Dynamic ensemble of regression neural networks based on predictive uncertainty
COMPUTERS & INDUSTRIAL ENGINEERING(2024)
摘要
In a data -decentralized environment where data are distributed across individual local nodes, inter -node data sharing is often restricted due to security concerns and communication costs. Under these restrictions, using entire data to train a global predictive model is challenging. A viable strategy is to leverage local predictive models trained independently on local nodes. However, this adversely affect generalization performance, particularly when the data distribution varies between local nodes. To address this issue in regression problems, we propose a dynamic ensemble method that combines the predictions of locally trained neural networks without the need to access data from local nodes. For a query instance, the prediction and its uncertainty from each neural network are computed by applying Monte Carlo dropout. Subsequently, smaller/larger weights are assigned to neural networks with higher/lower predictive uncertainty. The final prediction result is derived using the weighted average of the individual predictions. The proposed method is applicable to a data -decentralized environment where data are inaccessible and thus cannot be shared across local nodes. Transmitting query instances to local nodes and receiving predictions and weights from the local nodes are the only requirements, thereby ensuring low communication costs. Experimental results on simulated data -decentralized environments using benchmark regression datasets validated the superior generalization performance of the proposed method compared with baseline methods. The proposed method was found to be more effective for regression neural networks that demonstrate higher performance at their respective local nodes.
更多查看译文
关键词
Regression,Dynamic ensemble,Neural network,Uncertainty quantification,Decentralized environment
AI 理解论文
溯源树
样例
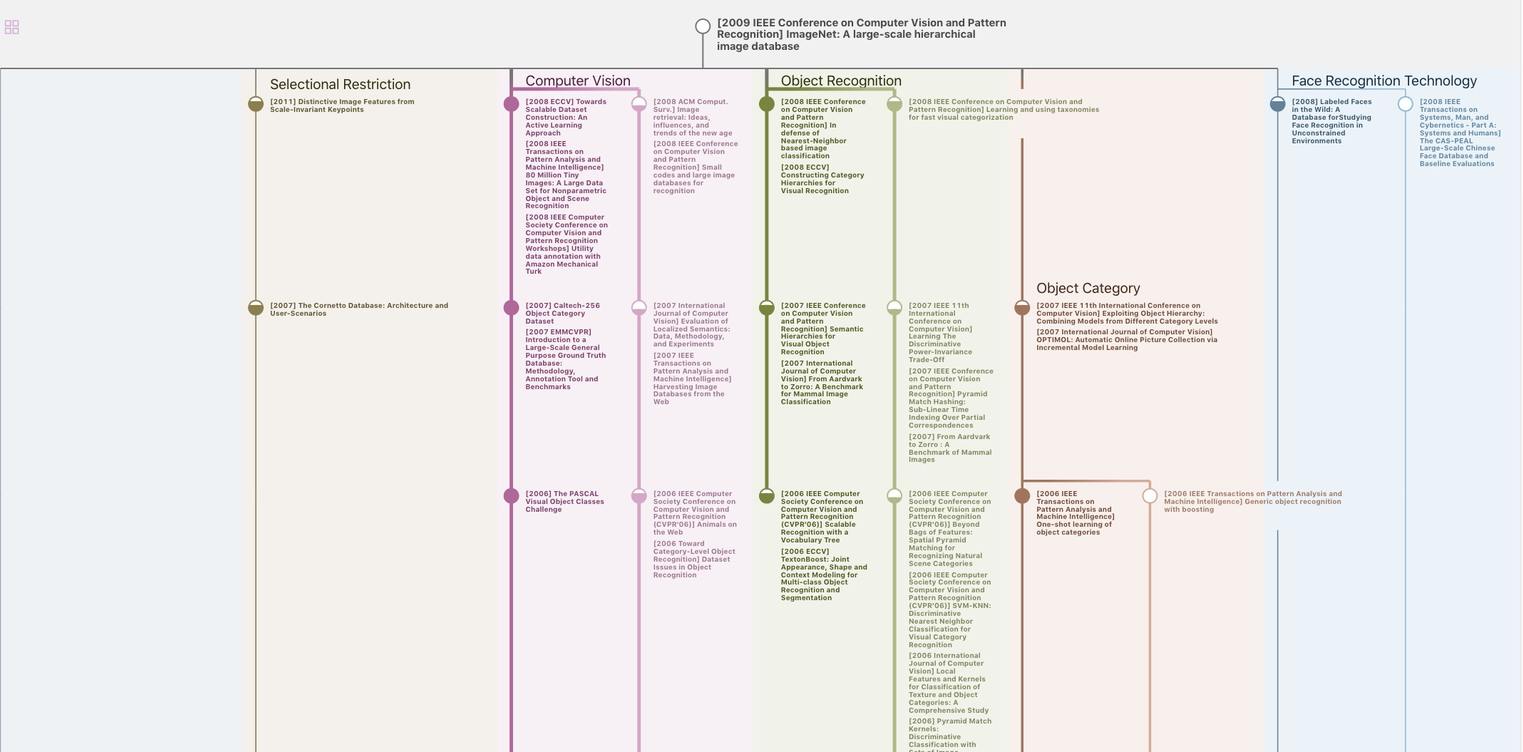
生成溯源树,研究论文发展脉络
Chat Paper
正在生成论文摘要