Machine learning-assisted design and scalable fabrication of high-performance fire-safe polycarbonate for advanced applications
CHEMICAL ENGINEERING JOURNAL(2024)
摘要
Polycarbonate exhibits great potential in various advanced applications, however its unsatisfied fire-safe performance has hindered its widespread application. Here, we use a structure-based machine learning algorithm and establish two random forest models using the molar group contribution method to engineer a robust and multifunctional polycarbonate copolymer. Our design contains both cardo structures and poly(dimethylsiloxane) chains as functional groups to achieve desired performance. As a result, the copolymer achieved UL-94 V-0 rating with a thickness of 0.8 mm, showed 57 % lower peak heat release rate, 70 % lower smoke density, as well as high glow-wire ignition temperature of 875(degrees )C and glow-wire flammability index of 975 C-degrees . It should be noted that the copolymer exhibited great resistance to butane torch fire of > 1300(degrees ) C. Experimental and calculation results confirm a condensed-phase dominant mechanism based on thermal rearrangement and crosslinking. Furthermore, enhanced mechanical strength and low dielectric constant were also observed, powering the potential applications of the obtained copolymer in various fields. The successful application of machine learning on multifunctional fire-safe polycarbonate provides a promising avenue for the design of highperformance polymeric materials.
更多查看译文
AI 理解论文
溯源树
样例
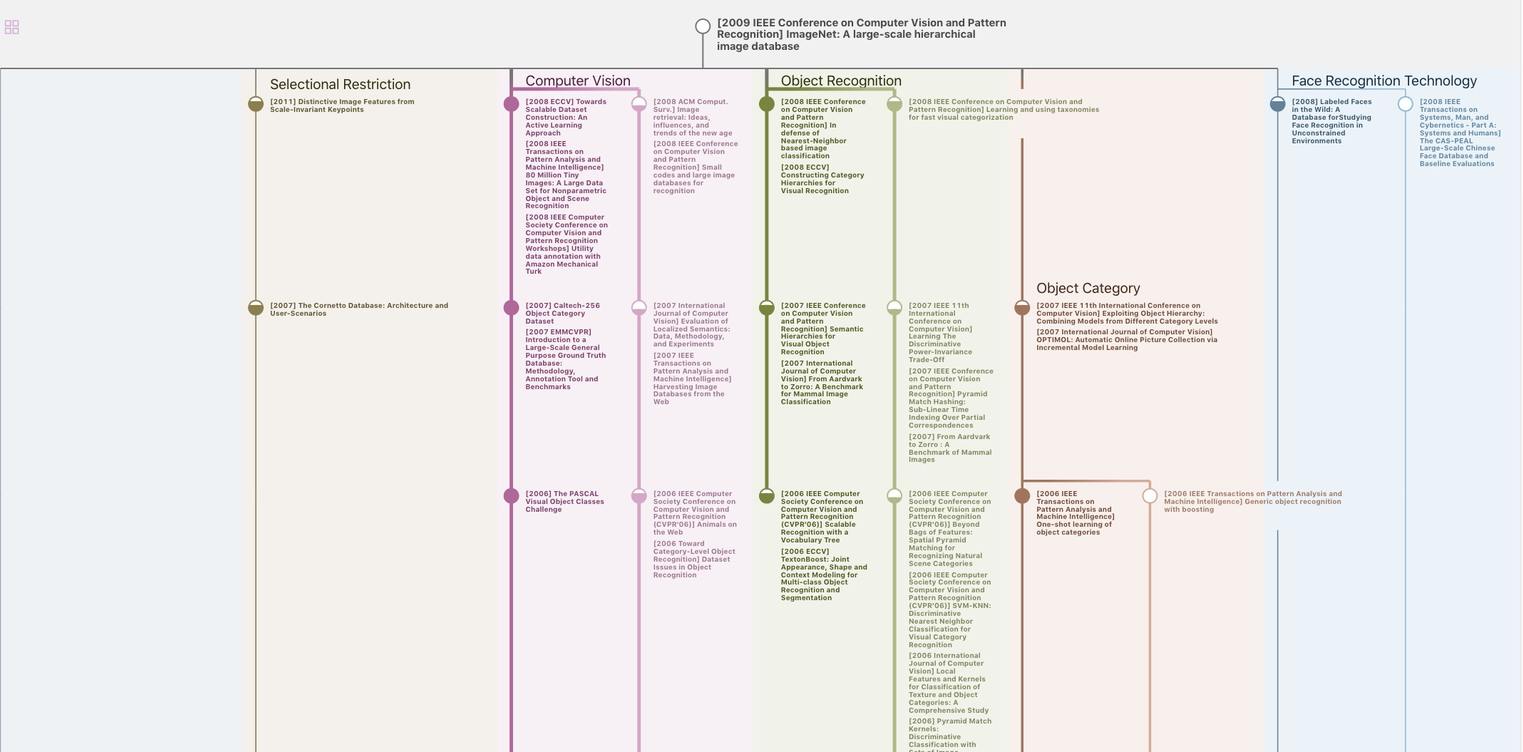
生成溯源树,研究论文发展脉络
Chat Paper
正在生成论文摘要