A Multi-User-Multi-Scenario-Multi-Mode aware network for personalized recommender systems
ENGINEERING APPLICATIONS OF ARTIFICIAL INTELLIGENCE(2024)
摘要
User personalized recommendation is increasingly vital in many industrial applications. How to precisely mine user's dynamic interests from multiple scenarios is a challenge task in Click -Through Rate (CTR) prediction. Existing works obtain representations based on feature engineering from training data. However, the CTR models are often sensitive to different users and scenarios. To address these concerns, we propose a novel approach, Multi -User -Multi -Scenario -Multi -Mode (MUMSMM), which learns user personalized preference from history behavior. Specifically, we adapt the cognitive gate -net, which can screen applicable networks automatically for different users and scenarios. Then, we design a feature mechanism to recognize valuable information, while also having an adaptive gate to optimize the hyper -parameters among tasks. Besides, we propose a novel interest unit, which can capture the user diverse interests and adjust the intensity. Furthermore, to generate a more stable interest representation, we employ transformer network. Our innovative approach can be flexibly embedded into the mainstream recommended CTR model. Extensive experiments on three public benchmark datasets (Alibaba, Amazon, and JD) show that the proposed MUMSMM model outperforms state -of- the -art models by an average improvement of +2.49% in AUC (Area Under Curve) and +4.33% of HR@10 (Hits Rate), respectively.
更多查看译文
关键词
Personalized aware network,Adaptive gate,Adaptive feature,Novel interest unit,Click through rate,User behavior modeling,Multi-user-multi-scenario-multi-mode
AI 理解论文
溯源树
样例
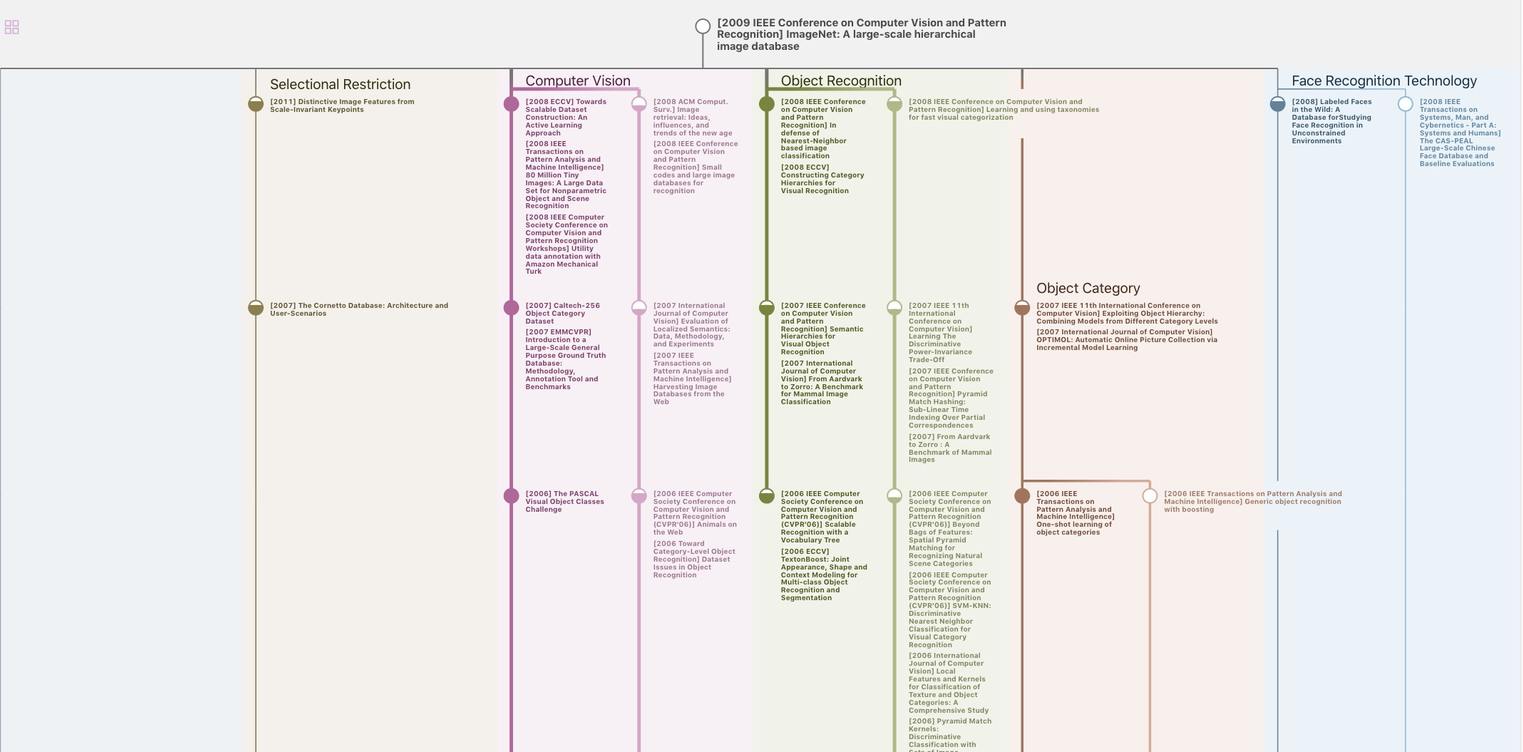
生成溯源树,研究论文发展脉络
Chat Paper
正在生成论文摘要