Application of supervised random forest paradigms based on optimization and post-hoc explanation in underground stope stability prediction
APPLIED SOFT COMPUTING(2024)
摘要
This study applies a supervised random forest (SRF) paradigm to predict the underground stope stability. To improve the model prediction accuracy, 400 actual stopes with three main features (the optimized stability number (N), the hydraulic radius (HR), and a rock mass quality factor (Q)) are used to train the model, and nine meta -heuristic (MH) optimization algorithms are utilized to select the optimal hyperparameters of the SRF model. The prediction results show that the tiki taka algorithm -based SRF (TTA-SRF) model obtains the most satisfactory classification performance, with the smallest variance in the overfitting evaluation (Precision of 0.0201, Recall of 0.0257, Specificity of 0.0061, Accuracy of 0.0125, and F1 -measure of 0.0246). Furthermore, four post -hoc explainable techniques (i.e., model -independent) including the feature importance (FI), the Shapley additive explanations (SHAP), the partial dependence plot (PDP), and the individual conditional expectation (ICE) are adopted to explain the best prediction model. The results of the model interpretation show that all features are essential for predicting the stope stability and they have an opposite tendency on the stability prediction of stable and caved stopes. In particular, the limits at which HR, Q, and N do not contribute to the stope stability prediction are 18 m, 40, and 100, respectively. The results of optimization designs for a specific case stope based on the visualization program showed that the suggestions given by the prediction model are desirable. In conclusion, a high-performance and strongly explanatory prediction model is proposed in this study to facilitate the refinement of stope stability assessment in underground space.
更多查看译文
关键词
Underground stope,Supervised random forest,Post -hoc explanation,Meta -heuristic optimization
AI 理解论文
溯源树
样例
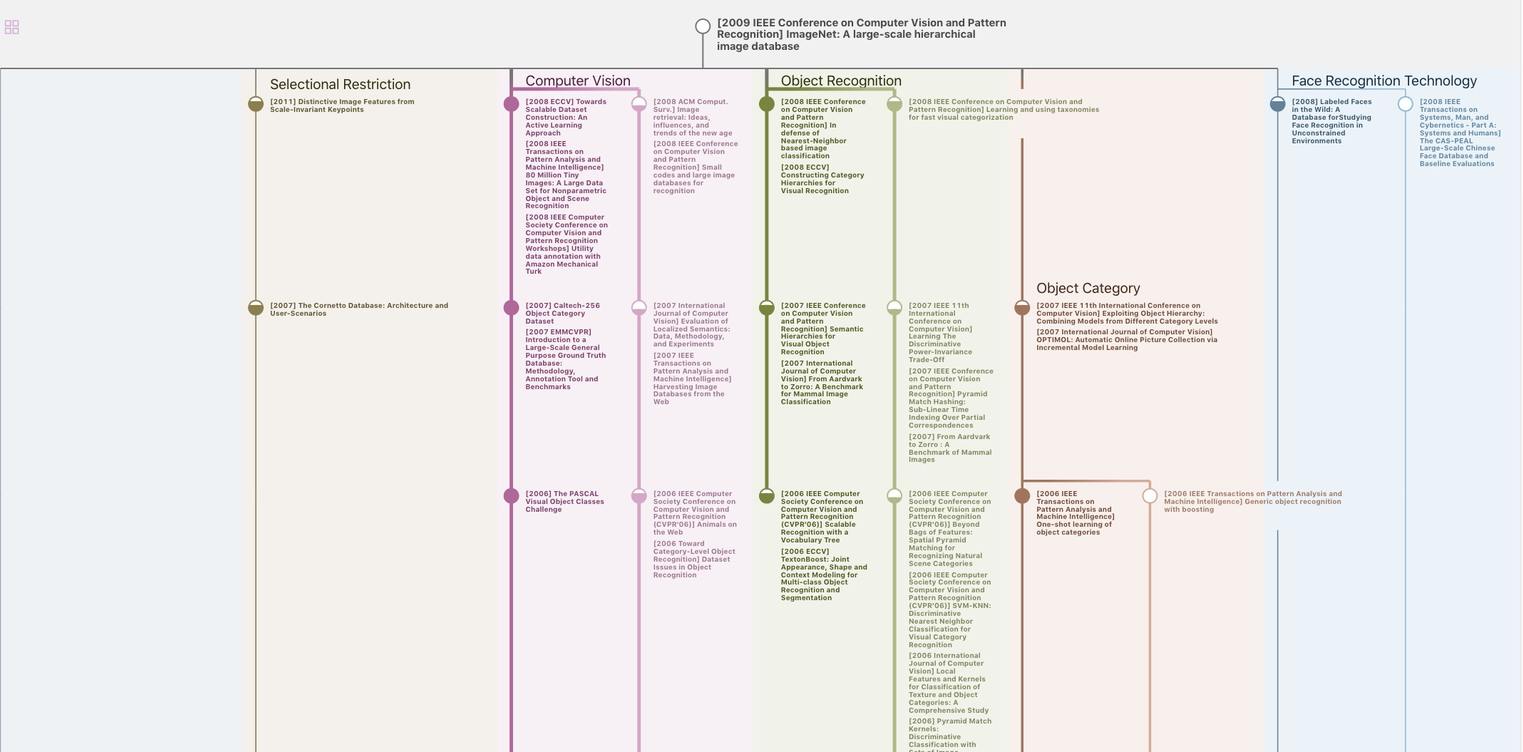
生成溯源树,研究论文发展脉络
Chat Paper
正在生成论文摘要