Landslide displacement prediction with step-like curve based on convolutional neural network coupled with bi-directional gated recurrent unit optimized by attention mechanism
ENGINEERING APPLICATIONS OF ARTIFICIAL INTELLIGENCE(2024)
摘要
This study aims to accurately predict landslide displacement characterized by a step -like displacement curve, resulting from complex interactions among multiple factors, including periodic and variable elements. Specifically, we propose a framework based on a Convolutional Neural Network (CNN) and optimized Bidirectional Gated Recurrent Unit (BiGRU) with an Attention mechanism, designed to forecast landslide displacement with a step -like curve. Initially, landslide displacements are decomposed into periodic and trend terms utilizing complementary ensemble empirical mode decomposition with adaptive noise (CEEMDAN) algorithm. Subsequently, a CNN layer is constructed to extract intricate high -dimensional features, while a BiGRU layer is established to capture temporal dependencies within historical sequences. Notably, an attention mechanism module is introduced to enhance the impact of key information within rainfall, water level, and historical displacement data. Finally, the nonlinear weighted Huber loss function (NLWHL) evaluation method is introduced to assess the accuracy of the model in predicting mutation states. The results demonstrate that the proposed framework exhibited superior accuracy in predicting landslide displacement in comparison to alternative intelligent algorithms. Regarding total displacement, the CNN-BiGRU-Attention model demonstrates superior predictive capabilities, reducing RMSE values by 12.52%, 14.15%, 15.58%, 21.68%, 21.84%, 32.81%, 58.10%, and 53.81% in the test set compared to CNN-BiGRU, CNN-BiLSTM, BiGRU-Attention, SMA-GRU, SMA-LSTM, SMA-SVM, GRU, and LSTM, respectively. Furthermore, the introduction of NLWHL underscores the remarkable accuracy of the proposed framework in forecasting both creep and mutation states. Assessing the displacement mutation state provides the necessary opportunity for early detection and intervention.
更多查看译文
关键词
Landslide monitoring,Baishuihe landslide,Complementary ensemble empirical mode,decomposition with adaptive noise,Machine learning,Deep learning,Nonlinear weighted huber loss function
AI 理解论文
溯源树
样例
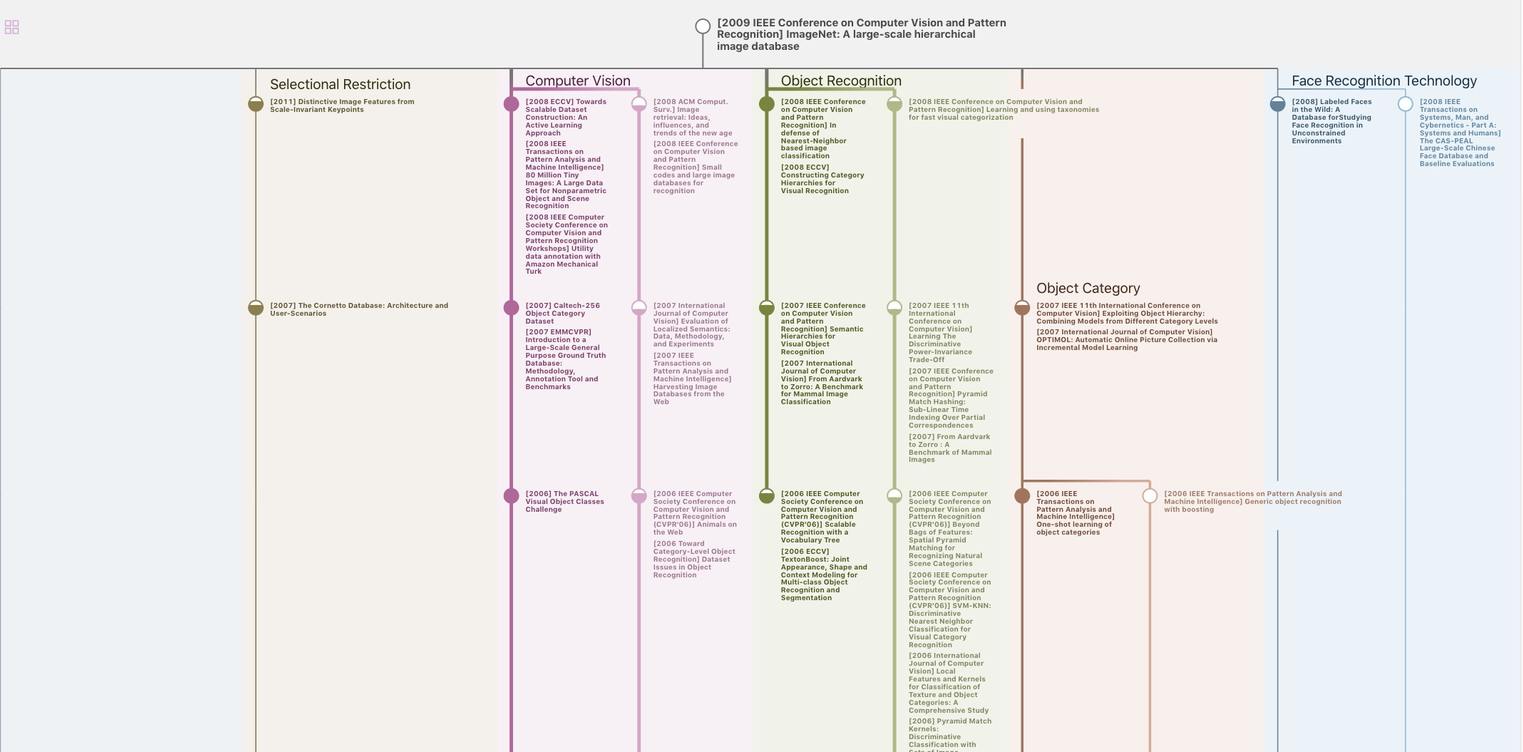
生成溯源树,研究论文发展脉络
Chat Paper
正在生成论文摘要