AMSC-Net: Anatomy and Multi-Label Semantic Consistency Network for Semi-Supervised Fluid Segmentation in Retinal OCT
Expert systems with applications(2024)
摘要
Automated segmentation of pathological fluid regions is crucial for digital diagnosis and individualized therapy under optical coherence tomography (OCT) images. Nonetheless, methods that rely on enormous annotations impede their clinical applications, as pixel-wise fine-grained labels are extraordinarily costly and require the expertise of ophthalmology. Though there exist works based on consistency-based and pseudo-label-based methods for reducing annotation reliance, the underutilization of consistency mechanisms and ignorance of fluid anatomical structures result in sub-optimal segmentation performance. In this work, we proposed a novel AMSC-Net devoted to semi-supervised fluid segmentation and achieves a 73.95% Dice score with 5% labeled data. Concretely, we developed a Heterogeneous Architecture Consistency (HAC) strategy based on our dual decoders enjoying different inductive biases. Moreover, we invented a Multi-label Semantic Consistency Loss (MSC-Loss) module in hierarchical semantic features and an Anatomy Contour Consistency Loss (ACC-Loss) module integrating anatomical constraint. These modules complementarily reinforce the quality of pseudo labels and boost semi-supervised training for robust segmentation results. To verify the superiority and bedside potential of our proposed AMSC-Net, we collected a large-scale fluid segmentation dataset composed of 22517 OCT images. Extensive quantitative and qualitative experiments validated the efficacy of our AMSC-Net with multiple novel techniques. Also, experimental results in a public fluid segmentation dataset demonstrated that our method achieves state-of-the-art performance. Code will be available at: https://github.com/ZeroOneGame/S4_Fluid_OCT.
更多查看译文
关键词
Semi-supervised learning,Fluid segmentation,Semantic consistency,Anatomy consistency,Pseudo label
AI 理解论文
溯源树
样例
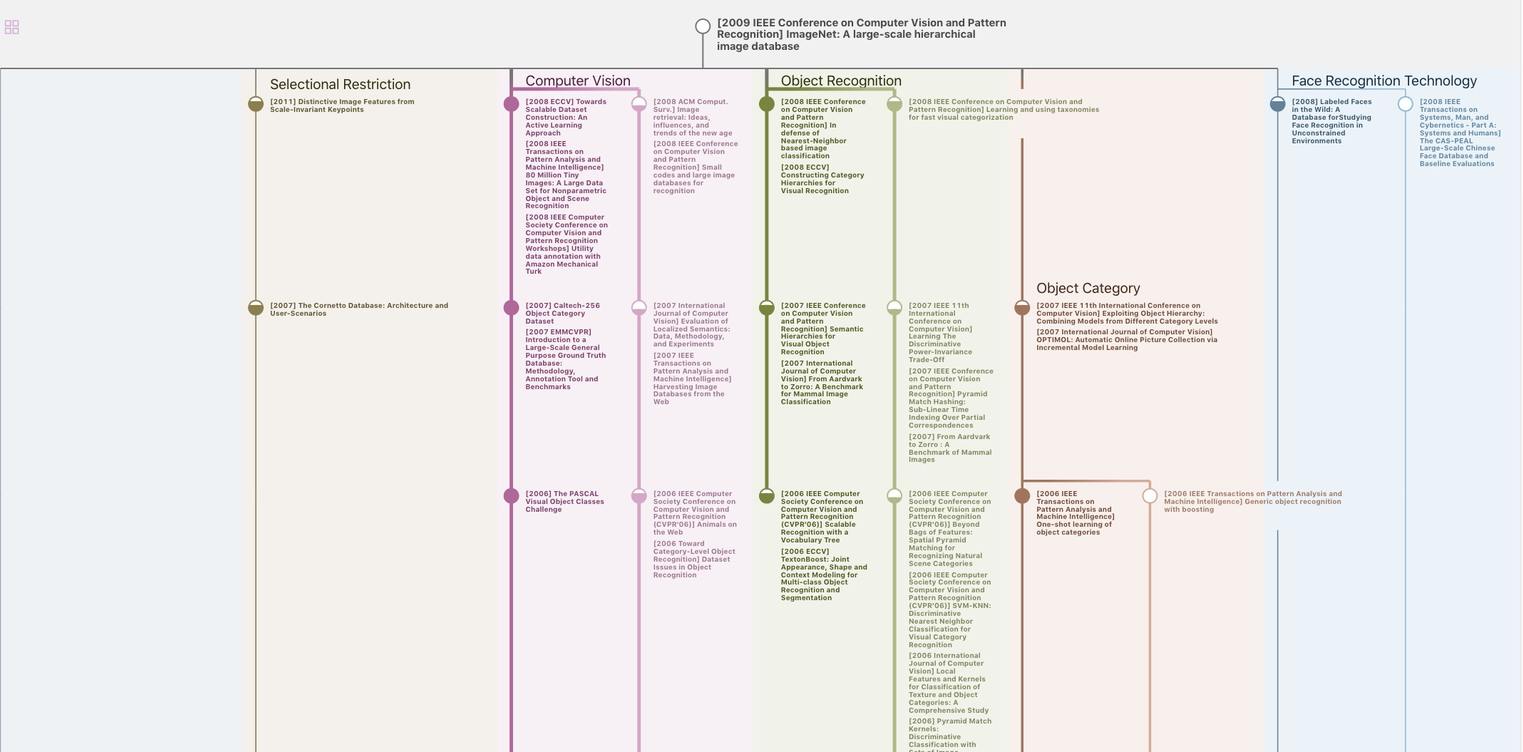
生成溯源树,研究论文发展脉络
Chat Paper
正在生成论文摘要