SPACE: Senti-Prompt As Classifying Embedding for sentiment analysis
Pattern Recognition Letters(2024)
摘要
In natural language processing, the general approach to sentiment analysis involves a pre-training and fine-tuning paradigm using pre-trained language models combined with classifier models. Recently, numerous studies have applied prompts not only to downstream generation but also to classification tasks as well. However, to fully utilize the advantages of prompts and incorporate the context-dependent meaning of class representations into the prompts, it is necessary for the prompts to be learned similarly to the sentiment representations of each class. To achieve this, We introduce a novel method to learn soft prompts during fine-tuning. In this method, the class prompts are initialized with sentiment-related embeddings and are trained by a denoising task, which replaces them with masked tokens, just like the conventional masked language model (MLM) approach. Furthermore, a novel attention pattern is designed to tune attention between class prompts effectively. As a result, we demonstrate that our approach outperforms state-of-the-art models through experiments on four common datasets, achieving superior performance on sentiment analysis.
更多查看译文
关键词
Sentiment analysis,Prompt-tuning,Representation learning,Attention pattern
AI 理解论文
溯源树
样例
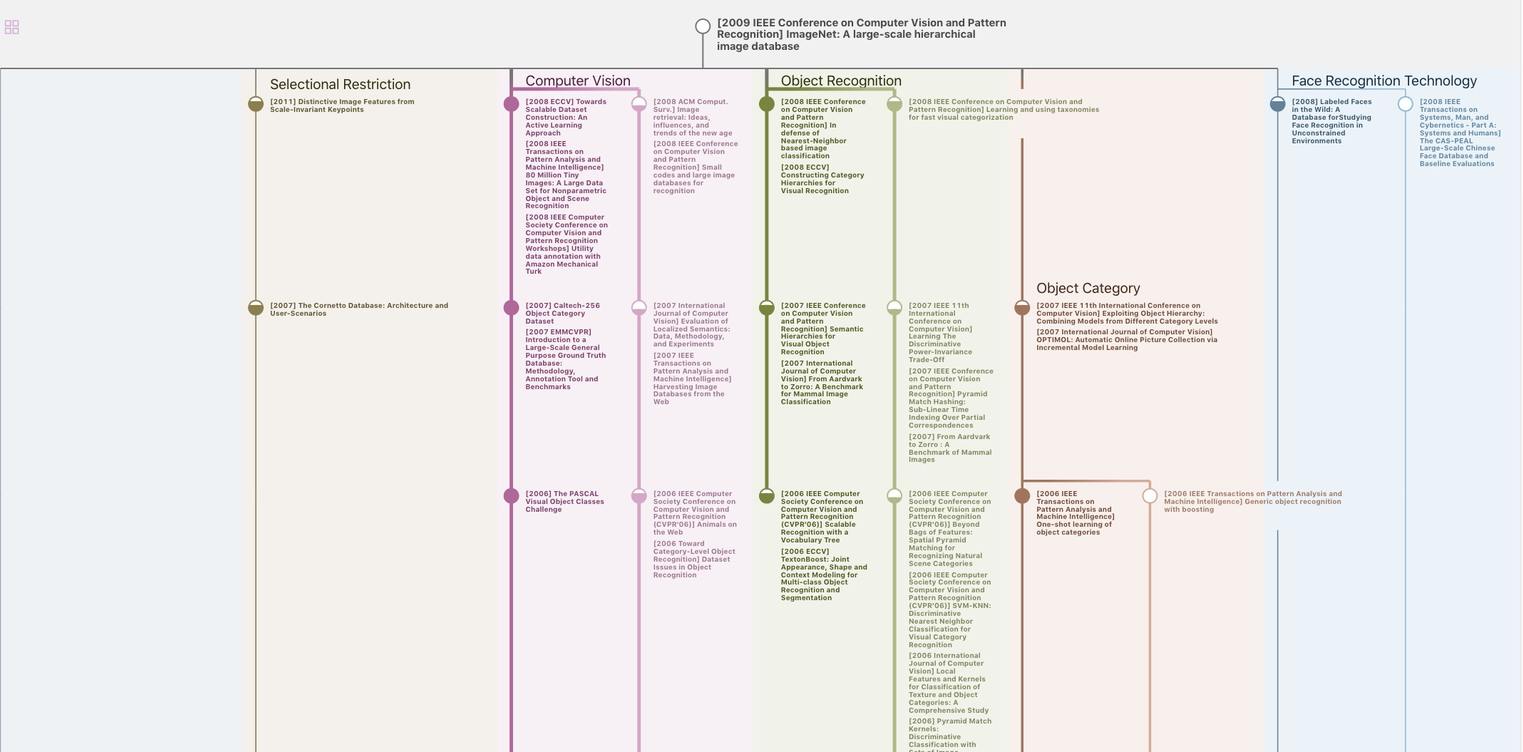
生成溯源树,研究论文发展脉络
Chat Paper
正在生成论文摘要