Multi-scale local-global transformer with contrastive learning for biomarkers segmentation in retinal OCT images
BIOCYBERNETICS AND BIOMEDICAL ENGINEERING(2024)
摘要
Quantitative analysis of biomarkers in Optical Coherence Tomography (OCT) images plays an import role in the diagnosis and treatment of retinal diseases. However, biomarker segmentation in retinal OCT images is very hard due to the large variations in size and shape of retinal biomarkers, blurred boundaries, low contrast, and speckle interference. We proposed a novel Multi -scale Local -Global Transformer network (MsLGT-Net) for biomarker segmentation in retinal OCT images. The network combines the proposed Multi -scale Fusion Attention (MFA) module, Local -Global Transformer (LGT) module, and Contrastive Learning Enhancement (CLE) module to tackle the challenges of biomarker segmentation. Specifically, the proposed MFA module aims to enhance the network's ability to learn multi -scale features of retinal biomarkers by effectively combining the local detail information and contextual semantic information of biomarkers at different scales, and improve the representation ability for different classes of biomarkers. The LGT module is designed to learn local and global information adaptively from multi -scale fused features to address the challenge of small biomarker segmentation. In addition, to distinguish features between different types of retinal biomarkers, we propose the CLE module to enhance the feature representation of different biomarkers. Our proposed method is validated on one public dataset and one local dataset. The experimental results show that the proposed method is more effective than other state-of-theart methods.
更多查看译文
关键词
Optical Coherence Tomography (OCT),Retinal biomarker segmentation,Multi -class segmentation,Transformer,Proposal contrastive learning
AI 理解论文
溯源树
样例
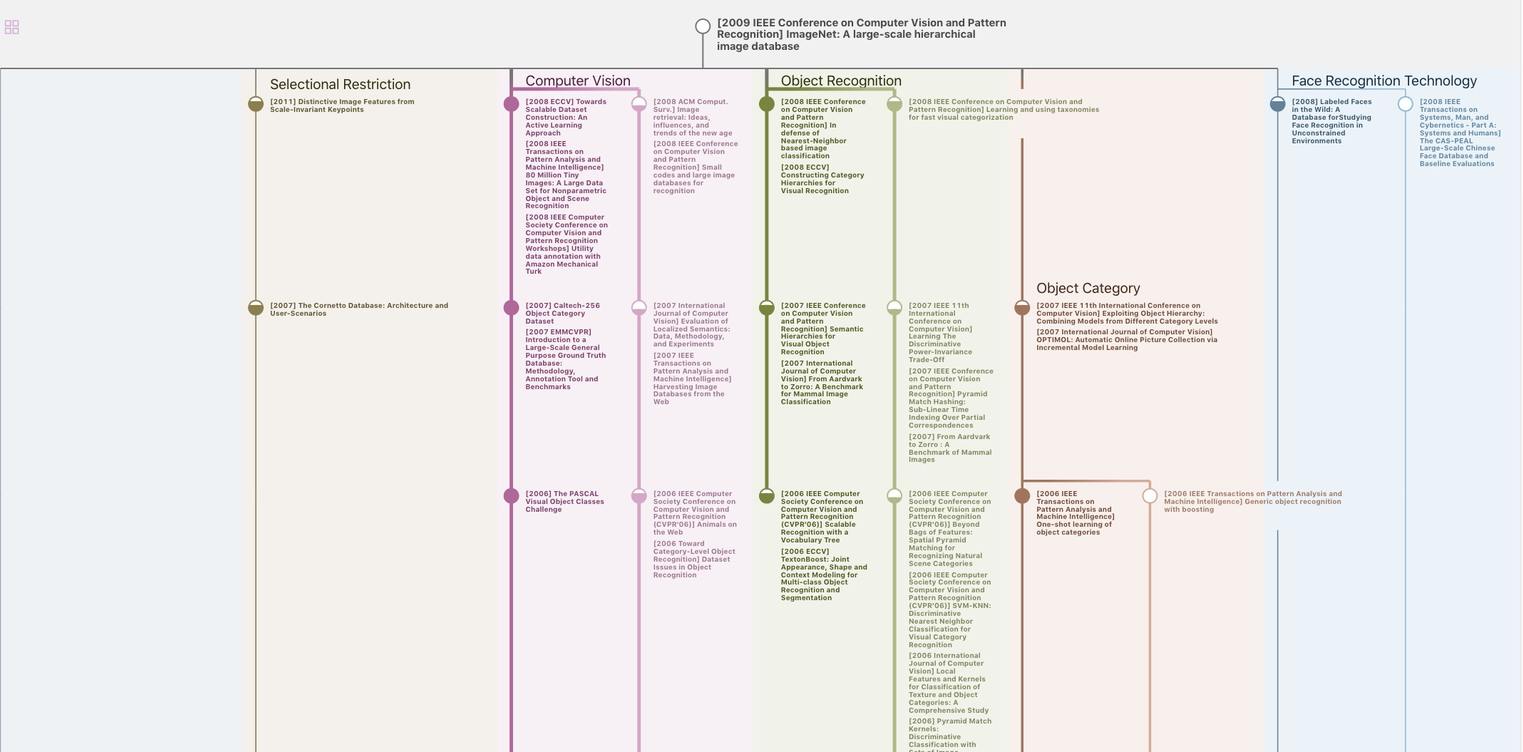
生成溯源树,研究论文发展脉络
Chat Paper
正在生成论文摘要