A label information fused medical image report generation framework
ARTIFICIAL INTELLIGENCE IN MEDICINE(2024)
摘要
Medical imaging is an important tool for clinical diagnosis. Nevertheless, it is very time-consuming and errorprone for physicians to prepare imaging diagnosis reports. Therefore, it is necessary to develop some methods to generate medical imaging reports automatically. Currently, the task of medical imaging report generation is challenging in at least two aspects: (1) medical images are very similar to each other. The differences between normal and abnormal images and between different abnormal images are usually trivial; (2) unrelated or incorrect keywords describing abnormal findings in the generated reports lead to mis-communications. In this paper, we propose a medical image report generation framework composed of four modules, including a Transformer encoder, a MIX-MLP multi -label classification network, a co -attention mechanism (CAM) based semantic and visual feature fusion, and a hierarchical LSTM decoder. The Transformer encoder can be used to learn long-range dependencies between images and labels, effectively extract visual and semantic features of images, and establish long-term dependent relationships between visual and semantic information to accurately extract abnormal features from images. The MIX-MLP multi -label classification network, the co -attention mechanism and the hierarchical LSTM network can better identify abnormalities, achieving visual and text alignment fusion and multi -label diagnostic classification to better facilitate report generation. The results of the experiments performed on two widely used radiology report datasets, IU X-RAY and MIMIC-CXR, show that our proposed framework outperforms current report generation models in terms of both natural linguistic generation metrics and clinical efficacy assessment metrics. The code of this work is available online at https://github.com/watersunhznu/LIFMRG.
更多查看译文
关键词
Medical image,Text generation,Attention mechanism,Multi-modal feature fusion,Feature extraction
AI 理解论文
溯源树
样例
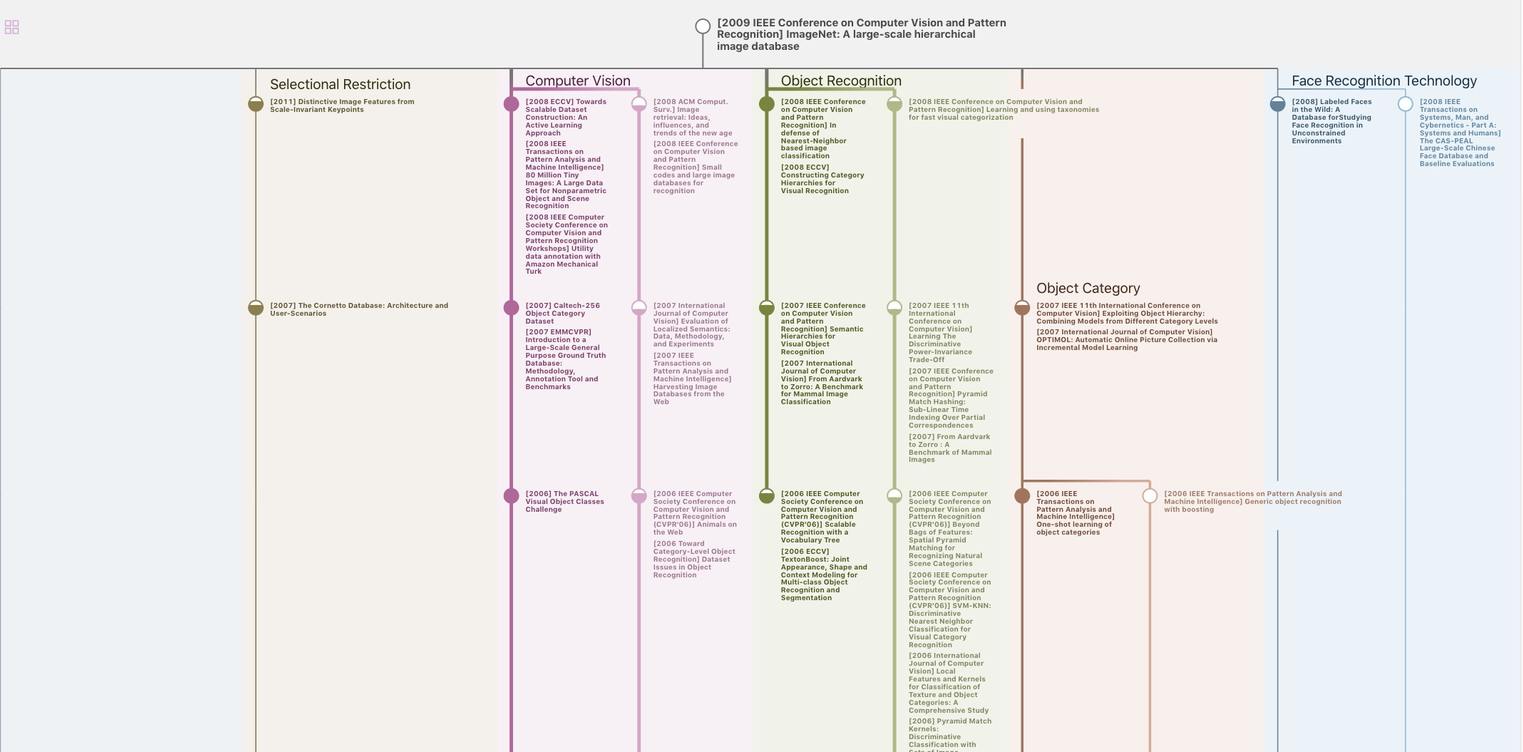
生成溯源树,研究论文发展脉络
Chat Paper
正在生成论文摘要