Lagrangian operator inference enhanced with structure-preserving machine learning for nonintrusive model reduction of mechanical systems
COMPUTER METHODS IN APPLIED MECHANICS AND ENGINEERING(2024)
Abstract
Complex mechanical systems often exhibit strongly nonlinear behavior due to the presence of nonlinearities in the energy dissipation mechanisms, material constitutive relationships, or geometric/connectivity mechanics. Numerical modeling of these systems leads to nonlinear full -order models that possess an underlying Lagrangian structure. This work proposes a Lagrangian operator inference method enhanced with structure -preserving machine learning to learn nonlinear reduced -order models (ROMs) of nonlinear mechanical systems. This two-step approach first learns the best -fit linear Lagrangian ROM via Lagrangian operator inference and then presents a structure -preserving machine learning method to learn nonlinearities in the reduced space. The proposed approach can learn a structure -preserving nonlinear ROM purely from data, unlike the existing operator inference approaches that require knowledge about the mathematical form of nonlinear terms. From a machine learning perspective, it accelerates the training of the structure -preserving neural network by providing an informed prior (i.e., the linear Lagrangian ROM structure), and it reduces the computational cost of the network training by operating on the reduced space. The method is first demonstrated on two simulated examples: a conservative nonlinear rod model and a two-dimensional nonlinear membrane with nonlinear internal damping. Finally, the method is demonstrated on an experimental dataset consisting of digital image correlation measurements taken from a lap -joint beam structure from which a predictive model is learned that captures amplitude -dependent frequency and damping characteristics accurately. The numerical results demonstrate that the proposed approach yields generalizable nonlinear ROMs that exhibit bounded energy error, capture the nonlinear characteristics reliably, and provide accurate long-time predictions outside the training data regime.
MoreTranslated text
Key words
Reduced-order modeling,Scientific machine learning,Data-driven methods,Mechanical systems,Lagrangian mechanics,Nonintrusive model reduction
AI Read Science
Must-Reading Tree
Example
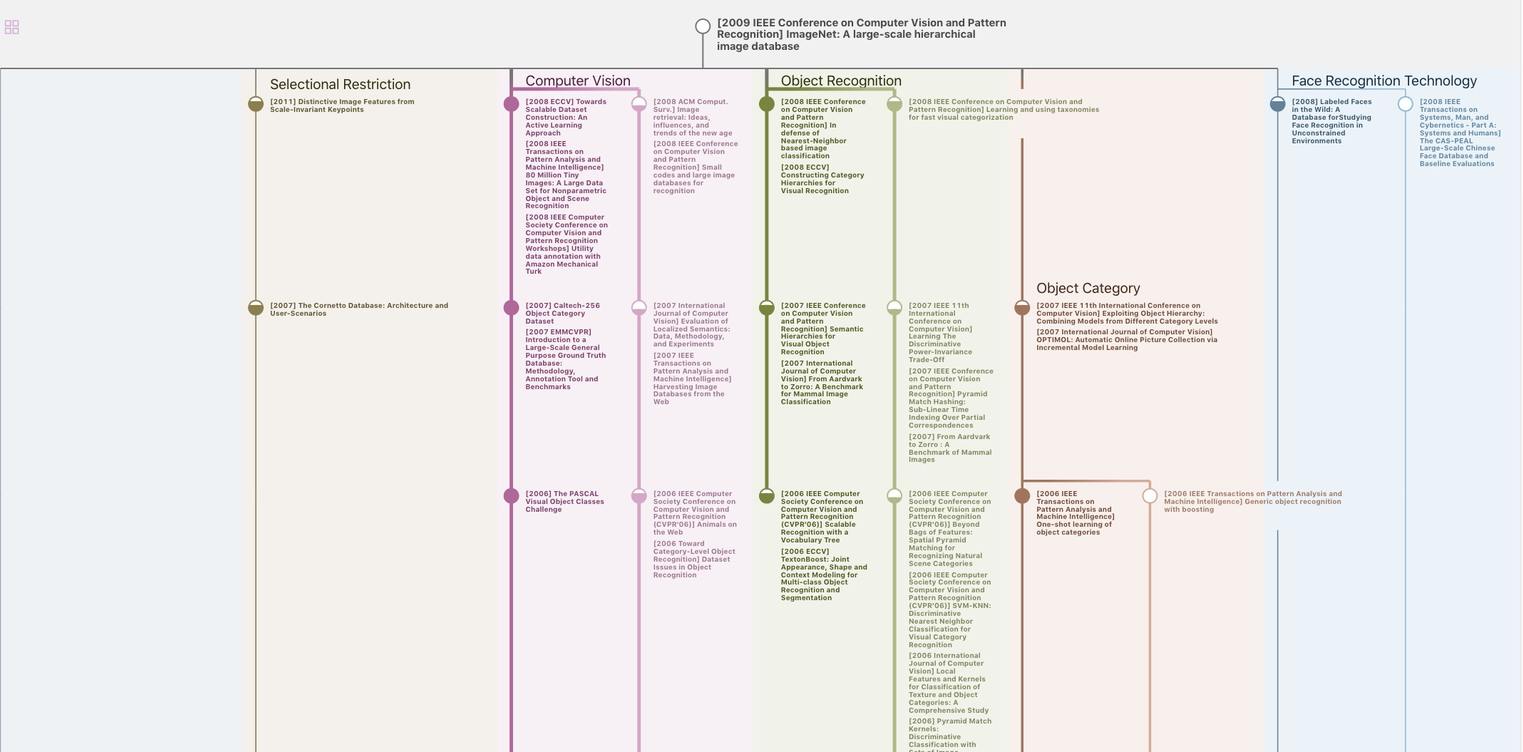
Generate MRT to find the research sequence of this paper
Chat Paper
Summary is being generated by the instructions you defined