Translating scientific abstracts in the bio-medical domain with structure-aware models
COMPUTER SPEECH AND LANGUAGE(2024)
摘要
Machine Translation (MT) technologies have improved in many ways and generate usable outputs for a growing number of domains and language pairs. Yet, most sentence based MT systems struggle with contextual dependencies, processing small chunks of texts, typically sentences, in isolation from their textual context. This is likely to cause systematic errors or inconsistencies when processing long documents. While various attempts are made to handle extended contexts in translation, the relevance of these contextual cues, especially those related to the structural organization, and the extent to which they affect translation quality remains an under explored area. In this work, we explore ways to take these structural aspects into account, by integrating document structure as an extra conditioning context. Our experiments on biomedical abstracts, which are usually structured in a rigid way, suggest that this type of structural information can be useful for MT and document structure prediction. We also present in detail the impact of structural information on MT output and assess the degree to which structural information can be learned from the data.
更多查看译文
关键词
Neural machine translation,Document-level machine translation,Bio-medical natural language processing
AI 理解论文
溯源树
样例
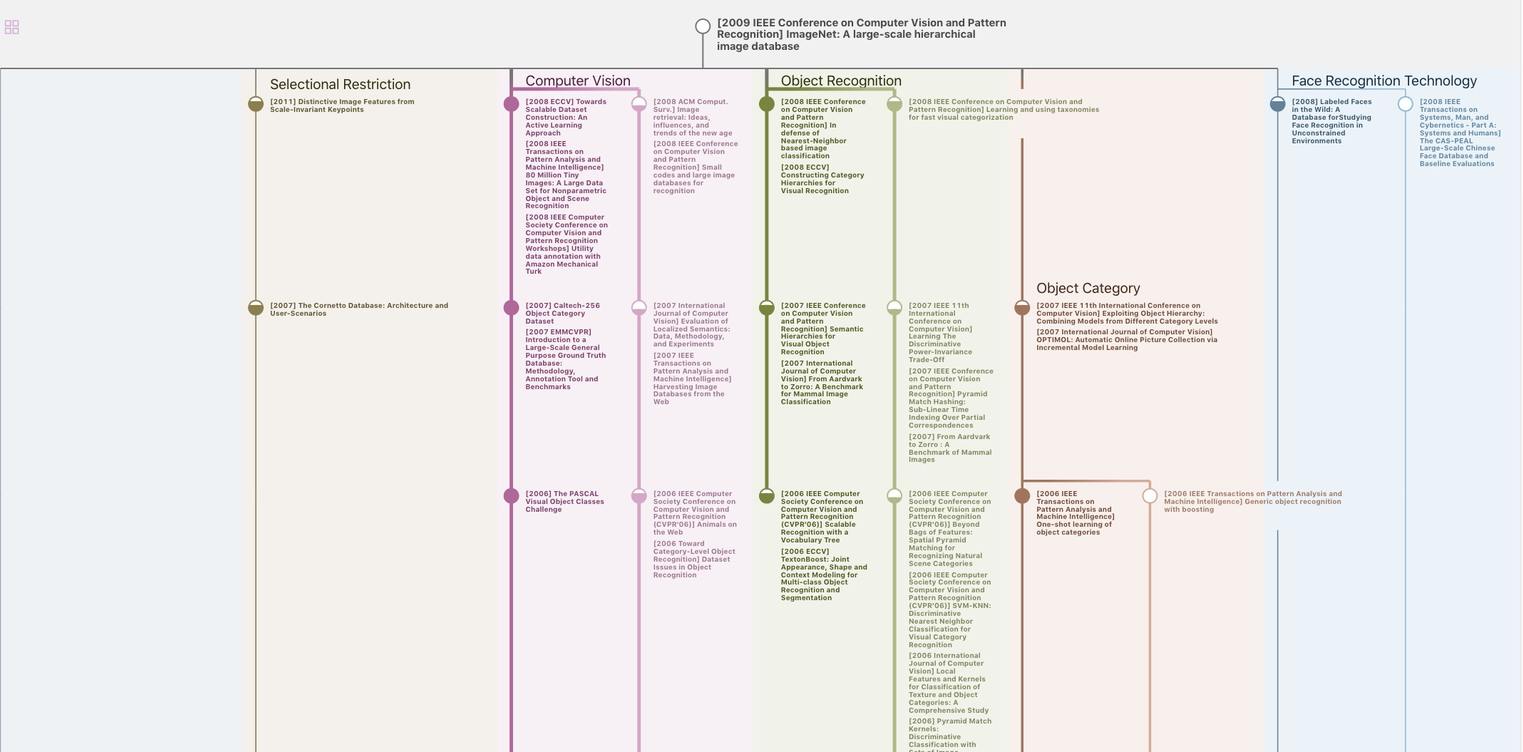
生成溯源树,研究论文发展脉络
Chat Paper
正在生成论文摘要