Optimal demand response based dynamic pricing strategy via Multi-Agent Federated Twin Delayed Deep Deterministic policy gradient algorithm
ENGINEERING APPLICATIONS OF ARTIFICIAL INTELLIGENCE(2024)
摘要
The intermittent integration of renewable energy sources and the enhancement of energy-saving awareness on the demand side have posed significant challenges to energy management in smart grids. To address the supply-demand imbalance issues caused by these challenges, this paper proposes a novel multiple time-scale dispatch model. The model utilizes demand response strategy for demand side management (DSM) and employs dynamic pricing strategy for supply -side management. Furthermore, an advanced approach based on federated learning and Twin Delayed Deep Deterministic(TD3) policy gradient algorithm is proposed to construct a federated learning mechanism among multi agents. This approach performs distributed optimization on the multi-agent system to derive the optimal network weights for multiple stakeholders, resulting in the generation of an optimal dynamic pricing strategy that ensures the economic efficiency and secure operation of the smart grid. From the methodological perspective, this approach enhances privacy protection and decision-making autonomy for each stakeholder, while improving the optimization efficiency of the reinforcement learning (RL) algorithm. Based on simulation results, it is evident that the proposed method facilitates economically efficient transactions between supply and demand, and the improved distributed multi-agent federated reinforcement learning (MAFRL) approach holds promise as a feasible and optimal optimization method for economic dispatch in smart grid.
更多查看译文
关键词
Multi-agent reinforcement learning,Federated learning,Economic dispatch,Privacy security,Overestimation
AI 理解论文
溯源树
样例
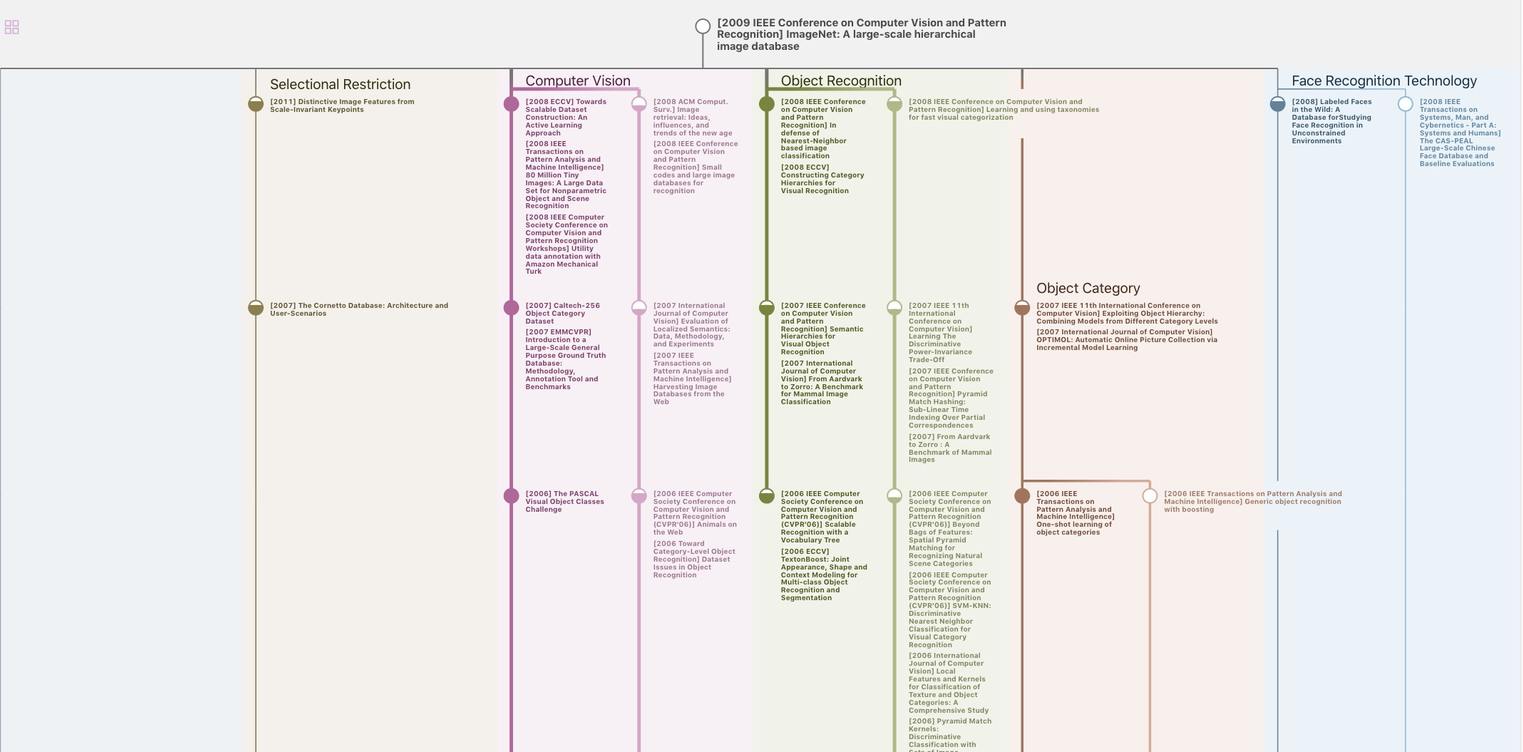
生成溯源树,研究论文发展脉络
Chat Paper
正在生成论文摘要