Dissecting the latent representation of age inside a deep neural network’s predictions of diastolic dysfunction using echocardiographic variables
Biomedical Signal Processing and Control(2024)
摘要
Objective
This study explored the hidden layer activations of previously published Deep Neural Networks (DNNs) that detect age-related diastolic function changes through echocardiographic parameters. The study also aimed to decode the prediction mechanism to understand if biological age and sex were latent factors in the DNN model.
Methods
We performed a secondary analysis of a previously published DNN model trained using data from 1009 patients (62 ± 17 years, 57 % females) to predict risk phenogroups from nine echocardiographic parameters. It was tested on an internal (n = 243, mean age = 62 ± 17 years, ∼57 % females) and an external (n = 5596, mean age = 76 ± 5 years, ∼57 % females) validation cohort. To predict biological age and sex, linear regression and classification models were developed using hidden layer activations from the DNN. Model performance was evaluated using Pearson’s correlation for regression; accuracy and area under the curve (AUC) metrics for classification.
Results
On assessing the hidden layer activations, we observed that the model captured biological age accurately in both younger and older populations, especially in the low-risk phenogroup with strong correlations for the total population (0.94, p < 0.001), males (0.90, p < 0.001), and females (0.94, p < 0.001). In the high-risk phenogroup, the correlations were lower (0.31, p = 0.274) for the entire population, (0.76, p = 0.003) for males, and (0.11, p = 0.723) for females. Sex was predicted as a latent variable in the internal (accuracy 58.02 %) and external (accuracy 52.2 %) validation cohorts, with an AUC of 0.65 for both groups.
Conclusion
The study indicates that DNN's latent space retains an age-dependent link with diastolic functional parameters, providing an age-independent solution for predicting diastolic dysfunction. Network dissection can yield a further understanding of the learned information in DNNs, providing novel pathophysiological insights for clinicians.
更多查看译文
关键词
Deep neural network,Echocardiography,Left ventricular diastolic dysfunction,Age,Sex prediction
AI 理解论文
溯源树
样例
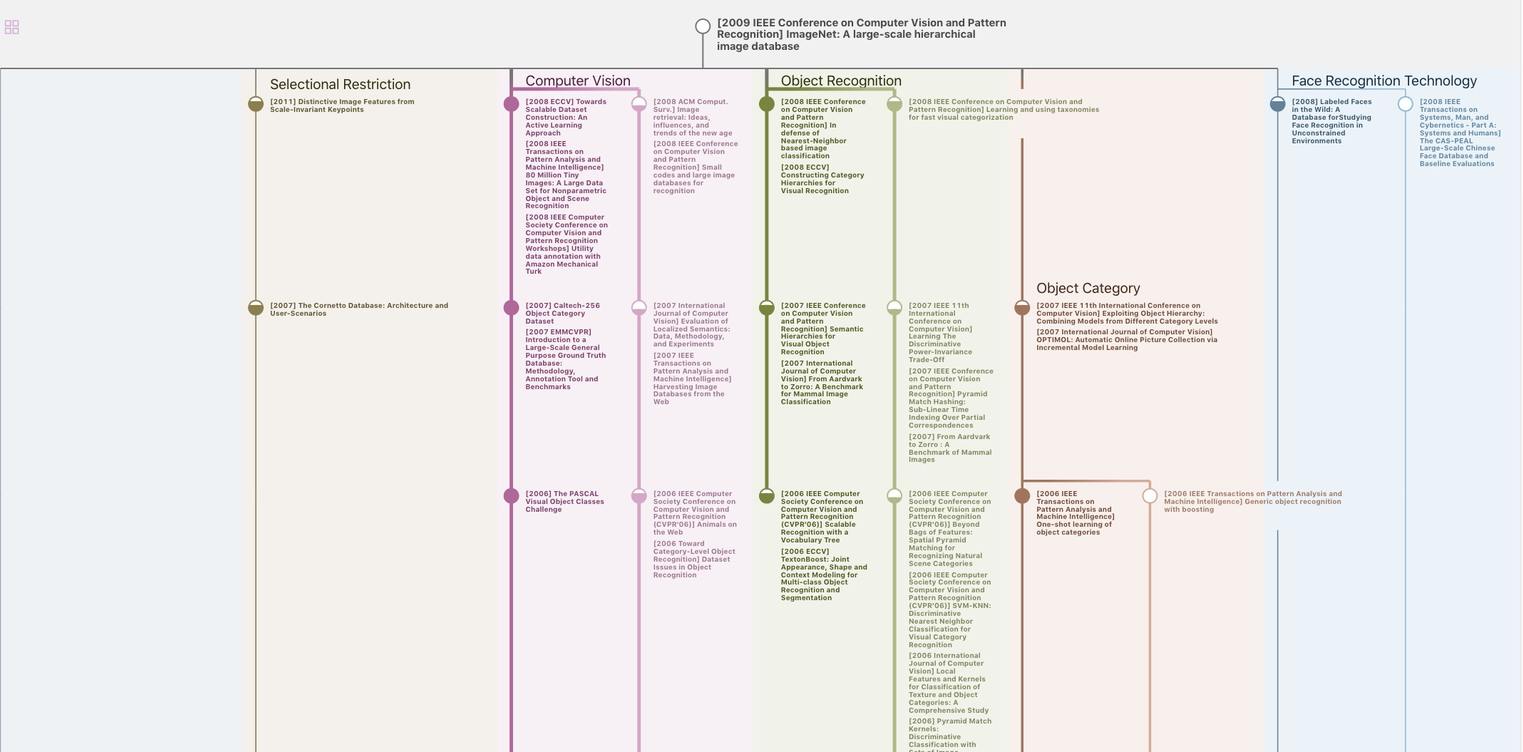
生成溯源树,研究论文发展脉络
Chat Paper
正在生成论文摘要