Precipitable water vapor estimation from Himawari-8/AHI observations using a stacking machine learning model
Atmospheric Research(2024)
摘要
The Advanced Himawari Imager (AHI) onboard the new-generation geostationary satellite Himawari-8 is designed to capture visible light and infrared images of the Asia-Pacific region in 16 bands, providing a potential mean for monitoring precipitable water vapor (PWV). However, no algorithm is specially developed for the Himawari-8/AHI to estimate PWV from its multichannel observations, resulting in underutilization of its capability. Therefore, this study proposes a stacking machine learning (SML) model to retrieve PWV over lands under clear-sky from Himawari-8/AHI observations. The SML model consists of two sub-models: a random forest (RF) model and a backpropagation neural network (BPNN) model. The PWVs are first estimated from each sub-model and then fused using a multiple linear regression (MLR) model. Moreover, the linear combination of the split-window channels is incorporated as an additional input to the SML model. The validation results show that the Himawari-8/AHI PWVs derived from the SML model are in good agreement with those derived from global navigation satellite system (GNSS) and radiosonde, and their accuracy outperforms the PWV products from Fengyun 4 A (FY-4 A). Compared to the radiosonde PWV, the root mean square errors (RMSE) and bias are 1.97 mm and −0.92 mm for the Himawari-8 PWV and 2.92 mm and 0.87 mm for the FY-4 A PWV. Furthermore, the SML model exhibited excellent performance in estimating PWV during the unmodelled period, with an RMSE/Bias of 1.73/−0.53 mm compared to GNSS.
更多查看译文
关键词
Precipitable water vapor,Himawari-8,Stacking model,Machine learning
AI 理解论文
溯源树
样例
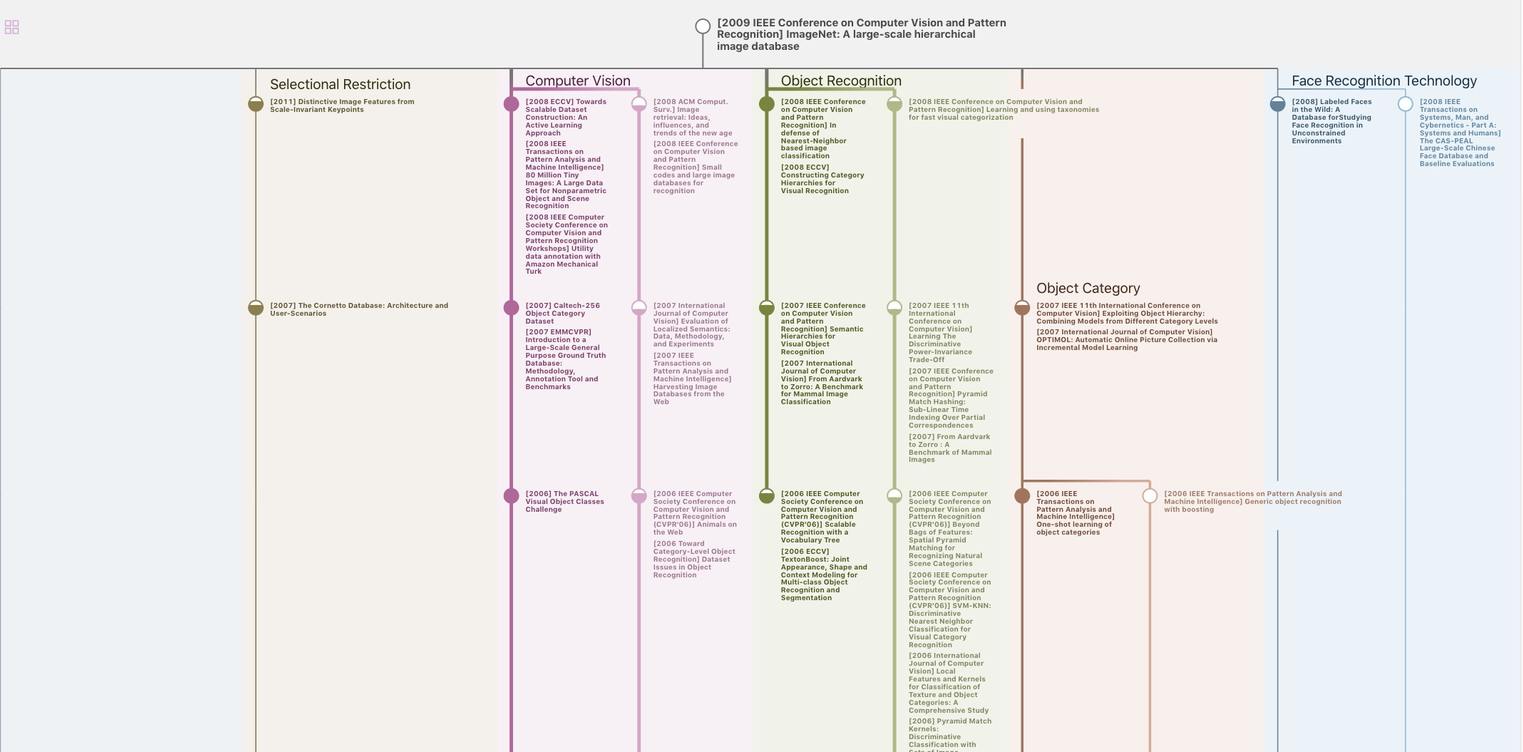
生成溯源树,研究论文发展脉络
Chat Paper
正在生成论文摘要