Filtering offensive language from multilingual social media contents: A deep learning approach
ENGINEERING APPLICATIONS OF ARTIFICIAL INTELLIGENCE(2024)
摘要
In the face of uncontrolled offensive content on social media, automated detection emerges as a critical need. This paper tackles this challenge by proposing a novel approach for identifying offensive language in multilingual, code-mixed, and script-mixed settings. The study presents a novel multilingual hybrid dataset constructed by merging diverse monolingual and bilingual resources. Further, we systematically evaluate the impact of input representations (Word2Vec, Global Vectors for Word Representation (or GloVe), Bidirectional Encoder Representations from Transformers (or BERT), and uniform initialization) and deep learning models (Convolutional Neural Network (or CNN), Bidirectional Long Short Term Memory (or Bi-LSTM), Bi-LSTMAttention, and fine-tuned BERT) on detection accuracy. Our comprehensive experiments on a dataset of 42,560 social media comments from five languages (English, Hindi, German, Tamil, and Malayalam) reveal the superiority of fine-tuned BERT. Notably, it achieves a macro average F1-score of 0.79 for monolingual tasks and an impressive 0.86 for code-mixed and script-mixed tasks. These findings significantly advance offensive language detection methodologies and shed light on the complex dynamics of multilingual social media, paving the way for more inclusive and safer online communities.
更多查看译文
关键词
Social media,Offensive language,Machine learning,Offensive content,Deep learning,Multilingual and bilingual,Hate speech,Code-mixed
AI 理解论文
溯源树
样例
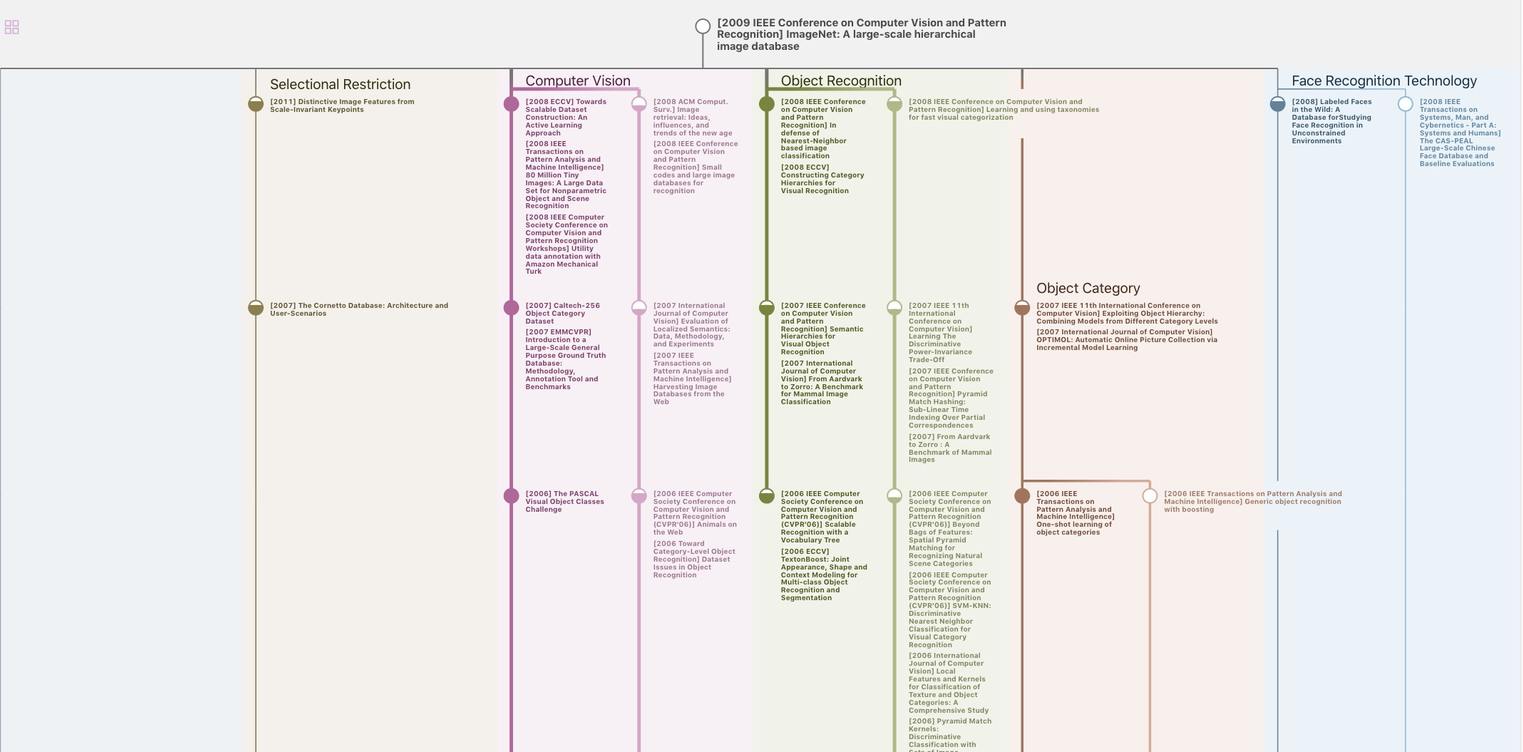
生成溯源树,研究论文发展脉络
Chat Paper
正在生成论文摘要