Advanced integration of ensemble learning and MT-InSAR for enhanced slow-moving landslide susceptibility zoning
ENGINEERING GEOLOGY(2024)
摘要
The Three Gorges Dam's operation has been recognized as a contributing factor to slope instability and the reactivation of pre-existing deep-seated landslides in the region. Regular human activities, including the regulation of the Yangtze River water level, urban development, and infrastructure expansion, combined with heavy rainfall, dynamically alter the state of existing slow-moving landslides and can provoke new slope failures. This study introduces a comprehensive approach aimed at assessing the susceptibility associated with potential reactivations or accelerations of pre-existing deep-seated landslides in Dazhou town, located in Wanzhou District's northern area. The approach encompasses a synthesis of ensemble learning models and non-invasive remote sensing (i.e. multi-temporal interferometric SAR, MT-InSAR) displacement monitoring to ascertain regions prone to slow-moving landslides. The addressed key challenges include: (i) the integration of three distinct ensemble algorithms-boosting, bagging, and stacking-to enhance the predictive precision of the first-level landslide susceptibility zonation and (ii) MT-InSAR data analysis, which allows the generation of kinematic indicators used to derive a second-level enhanced susceptibility zonation. The investigation primarily focuses on slope units, deemed critical for susceptibility zoning. The derived insights are then cross-checked with in-situ landslide data, consolidating the empirical findings. This integrated knowledge is crucial for the development of effective risk mitigation strategies and the advancement of landslide risk management for future scenarios.
更多查看译文
关键词
Slow-moving landslides,Enhanced landslide susceptibility zonation,Stacking-based RF-XGBoost model,MT-InSAR,Three Gorges Reservoir Area
AI 理解论文
溯源树
样例
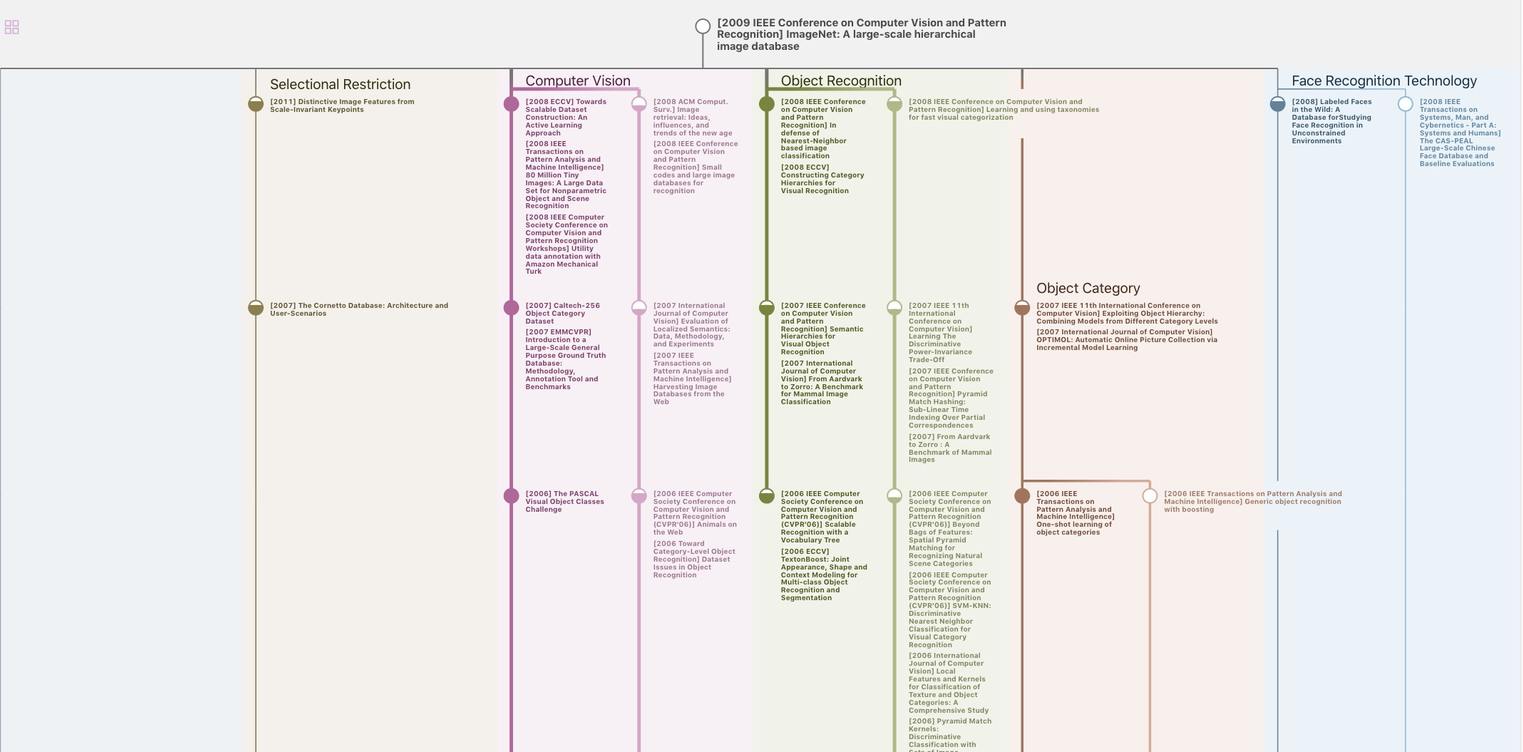
生成溯源树,研究论文发展脉络
Chat Paper
正在生成论文摘要