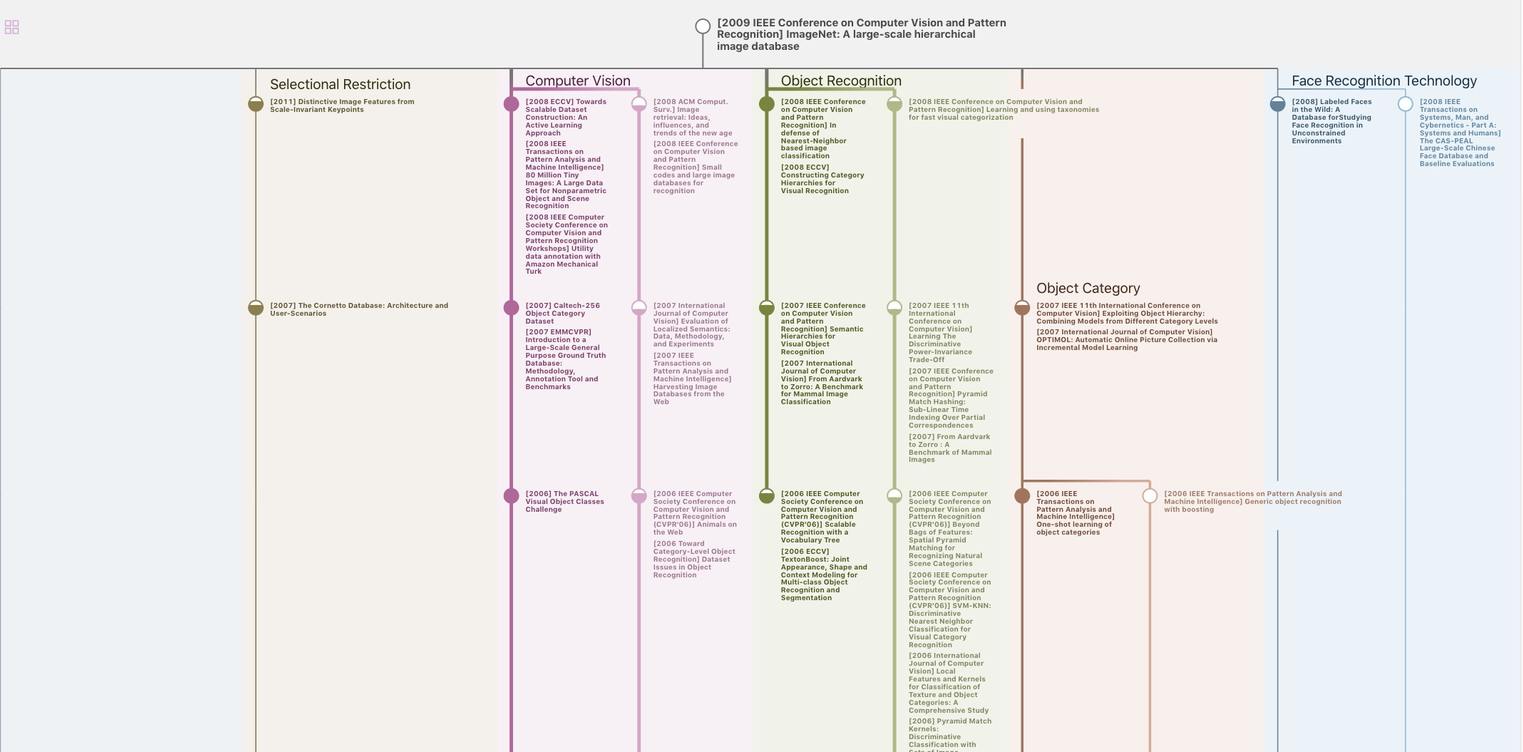
生成溯源树,研究论文发展脉络
Chat Paper
正在生成论文摘要