TRespNET: A dual-route exploratory CNN model for pediatric adventitious respiratory sound identification
BIOMEDICAL SIGNAL PROCESSING AND CONTROL(2024)
摘要
Pediatric respiratory diseases significantly contribute to the global burden of morbidity and mortality among children. Moreover, the long-term persistence of respiratory diseases from childhood into adulthood underscores the critical importance of early identification and diagnosis of such diseases. Auscultation, the widely recognized diagnostic method for respiratory diseases, relies on expertise and is subject to variability across different practitioners. To address these challenges, extensive research has focused on automating respiratory disease detection; however, the majority of existing studies have focused on adult populations. Therefore, in this study, we utilize the SPRSound database, which comprises respiratory events from 288 youths aged between 1 month and 18 years to develop deep learning-based models for automatic adventitious lung sound detection in children. Initially, we explore various architectures, including convolutional neural networks with and without transformer encoders, as well as vision transformer. Building upon these investigations, we propose a novel spectrotemporal deep neural network called TRespNET, which incorporates both Mel-scale spectrograms and raw time series of sound recordings. In addition, we integrate hand-crafted acoustic features with the proposed neural network feature maps for further model development. Our results yield a specificity of 0.98, a sensitivity of 0.84, and a harmonic score of 0.90, demonstrating the superiority of the proposed method over all existing methods on the SPRSound dataset. This research provides remarkable insights into an automated and accurate approach for respiratory disease classification in children.
更多查看译文
关键词
Adventitious lung sounds,Convolutional neural network,Vision transformer,TRespNET,Neural fusion,Acoustical analysis
AI 理解论文
溯源树
样例
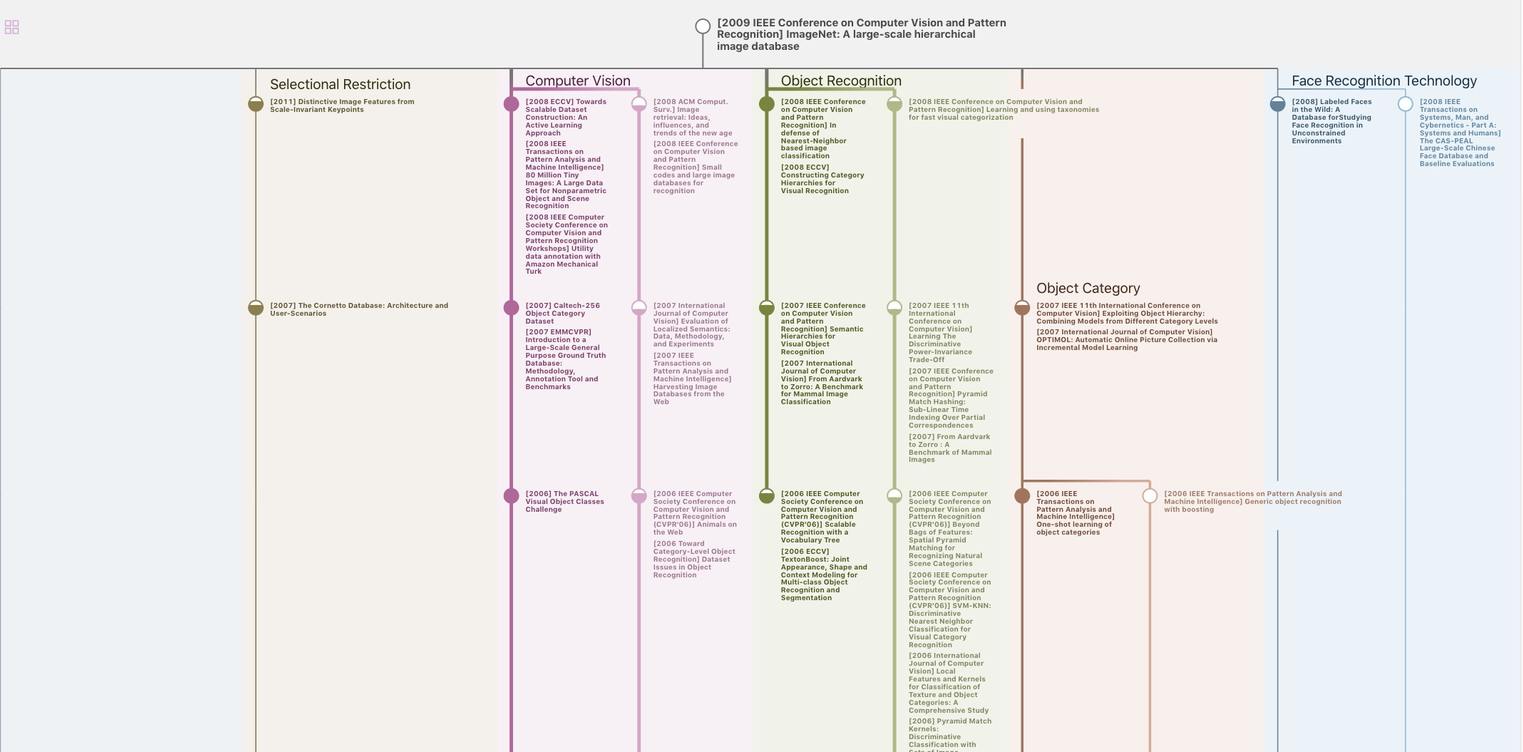
生成溯源树,研究论文发展脉络
Chat Paper
正在生成论文摘要