On-policy and Off-Policy Q-learning Strategies for Spacecraft Systems: an Approach for Time-Varying Discrete-Time Without Controllability Assumption of Augmented System
Aerospace science and technology(2024)
摘要
This article investigates two On-policy and Off-policy Q-learning algorithms for time-varying linear discrete-time systems (DTSs) in the presence of complete dynamic uncertainties. To handle the challenge of time-varying description, the lifting method is employed to transform the original time-varying linear DTS into time-invariant linear DTS in the absence of the conventional controllability condition, which affects to the convergence of traditional Q-learning algorithms. Based on theoretical analysis of the structure in the obtained time-invariant linear DTS, On-policy and Off-policy algorithms are proposed to guarantee the convergence of Q-learning algorithms. Both On-policy and Off-policy Q-learning algorithms guarantee model-free consideration under the data collection. Especially, the Off-policy technique is able to develop the algorithm with high data efficiency because the collected data can be utilized again after each iteration. Finally, the simulation results of two-dimensional systems and spacecraft control systems are presented to validate the effectiveness of the two proposed control schemes.
更多查看译文
关键词
Linear periodic systems,Time-varying systems,Q-learning,Spacecraft control
AI 理解论文
溯源树
样例
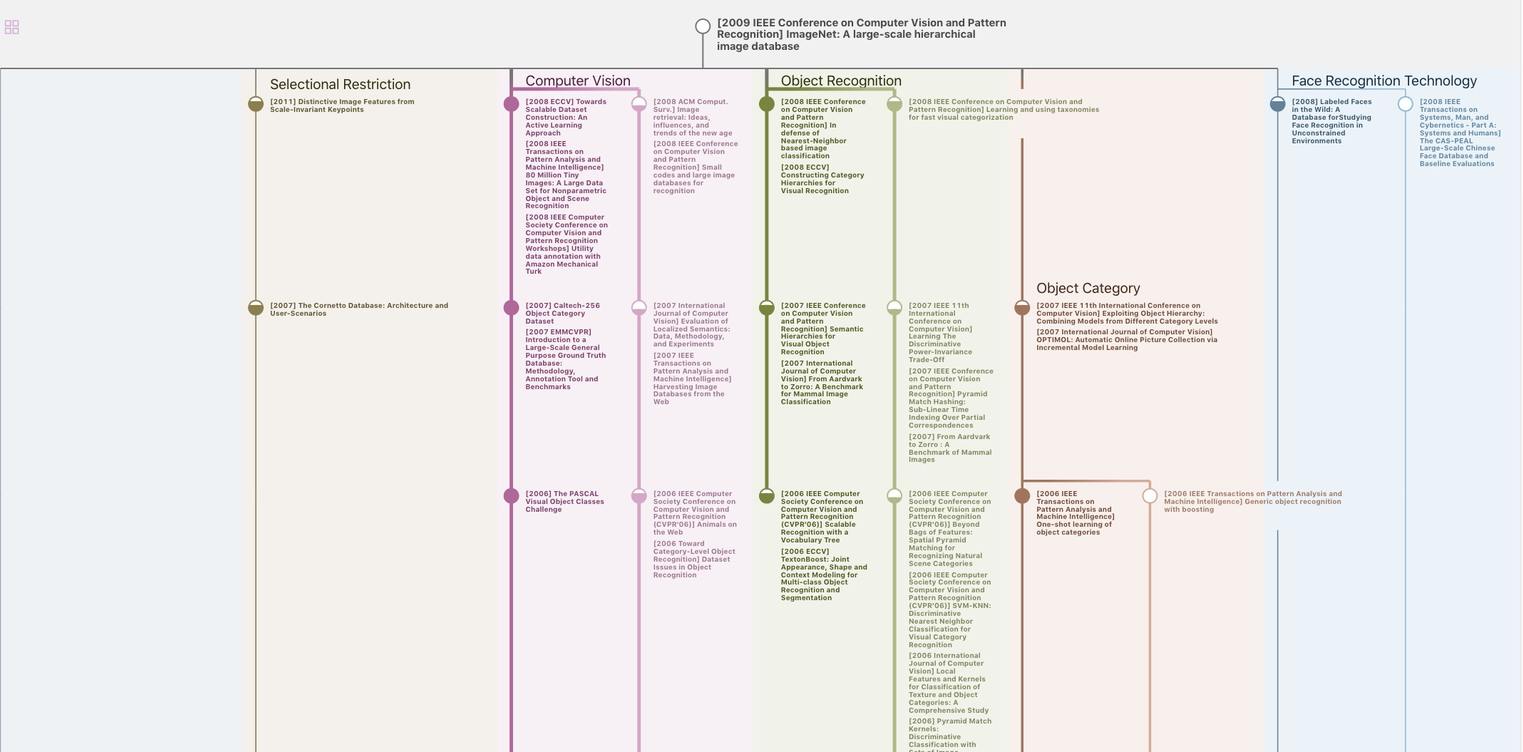
生成溯源树,研究论文发展脉络
Chat Paper
正在生成论文摘要