A method for estimating the state of health of lithium-ion batteries based on physics-informed neural network
ENERGY(2024)
摘要
Data-driven methods have been widely used to estimate the State of health (SOH) of Lithium-Ion batteries (LIBs). However, these methods lack interpretability. In response to this issue, this article proposes a method called Physics-informed neural network (PIFNN) to enhance the interpretability of predictions made by a feedforward neural network (FNN). First, the features are extracted from incremental capacity (IC) curves and differential temperature curves, which can characterize battery aging from different perspectives. Specifically, the peaks of the IC curves (P-IC) reflect the electrochemical reactions that occur during the charge-discharge processes of LIBs. The decline of the P-IC is related to the loss of active materials in LIBs, which is a major cause of the decrease of the SOH. This article converts the monotonous relationship between the P-IC and the SOH into physical constraints to guide the "learning process" of the model. In the prediction process, a physics-constrained secondary "training" is applied to the FNN predictions to further enhance interpretability and improve prediction accuracy. The feasibility of the proposed method is validated using the Oxford and NASA battery datasets. The results indicate that PIFNN effectively improves prediction accuracy and reduces errors to below 1.5 %.
更多查看译文
关键词
Lithium -ion battery,State of health,Neural network,Physical constraints,Incremental capacity curves
AI 理解论文
溯源树
样例
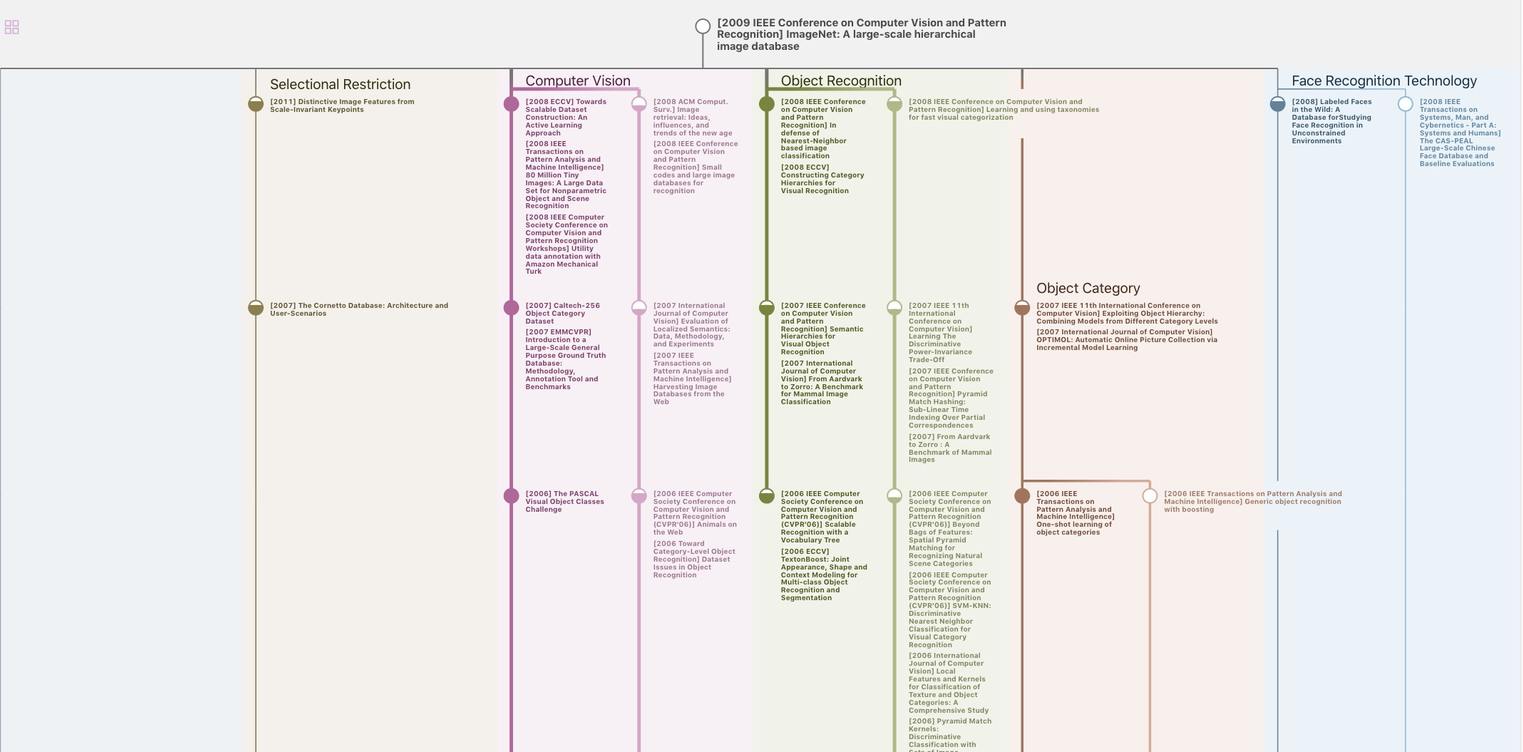
生成溯源树,研究论文发展脉络
Chat Paper
正在生成论文摘要