SwinT-SRNet: Swin transformer with image super-resolution reconstruction network for pollen images classification
ENGINEERING APPLICATIONS OF ARTIFICIAL INTELLIGENCE(2024)
摘要
With the intensification of urbanization in human society, pollen allergy has become a seasonal epidemic disease with a considerable incidence rate, seriously affecting the healthy life of residents. Accurately classifying and recognizing major allergenic pollens for effective pollen monitoring and forecasting is of great practical significance for improving urban livability and citizens' quality of life. With the development of deep learning, automatic classification gradually replaces the process of manually recognizing pollen grains. Recently, Swin Transformer (SwinT) has demonstrated strong competitiveness in various tasks. In order to solve the problem of low resolution and complex background information of pollen images, we propose a novel classification framework titled Swin Transformer with Image Super -resolution Reconstruction Network (SwinTSRNet) for pollen images classification. In the proposed SwinT-SRNet network, an image super -resolution reconstruction method based on the Efficient Super -resolution Transformer (ESRT) is designed to eliminate the blurring problem that arises when resizing low -resolution images to fit the training dimensions of the SwinT model. Furthermore, a high -frequency (HF) information extraction module is proposed to capture highfrequency information in images to provide richer information for the SwinT-SRNet classification network. Extensive experimental evaluations on a self -constructed allergic pollen dataset (POLLEN8BJ) in Beijing, China, as well as a public pollen dataset POLLEN20L-det, show that the SwinT-SRNet model achieves remarkable accuracies of 99.46% and 98.98%. Notably, even without pre -training weights, the model achieved 98.57% and 98.31% accuracy on the POLLEN8BJ and POLLEN20L-det datasets, which are 1.05% and 1.19% higher than SwinT, respectively.
更多查看译文
关键词
Pollen images classification,Swin transformer,Image super-resolution,Deep learning
AI 理解论文
溯源树
样例
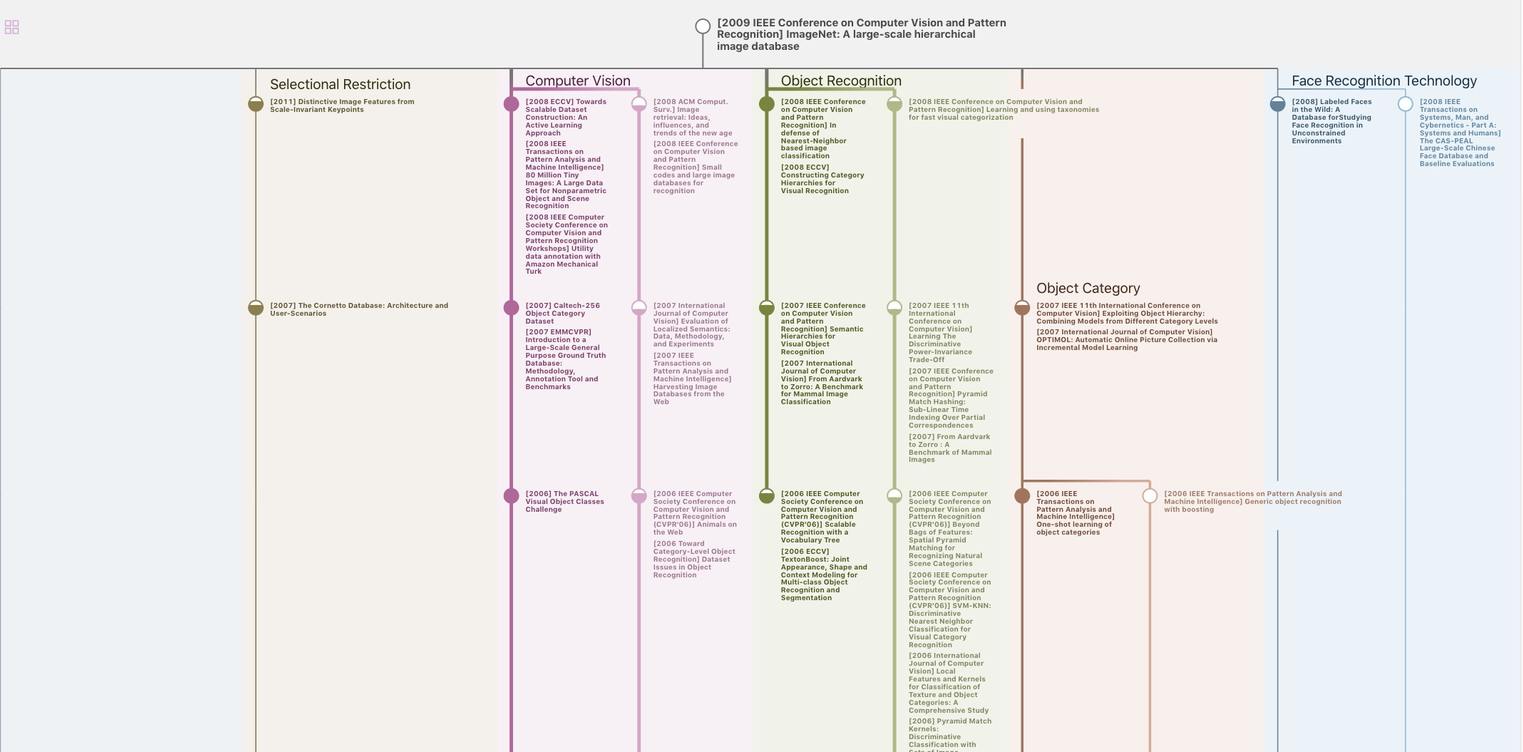
生成溯源树,研究论文发展脉络
Chat Paper
正在生成论文摘要