Modelling long medical documents and code associations for explainable automatic ICD coding
EXPERT SYSTEMS WITH APPLICATIONS(2024)
摘要
Quick and accurate International Classification of Diseases (ICD) code assignment is vital for billing, reimbursement and medical research. Owing to the labour-intensive and error-prone nature of manual coding, automatic ICD coding using deep learning methods has flourished. However, this task is still challenging because of (1) the interpretability of coding, (2) lengthy clinical documents and (3) long-tail label distribution. In the current study, we propose a novel automatic ICD coding framework to address these issues. First, a biomedicalspecific pre-trained language model, Clinical-Longformer, is used as an encoder, which generates meaningful representations of long clinical documents by injecting rich medical knowledge and capturing long-distance dependence among tokens. Second, a decoding architecture that combines the multi-synonym attention mechanism, hierarchical curriculum learning and distribution-balance loss is designed to perform ICD code prediction. The decoder improves the tail-end performance by fully capturing code associations in terms of semantics, structure and co-occurrence. In addition, the label-wise attention mechanism provides the interpretability of prediction. Experimental results on benchmark MIMIC-III datasets indicate that our model achieves higher F1 scores than previous state-of-the-art baselines. Our suggested model is expected to serve as an aid in improving the efficiency of manual ICD coding and to offer insights for other long text classification tasks with multiple label associations.
更多查看译文
关键词
Automatic ICD coding,Multi -label text classification,Long medical documents,Code associations,Interpretability
AI 理解论文
溯源树
样例
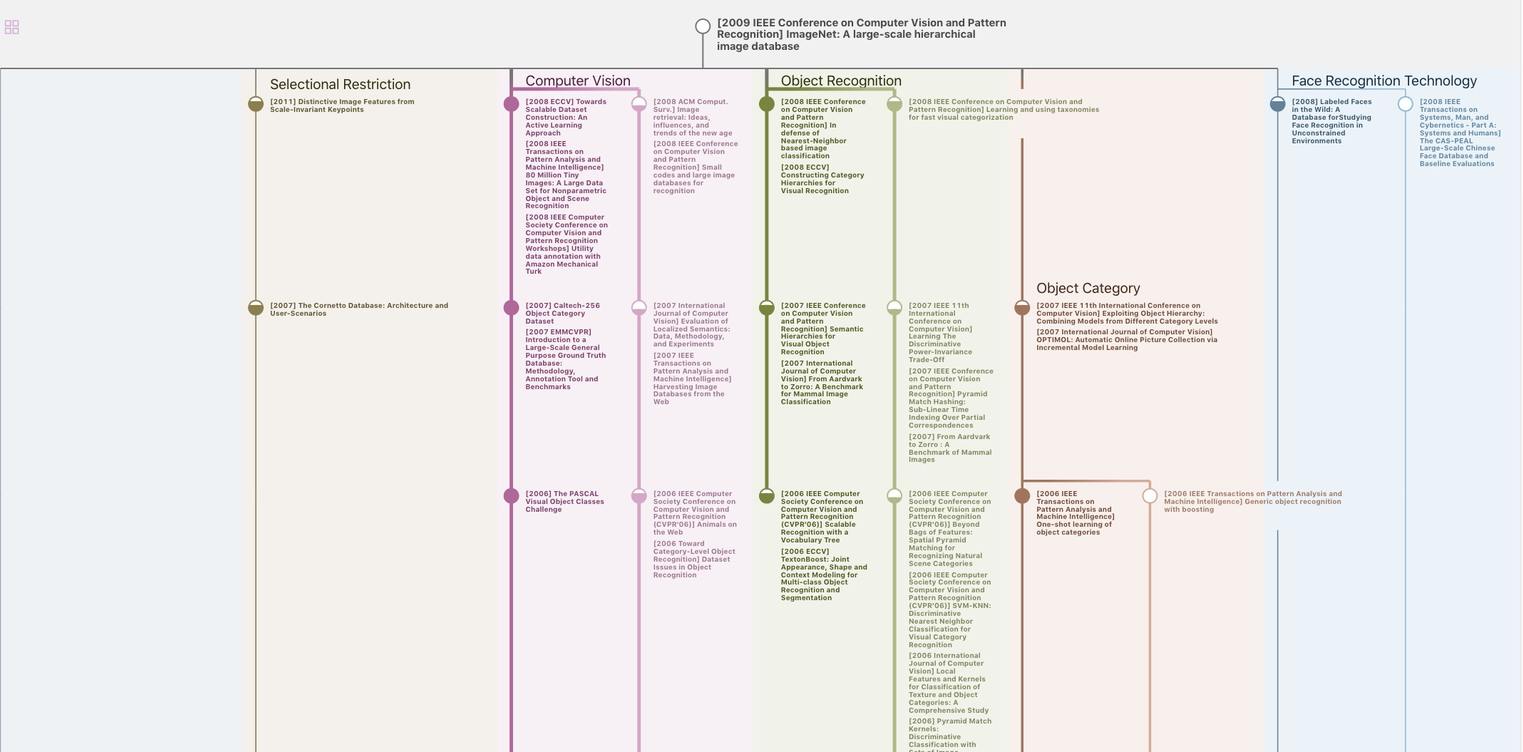
生成溯源树,研究论文发展脉络
Chat Paper
正在生成论文摘要