Anti-disturbance state estimation for PDT-switched RDNNs utilizing time-sampling and space-splitting measurements
Communications in Nonlinear Science and Numerical Simulation(2024)
摘要
Anti-disturbance state estimation for reaction–diffusion neural networks (RDNNs) subject to persistent dwell-time (PDT) switching constraints is investigated in this paper. First, PDT switching that can be utilized to characterize both the fast and slow switching processes is used in this paper to accurately model the RDNNs. Moreover, by designing the time-sampling and space-splitting measurement algorithms, the proposed state estimator can significantly reduce the measurement cost while tolerating the frequent asynchrony of the system modes and estimator ones caused by the sensor update lag. Furthermore, a state estimator is constructed to obtain the state of RDNNs affected by matched disturbances. To suppress the impact of the disturbance on the system’s state estimation, a disturbance observer and a disturbance-related controller are designed to estimate the disturbance information and ensure state estimation performance. Then, sufficient conditions for the proposed state estimator making the estimation error bounded are derived. Finally, numerical simulations for switched RDNNs with two-dimensional spatial diffusion are presented to illustrate the effectiveness and superiority of the proposed method.
更多查看译文
关键词
Anti-disturbance state estimation,Disturbance observer,Persistent dwell-time switching,Reaction–diffusion neural networks,Time-sampling and space-splitting measurements
AI 理解论文
溯源树
样例
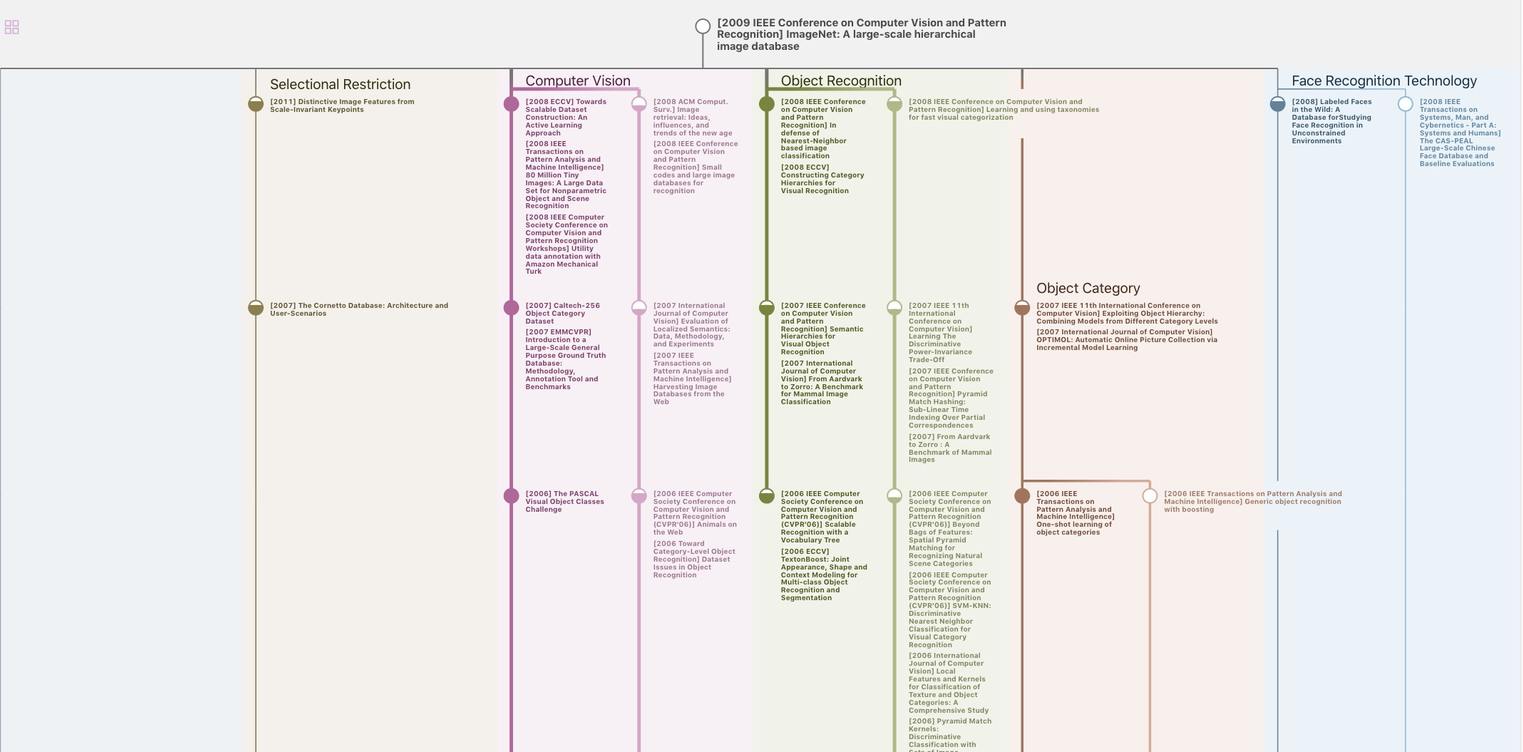
生成溯源树,研究论文发展脉络
Chat Paper
正在生成论文摘要