Optimization of inter-subject sEMG-based hand gesture recognition tasks using unsupervised domain adaptation techniques
BIOMEDICAL SIGNAL PROCESSING AND CONTROL(2024)
摘要
Neuromuscular diseases or physical disabilities have the potential to impair hand dexterity, significantly affecting daily life. To date, technologies for hand gesture recognition based on surface electromyography (sEMG) have garnered increasing attention. These technologies aim to decode motion intentions, thereby advancing assistive devices such as prosthetic hands in restoring lost hand function. However, the limited generalization capacity across different users has hindered progress towards practical implementation. In this study, high-density (256-channel) sEMG data of 10 commonly used hand gestures were collected from 41 subjects on their two days. Then, we evaluated the inter-subject classification performances. To guarantee strong robustness over users, we systematically investigated eight prevailing unsupervised domain adaptation techniques to align the feature distribution between the source domain and the target domain, and combined these techniques with 5 classifiers. Afterwards, a simplified approach is proposed. Meanwhile, to make a comprehensive comparison, extensive validation on both private dataset and two publicly available datasets (Ninapro DB4 and Ninapro DB5) are evaluated. As a result, our proposed approach achieving remarkable classification accuracies of 81.74%, 84.00%, and 93.50%, respectively. The outcomes showed that the proposed approach is promising to build for addressing the inter-subject differences and make significant strides in the field of gesture recognition for inter-subject scenario.
更多查看译文
关键词
Hand gesture recognition,surface electromyogram (sEMG),Transfer learning,Unsupervised domain adaptation
AI 理解论文
溯源树
样例
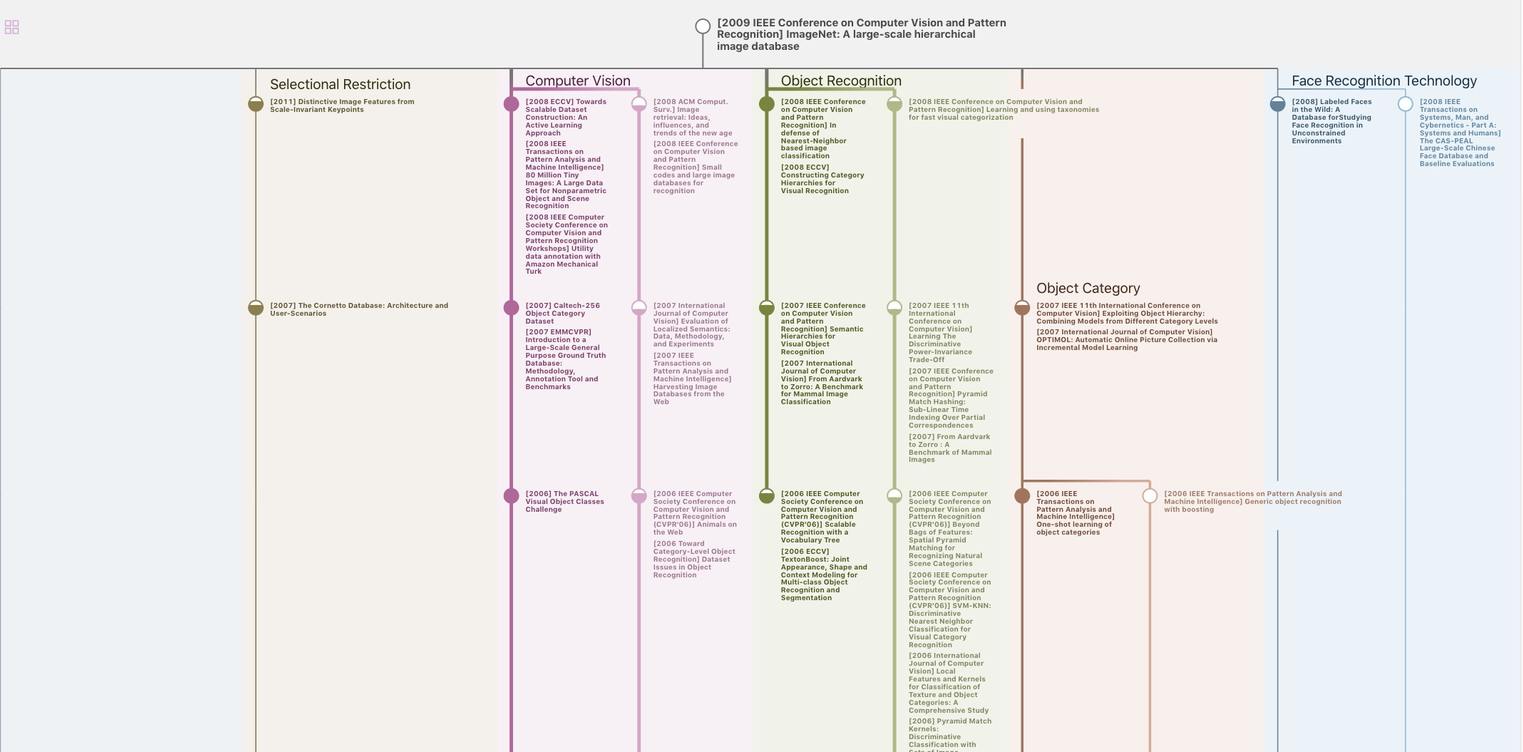
生成溯源树,研究论文发展脉络
Chat Paper
正在生成论文摘要