A multi-step fast charging-based battery capacity estimation framework of real-world electric vehicles
Energy(2024)
摘要
Accurately evaluating battery degradation is not only crucial for ensuring the safe and reliable operation of electric vehicles (EVs) but also fundamental for their intelligent management and maximum utilization. However, the non-linearity, non-measurability, and multi-stress coupled operating conditions have posed significant challenges for battery health prediction. This paper proposes a battery capacity estimation framework based on real-world operating data. Firstly, a comprehensive feature pool is constructed from the direct external features extracted during multi-step fast charging processes and the quantitative representation of operating conditions. Subsequently, a two-step feature engineering is introduced to select the most relevant features and eliminate the interference components. The battery capacity estimation framework is then implemented using machine learning methods. Validation results demonstrate that the proposed framework achieves superior estimation accuracy with lower computational expense compared to the modelling process without feature engineering. The MAPE and RMSE reach 1.18% and 1.98 Ah, respectively, representing reductions in errors of up to 8.53% and 11.21%. Collectively, the proposed framework paves the foundation for online health prognostics of batteries under practical operating conditions.
更多查看译文
关键词
Lithium-ion battery,Capacity estimation,Multi-step fast charging,Machine learning,Real-world data
AI 理解论文
溯源树
样例
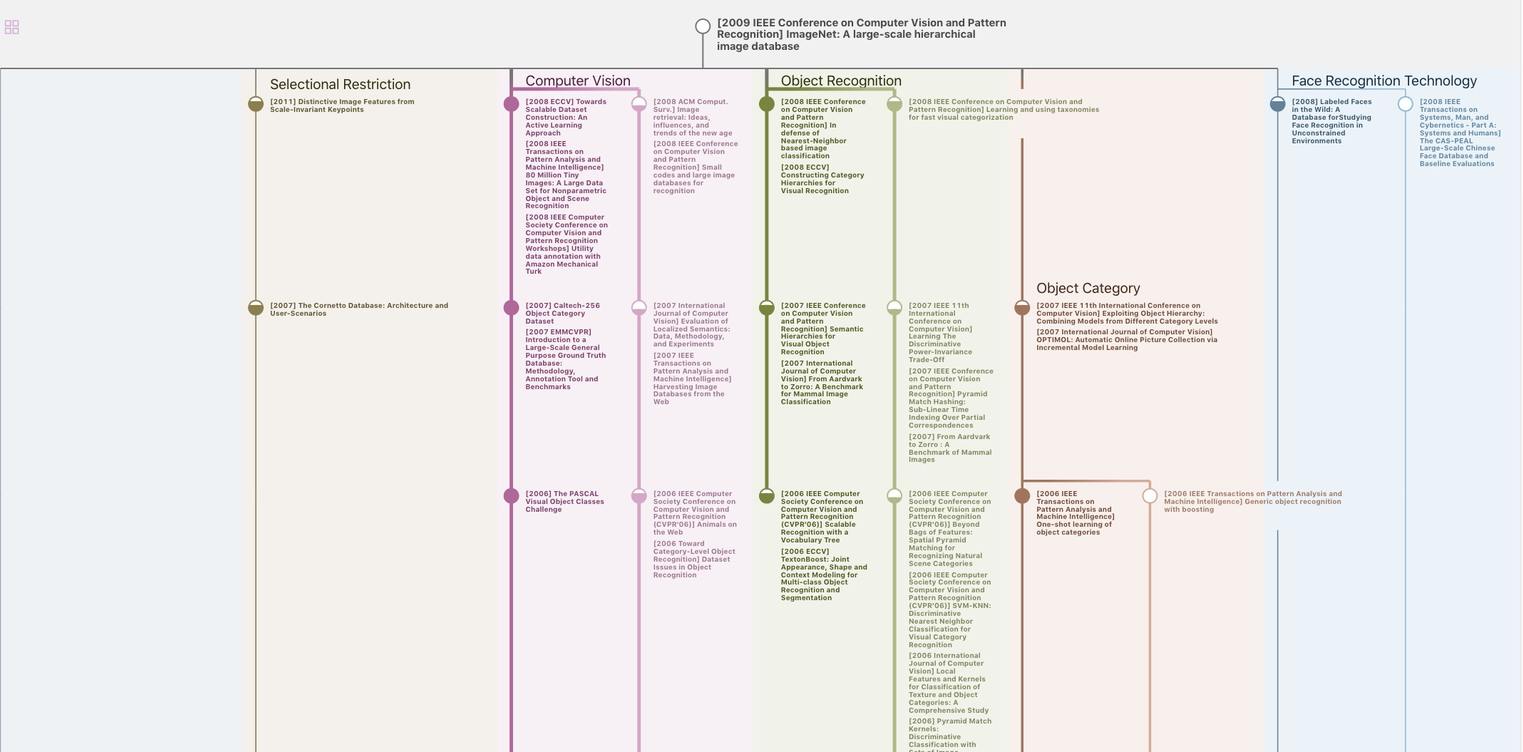
生成溯源树,研究论文发展脉络
Chat Paper
正在生成论文摘要