Data-driven estimation for uphill continuous rehabilitation motion at different slopes using sEMG
BIOMEDICAL SIGNAL PROCESSING AND CONTROL(2024)
摘要
Uphill movement is considered an important rehabilitation training activity, with the estimation of its motion intentions based on human surface electromyography (sEMG) signals proving advantageous for enhancing the effectiveness of rehabilitation training. In this study, we propose a novel uphill continuous rehabilitation motion estimation (UCRME) method called URENet to build a mapping model from sEMG of human to multi-joint intentions. First, we introduce a data-driven model and enhance its performance with a new feature extraction set. We evaluated the effectiveness of URENet through walking experiment with six subjects at random slopes. Second, our results indicate that URENet outperforms other methods of sequence prediction in terms of UCRME (p<0.001). The performance of the proposed method is compared with the performance of LSTM, ELM and GRU. Different feature sets were also compared. The results show that the proposed URENet method has better performance than the comparison scheme for UCRME, which achieved the minimum normalized root mean squared error (NRMSE) +/- standard error of the mean (SEM) (degrees) of 0.077 +/- 0.011, 0.076 +/- 0.012, 0.127 +/- 0.016 for the hip joint motion estimation and 0.065 +/- 0.01, 0.081 +/- 0.01, 0.128 +/- 0.008 for the knee joint motion estimation at slopes 7 degrees,12 degrees,17 degrees. Compared with the comparative schemes, the average reduction rate of multi-joint estimation for the URENet method in the UCRME reached 28.31%. In summary, this study underscores the potential for heightened intention recognition in UCRME through the application of accurate and stable continuous motion estimation.
更多查看译文
关键词
sEMG,Data-driven estimation,Feature extraction,Rehabilitation training
AI 理解论文
溯源树
样例
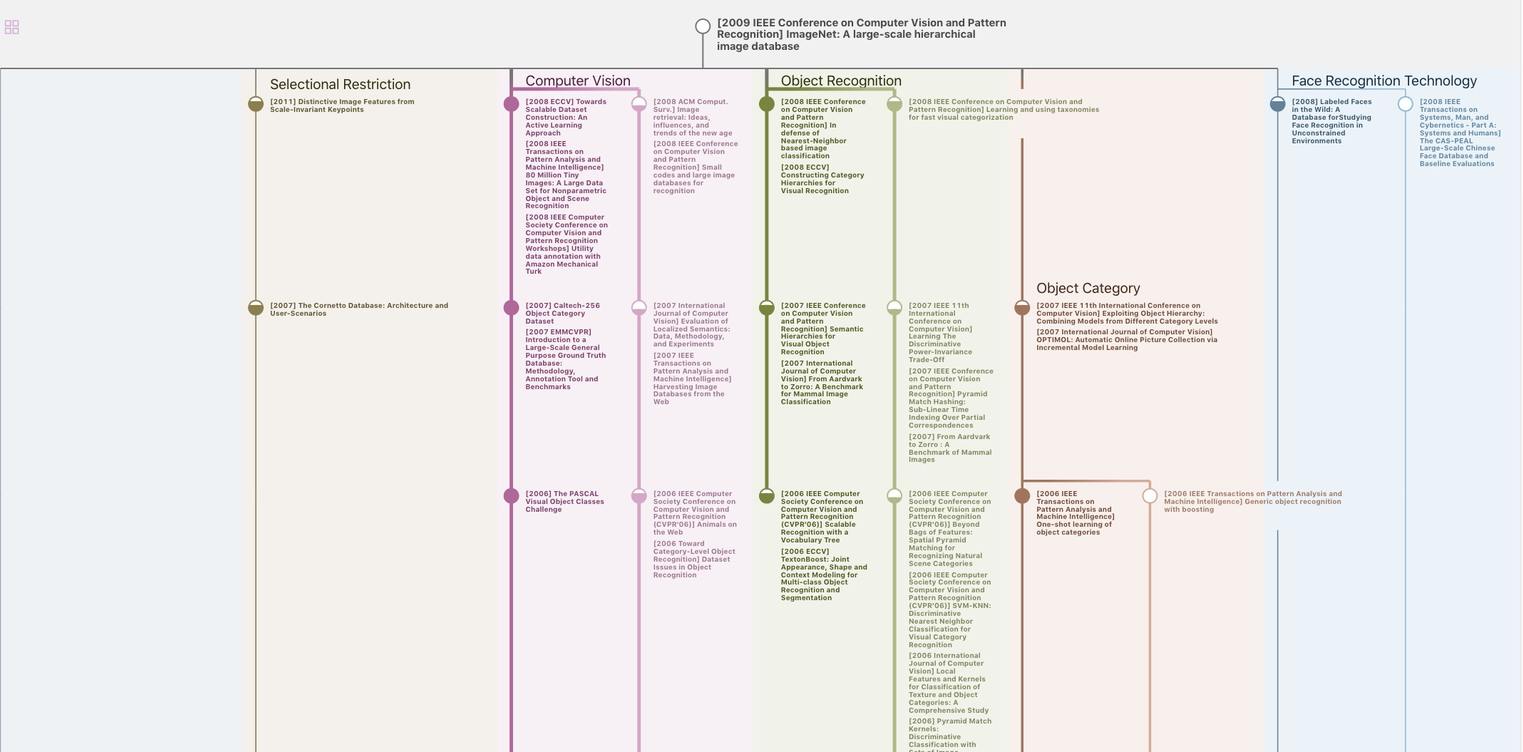
生成溯源树,研究论文发展脉络
Chat Paper
正在生成论文摘要