Within-season crop yield prediction by a multi-model ensemble with integrated data assimilation
FIELD CROPS RESEARCH(2024)
摘要
Improving crop yield prediction accuracy is crucial for sustainable agriculture. One approach is to use data assimilation (DA) techniques based on satellite remote sensing, which can help improve predictions at the regional to national scale. However, the interaction between uncertain crop model inputs and DA, as well as the impact of crop model structure on DA results, have received little attention to date. In this work, we assimilated leaf area index (LAI) data into three single crop models (CERES, GECROS, and SPASS) as well as into their multimodel ensemble (MME) using a particle filtering (PF) algorithm. Mimicking the common lack of information at a large scale, we considered nitrogen fertilization, sowing date, soil hydraulic parameters, and weather data as the sources of uncertainties. In a case study, we applied this setup to six winter wheat site years in southwestern Germany. Before applying DA, all models were calibrated and validated using in-situ measured data from a multi-site, multi-year independent data set. The model performance in the calibration was used to assign weights to the models of the MME. Results show that weather data and soil hydraulic parameters had the highest impact on all model predictions. DA substantially improved the accuracy and precision of LAI simulation in all models. Moreover, DA enhanced grain yield prediction by GECROS, SPASS, and the multi-model ensemble, but had no considerable effect on CERES. Specifically, the bias in yield prediction decreased from 25% to 15% in the case of GECROS, from 26% to 15% in SPASS, and from 19% to 7% in the MME. In contrast, even without DA, the yield prediction error in CERES was below 5%. The correlation between LAI errors and yield errors was a key factor indicating how DA can be effective on a specific model. When the correlation analysis is unavailable, the multimodel ensemble is a promising approach for data assimilation. Further investigations on regional model calibration, input uncertainty, MME size, and model weighting scheme are necessary to improve the performance of data assimilation applications.
更多查看译文
关键词
LAI assimilation,Particle filtering,Process-based crop model,Uncertainty analysis,Yield forecast,Multi-model ensemble
AI 理解论文
溯源树
样例
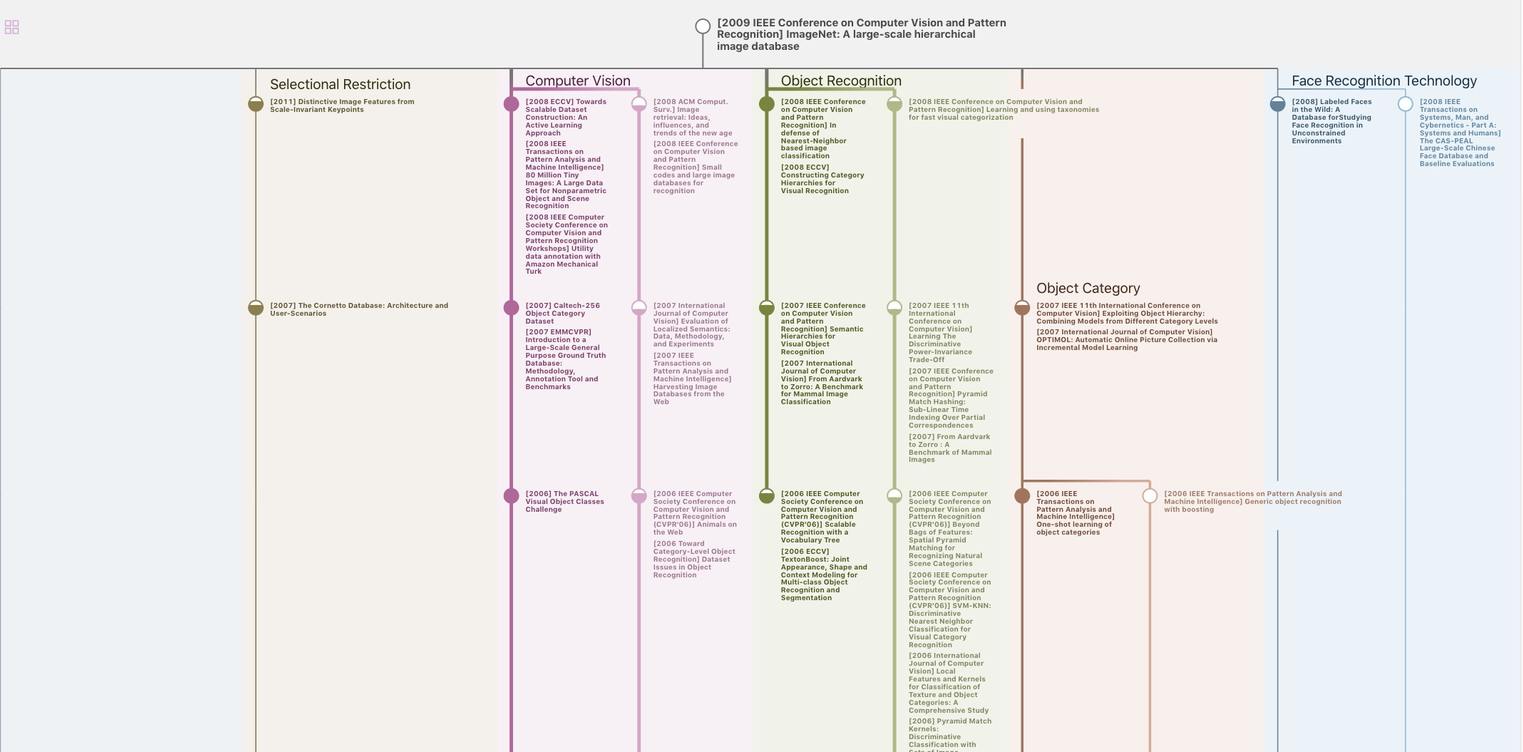
生成溯源树,研究论文发展脉络
Chat Paper
正在生成论文摘要