Degradation path approximation for remaining useful life estimation
ADVANCED ENGINEERING INFORMATICS(2024)
摘要
Modern industry systems usually need to employ remaining useful life (RUL) prediction techniques to monitor the health status of equipment or components. Data -driven RUL prediction methods mainly include machinelearning -based and statistical -model -based methods. However, most machine -learning -based methods rely on amounts of multi -sensor monitoring data. Statistical -model -based methods adopt statistical models to predict the degradation path of the health indicator, which requires model construction and mathematical derivation, relying on extensive expert knowledge. Therefore, we propose a highly easy -to -understand and brand-new solution way for the data -driven RUL prediction, Degradation Path Approximation (DPA). DPA is expertknowledge -free and can perform excellently in less degradation data, even a single historical degradation path. In DPA, the weight, translation, and compensation variables are proposed to approximate the actual degradation path of the health indicator to estimate RUL. We theoretically analyzed and proved the feasibility of DPA. A series of comparative and ablation experiments on Li -ion prismatic cells, milling cutters, 18650 Li -ion batteries, and IGBT are conducted to demonstrate the superiority of DPA and the importance of DPA variables, respectively. DPA performed best, with the prediction accuracy of 77.1%, 96.0%, 72.4%, and 71.9% in four cases. Furthermore, to figure out why DPA can achieve excellent RUL prediction performance, we conducted interpretability analyses by the influence of DPA variables on the predicted degradation paths. Code and data are available at Github.
更多查看译文
关键词
Remaining useful life,Degradation path,Health indicator,Data-driven prognostic,RUL prediction
AI 理解论文
溯源树
样例
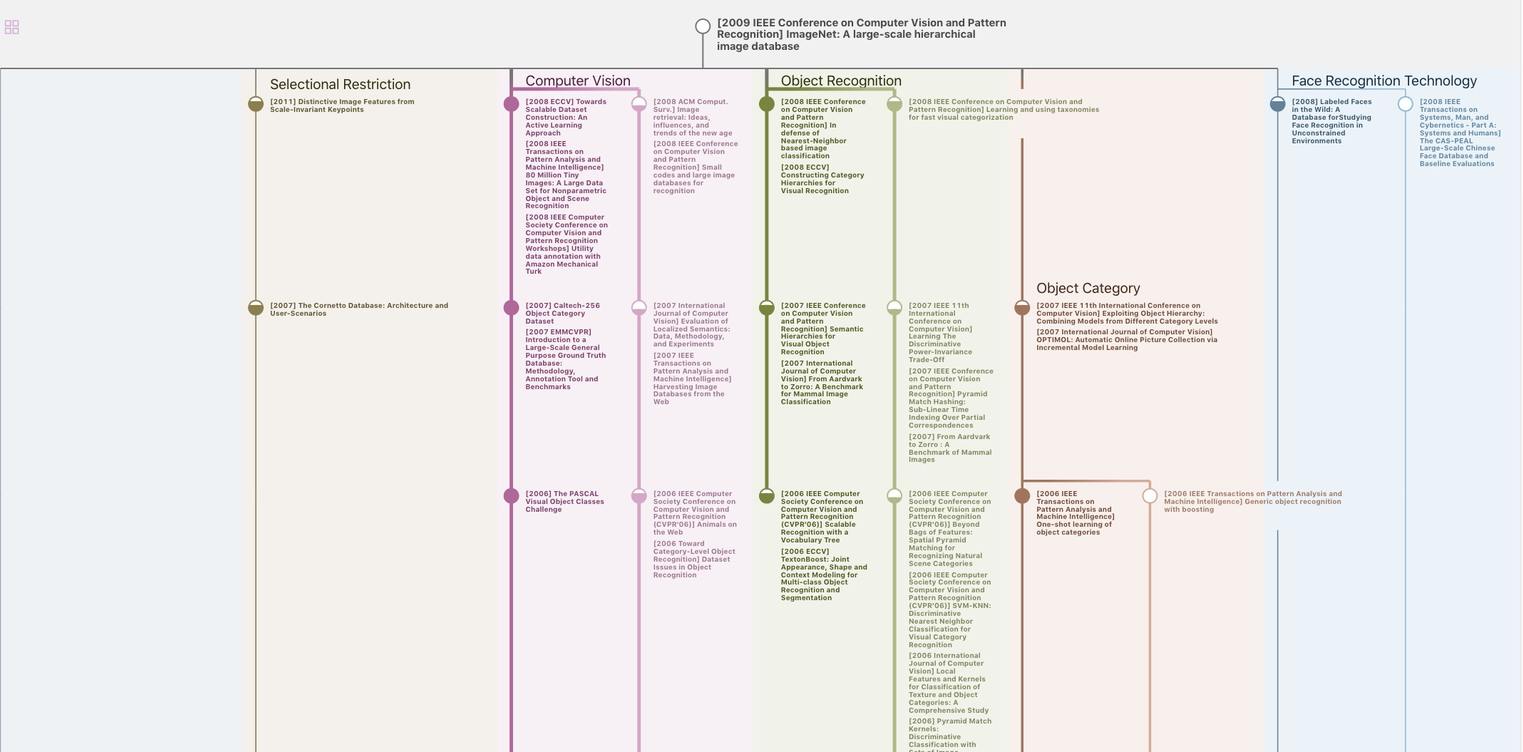
生成溯源树,研究论文发展脉络
Chat Paper
正在生成论文摘要