Swin transformer based transfer learning model for predicting porous media permeability from 2D images
COMPUTERS AND GEOTECHNICS(2024)
摘要
Soil and rock, as typical porous media, widely exist in natural slopes and landslides and underground reservoirs. Accurate predicting the permeability of porous media is crucial in preventing geological disasters and extracting underground energy. The conventional methods for obtaining the porous media permeability involve experiments or numerical simulations, both of which are usually time-consuming. In recent years, a new approach to predicting the porous media permeability has emerged, utilizing porous media images and leveraging deep learning algorithms. However, previous image -based permeability prediction frameworks have been dominated by convolutional neural networks (CNNs). CNNs require extensive data and intricate network architectures to achieve reasonably accurate predictive performance. State-of-the-art neural networks and methods are employed to overcome the shortcomings of CNNs. To initiate this research, we generated 1200 two-dimensional porous media images. The permeability labels for the target porous media were acquired through computational fluid dynamics. Subsequently, an end -to -end permeability prediction surrogate model was established based on the Swin Transformer architecture. The proposed model falls under the category of transfer learning models, where pre -trained weights and bias from other datasets serve as the initial weights and bias for our dataset. Our model has achieved State-of-the-art performance, outperforming CNN by a significant margin on small -size datasets. Even with a small dataset size as 200, the proposed model still achieves an R2 score of 0.9717 on the testing set. Both transfer learning and the Swin Transformer architecture have demonstrated robust predictive capabilities in the context of predicting permeability in small -size dataset porous media based on computer vision.
更多查看译文
关键词
Deep learning,Transfer learning,Permeability prediction,Swin transformer,Computer vision,Porous media
AI 理解论文
溯源树
样例
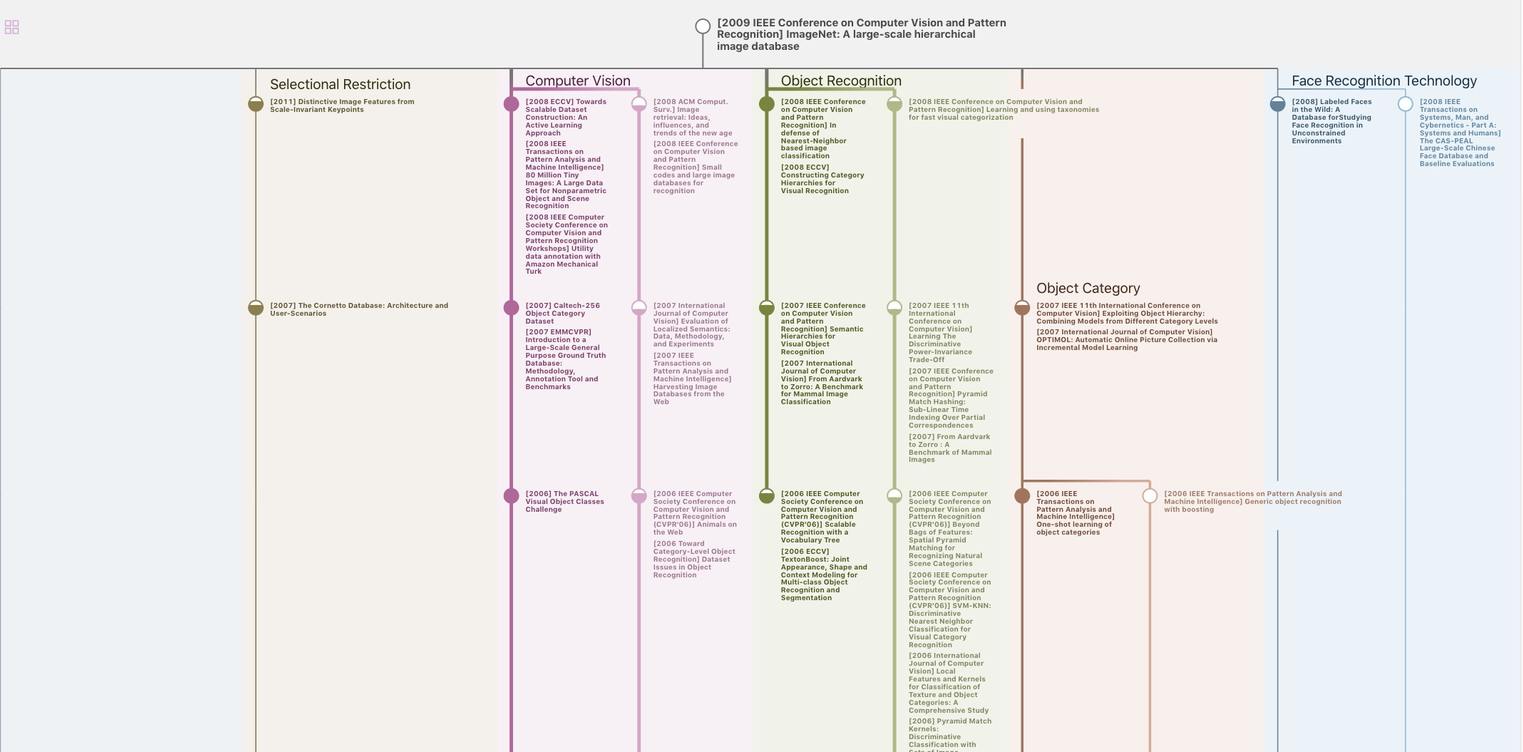
生成溯源树,研究论文发展脉络
Chat Paper
正在生成论文摘要