Data-physics-driven estimation of battery state of charge and capacity
ENERGY(2024)
摘要
High -power density lithium -ion batteries have been utilized in both energy storage and high rate charging and discharging applications. Accurate state estimation is fundamental to enhancing battery life and safety. Therefore, a data -physics -driven estimation of the state of charge and capacity for lithium-titanate batteries was conducted using Gaussian distribution fusion. Firstly, a fractional order model was selected as the physical analytical model for lithium-titanate batteries. Secondly, a data -driven model that combines convolutional neural networks and long short-term memory networks was employed to predict the battery state of charge. Thirdly, the physical analytical model and data -driven model were fused to estimate the state of charge by employing the principle of Gaussian distribution fusion. Finally, both the state of charge and actual capacity of the battery were jointly estimated. The results showcase the capability of the proposed method to accurately estimate the state of charge and capacity, ensuring an accuracy level within 1%. Furthermore, this approach exhibits a 20% improvement in accuracy compared to traditional methods.
更多查看译文
关键词
Lithium-ion battery,State of charge estimation,Capacity estimation,Gaussian fusion distribution
AI 理解论文
溯源树
样例
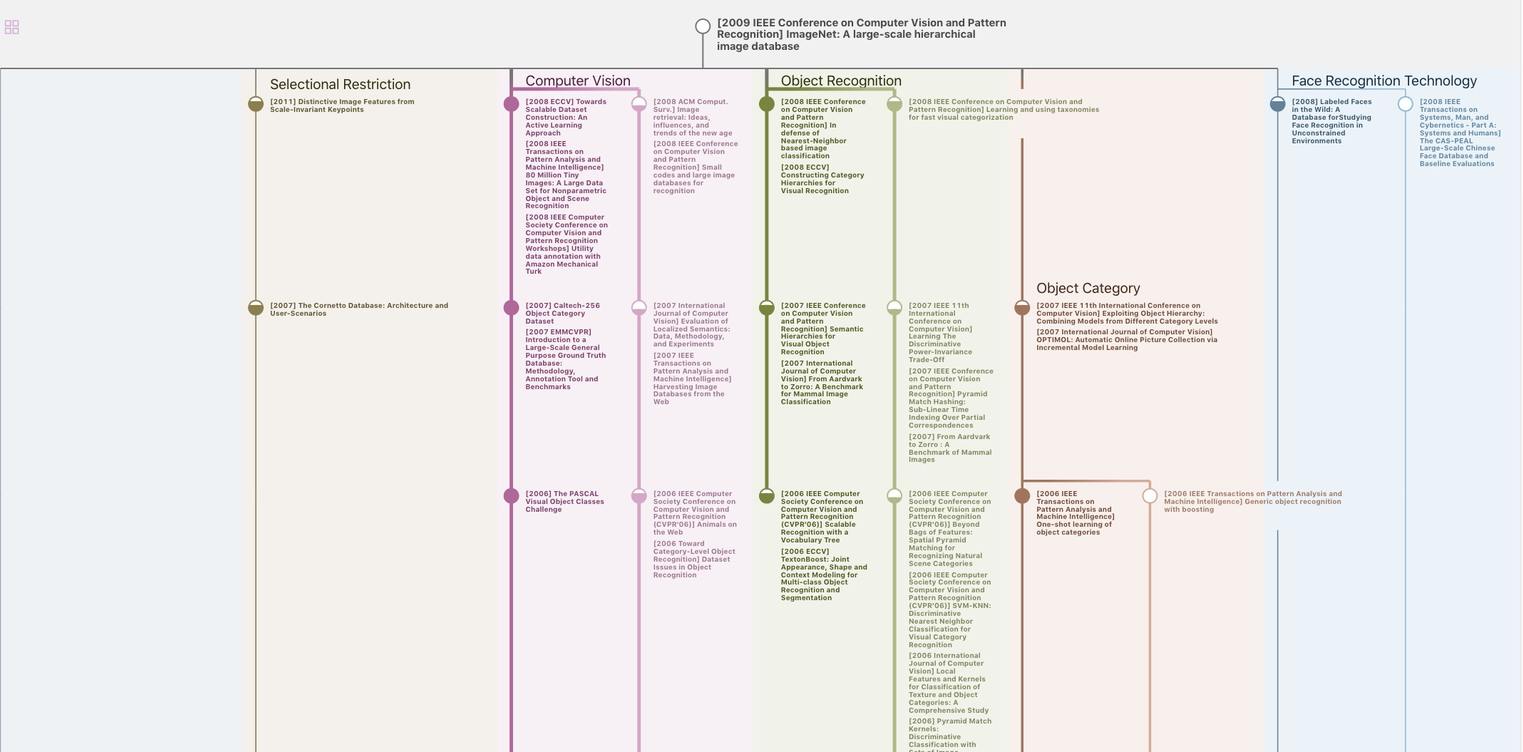
生成溯源树,研究论文发展脉络
Chat Paper
正在生成论文摘要