Relation mapping based on higher-order graph convolutional network for entity alignment
ENGINEERING APPLICATIONS OF ARTIFICIAL INTELLIGENCE(2024)
摘要
Recently, entity alignment for building knowledge graphs (KGs) has gathered increasing interest in the field of knowledge engineering. Existing models that are based on translation embeddings and graph convolutional network (GCN) further promote quality of entity embeddings, but most of them fail to pay attention to the influence of higher -order neighbors. However, higher -order information is strikingly central to perform entity alignment. Although the introduction of relationships between entities can further enhance the alignment, the existing methods have poor quality for relation embeddings. To overcome the issues, we design a novel Relation Mapping based on Higher -order Graph Convolutional Network for entity alignment, named RMHN. Specifically, a novel higher -order GCN is designed to aggregate higher -order information to considerably obtain entity embeddings. Additionally, we design a new relational mapping mechanism to obtain relation embeddings, which can drastically assist in the alignment process. To unlock the critical bottleneck that the current sampling strategies do not substantially improve the performance of entity alignment, we propose a new adversarial sampling strategy. Finally, experimental results on benchmark datasets exhibit the RMHN model surprisingly outperforms the state-of-the-art models.
更多查看译文
关键词
Entity alignment,Higher-order,Relation mapping,Adversarial sampling
AI 理解论文
溯源树
样例
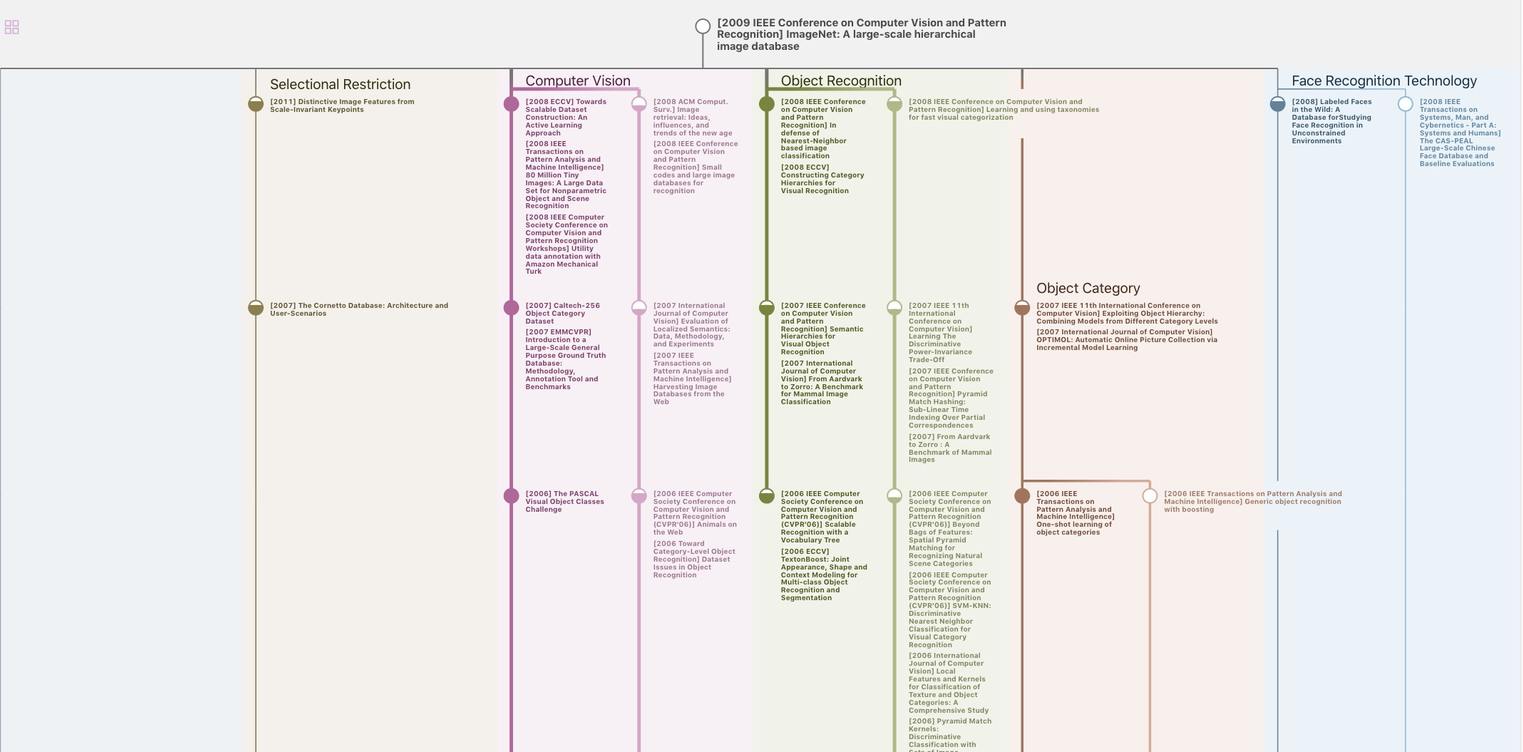
生成溯源树,研究论文发展脉络
Chat Paper
正在生成论文摘要