An outlier detection based two-stage EEG artifact removal method using empirical wavelet transform and canonical correlation analysis
BIOMEDICAL SIGNAL PROCESSING AND CONTROL(2024)
摘要
Electroencephalography (EEG) is commonly used for measuring brain activity information due to its high temporal resolution. However, it severely suffers from noises produced by non-brain sources, called EEG artifacts. Ocular, muscle, and power line artifacts are the most common ones. In this paper, a novel twostage automatic artifact removal method is proposed to handle different artifacts under miscellaneous EEG applications. Empirical wavelet transform (EWT), canonical correlation analysis (CCA), and an outlier detection algorithm, isolation forest constitute the pipeline. In the first stage, EEG data are decomposed by CCA and preliminary purified by isolation forest. In the second stage, a further decomposition of the EEG data is conducted using EWT and CCA, followed by removal of irrelevant components using isolation forest. We thoroughly evaluate the qualitative and quantitative performance of the proposed method on multiple datasets. Experiment results show that the proposed method can effectively remove artifacts under complex conditions and different signal-to-noise ratios. Ablation studies and comparison results demonstrate the significance of the two-stage combination, which outperforms single -stage methods and state-of-the-art methods. This paper explores the potential for a fully data-driven and adaptive way for robust artifact removal in various EEG applications.
更多查看译文
关键词
Electroencephalography (EEG),Artifact removal,Empirical wavelet transform (EWT),Canonical correlation analysis (CCA),Isolation forest
AI 理解论文
溯源树
样例
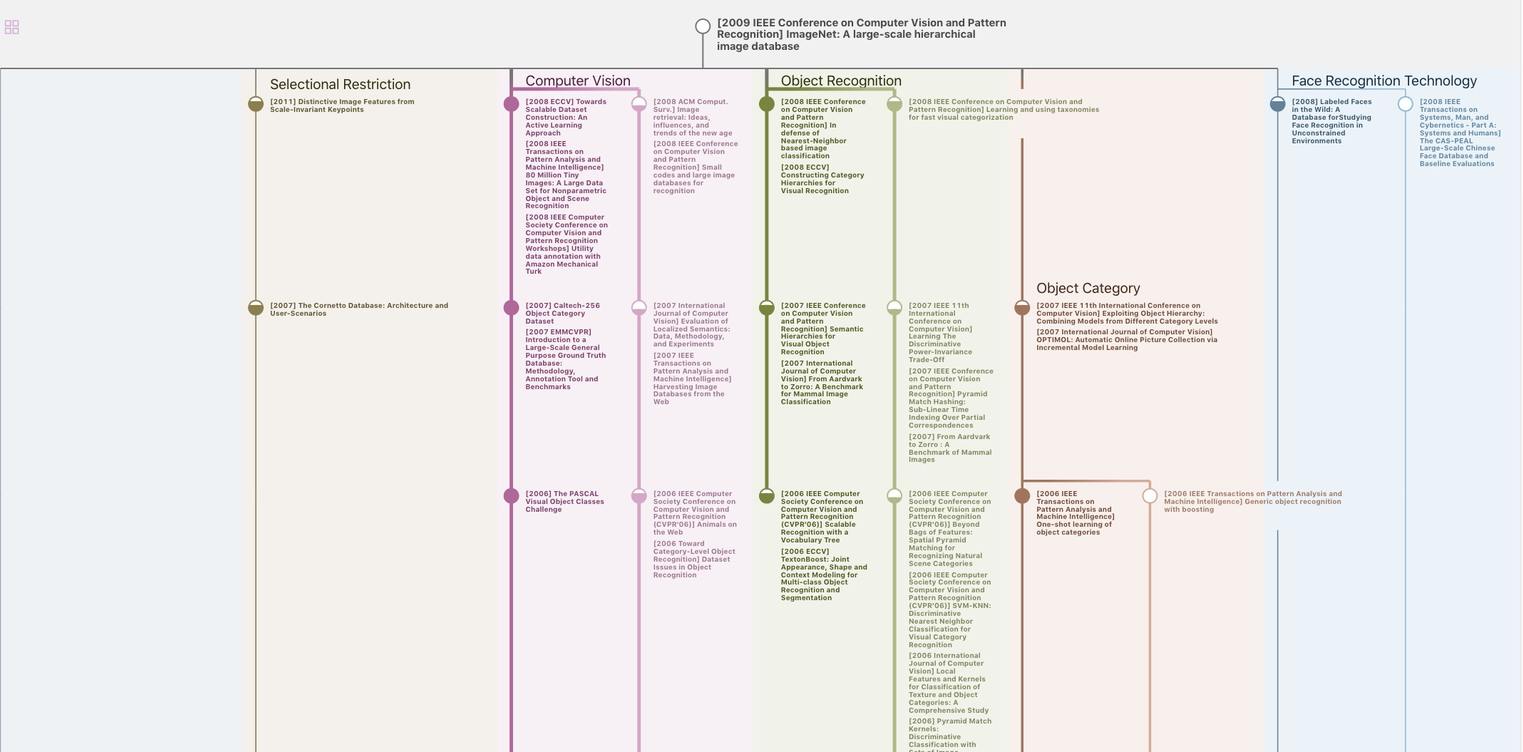
生成溯源树,研究论文发展脉络
Chat Paper
正在生成论文摘要