Tracking element-mineral associations with unsupervised learning and dimensionality reduction in chemical and optical image stacks of thin sections
CHEMICAL GEOLOGY(2024)
摘要
In -situ chemical analysis on thin sections is a cornerstone of geochemical research. Despite massive advances in digital image analysis, the interpretation of such data within the optical petrological context of the thin section has largely remained an analogue task in geochemistry. In this contribution, we registered optical and microchemical images from large thin section areas. Chemical datasets from scanning electron microscopy energy dispersive spectrometry (SEM-EDX) and Synchrotron X-ray fluorescence microscopy (S-XFM) were exported from proprietary software. We then evaluated two dimensionality reduction techniques against false -colour images and phase maps to test whether previously unnoticed features became discoverable. Principal component analysis (PCA) and deep sparse autoencoder (DSA) neural networks were used to summarise the multi -channel SEM-EDX and S-XFM into one single red -green -blue (RGB) representation each. Applied to an oceanic gabbro, a cratonic peridotite, and a pelagic limestone, the PCA-based dimensionality reduction was found to produce crisp RGB maps of phases with less noise than false -colour three -element RGB maps. They were registered with optical images to form a simple multi -channel input for pixel -based classification into classes (i.e., semantic segmentation). This was fast and worked well for typical igneous and metamorphic rocks (1-3 h routine). In the chemically quite homogenous limestone, the combined optical and S-XFM PCA input was successfully classified into many phases that were unclassifiable on the conventional SEM-EDX phase map. From the segmented optical and S-XFM PCA map, we exported pixel locations of classified accessory phases back into the original proprietary S-XFM software. This allowed quantification of trace elements whose X-ray peaks were invisible in the original S-XFM quantification. The DSA-based approach yielded phase maps with greater noise, but the technique was very strong at detecting subtle features. In the gabbro sample, the DSA image identified cryptic relict cores in clinopyroxene more clearly than in the previously used Cr concentration map. A DSA training set can be obtained on a small ROI to generate a consistent large area DSA image from which more fragmental cores were observed. For a ROI on the gabbro thin section, the DSA representation map and the blended optical image were segmented and inspected with a loupe tool. This allowed us to generate interactive histograms and bin -scatter concentration plots of selected phases and to search for co -variations of element concentrations. This approach can be expanded to include data from any in situ geochemical acquisition tools and may help discover previously unseen features in complex elemental imagery.
更多查看译文
关键词
In situ analysis,Correlative microscopy,Chemical dimensionality reduction,Semantic segmentation,Phase maps,Automated mineralogy
AI 理解论文
溯源树
样例
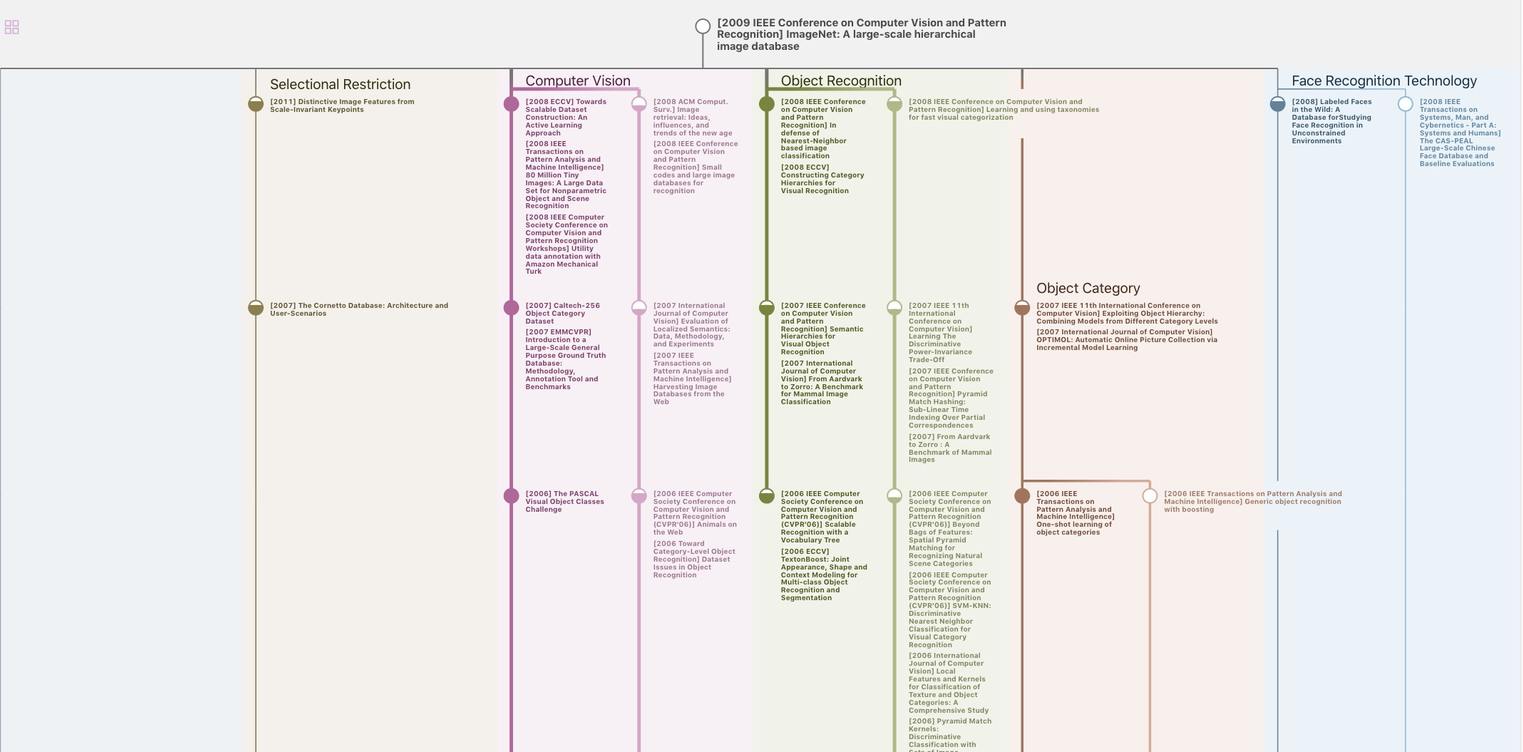
生成溯源树,研究论文发展脉络
Chat Paper
正在生成论文摘要