A spatial-temporal model for network-wide flight delay prediction based on federated learning
APPLIED SOFT COMPUTING(2024)
摘要
Network -wide flight delay prediction has emerged as a severe and prominent issue within the realm of intelligent aviation systems, owing to its crucial impact on flight scheduling, airline planning, and airport operations. The methodology employed in flight delay prediction has undergone substantial evolution in recent years, progressing from rudimentary statistical models to more sophisticated and intricate deep learning models. Nevertheless, the majority of current research endeavors primarily concentrate on the utilization of all available local data to collectively construct a prediction model, enduring significant challenges pertaining to data security and privacy issues as well as enormous communication overhead. To tackle these challenges, this investigation centers its attention on developing a hybrid federated deep learning model (HFDL), which only emphasizes updating the model parameters exclusively on the central server without the need for accessing or sharing any private data. Firstly, we employ the Louvain algorithm to partition the global spatial graph of the airport network into several sub -regions. Then, we present a local training model for each sub -region, which uses a diffusion graph convolutional network (DGCN) and residual gated recurrent unit (RGRU) to model the complex local temporal and spatial dependencies of the airport network. The model utilizes a branch structure to predict the flight delay at each airport within a sub -region. Finally, the local models are aggregated through federated learning to form a vigoroso central model that bridges the limits on global data sharing and privacy guarantee. Experiments are carried out on a real flight delay dataset from the U.S. Bureau of Transportation Statistics (BTS) database with the aim of analyzing the performance of the proposed prediction model. The experimental results demonstrate that the HFDL model can attain excellent prediction performance without the interaction of sub -region raw data and improve training efficiency. Moreover, the model has strong generalization ability. The visualization results demonstrate that the proposed model can effectively explore the spatial-temporal dependencies of flight delays, providing guidance for formulating air traffic control strategies.
更多查看译文
关键词
Network-wide flight delay prediction,Spatial-temporal network,Federated learning,Deep learning,Graph convolutional network
AI 理解论文
溯源树
样例
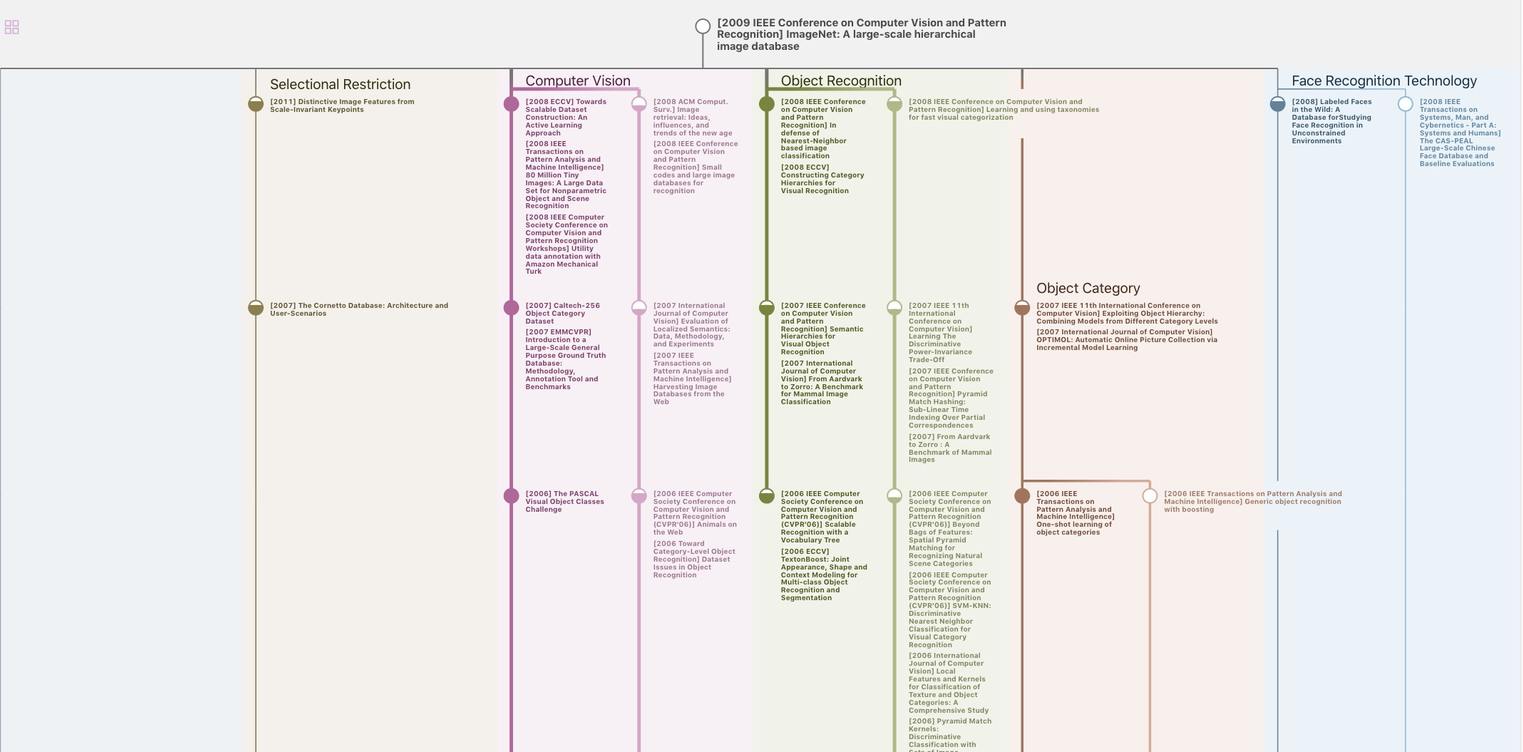
生成溯源树,研究论文发展脉络
Chat Paper
正在生成论文摘要