Data-driven approaches for strength prediction of alkali-activated composites
CASE STUDIES IN CONSTRUCTION MATERIALS(2024)
摘要
Alkali-activated composites (AACs) have attracted considerable interest as a promising alternative to reduce CO2 emissions from Portland cement production and advance the decarbonisation of concrete construction. This study describes the data-driven predictive modelling to anticipate the compressive strength (CS) of AACs. Four different modelling techniques have been chosen to forecast the CS of AACs using the selected data set. The decision tree (DT), multi-layer perceptron (MLP), bagging regressor (BR), and AdaBoost regressor (AR) were employed to investigate the precision level of each model. When it comes to predicting the CS of AACs, the results show that the AR model performs better than the BR model, the MLP model, and the DT model by providing a higher value for the coefficient of determination, which is equal to 0.91, and a lower MAPE value, which is equal to 13.35%. However, the accuracy level of the BR model was very near to that of the AR model, with the R2 value suggesting a value of 0.90 and the MAPE value indicating a value of 14.43%. Moreover, the graphical user interface has also been developed for the strength prediction of alkali-activated composites, making it easy to get the required output from the selected inputs.
更多查看译文
关键词
Alkali -activated composites,Input parameters,Compressive strength,Prediction,Machine learning
AI 理解论文
溯源树
样例
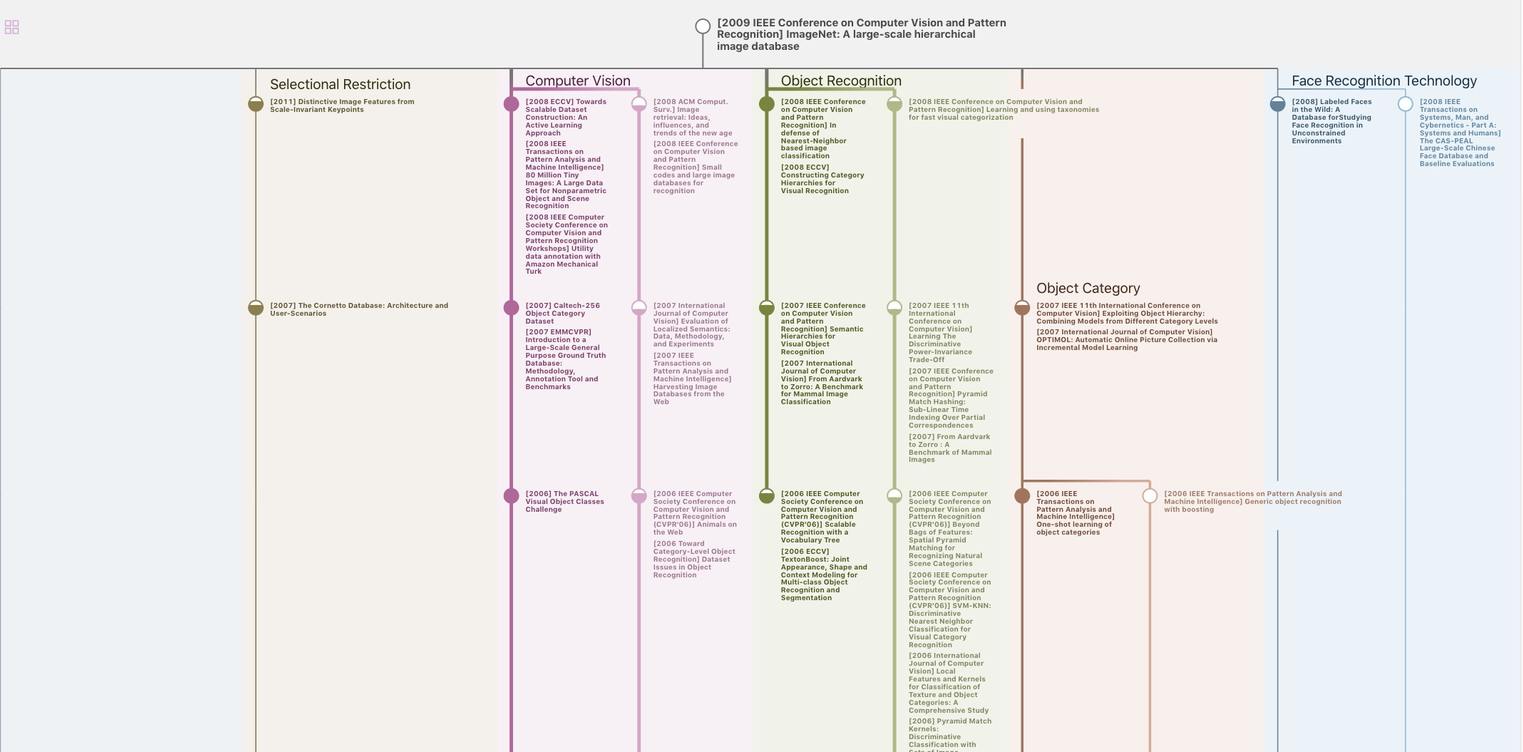
生成溯源树,研究论文发展脉络
Chat Paper
正在生成论文摘要