An approach based on maximal cliques and multi-density clustering for regional co-location pattern mining
EXPERT SYSTEMS WITH APPLICATIONS(2024)
摘要
Spatial co-location pattern (SCP) mining aims to mine the implicit relationships between different spatial features. These features often have certain connections and co-occur in close geographical proximity. Regional colocation pattern (RCP) mining is a branch of SCP mining, which is usually used to discover some sets of spatial features that do not often co-occur in large spatial scales but co-occur in local regions. Discovering RCPs is still very challenging, because different RCPs will be obtained under different region partitions. However, existing region division methods still suffer from ignoring the influence of the density of individual feature instances, low recognition rate of regions with low density distribution but containing RCPs, and lack of semantic information in the delineated regions. To this end, first, we propose a novel multi-density clustering method based on maximal cliques (MCs) during the partitioning phase of RCP mining. Second, we design a two-stage mining algorithm based on MCs in the mining phase, which fully exploits the advantages of the MC to improve the mining efficiency, and the algorithm can quickly obtain new mining results when changing the prevalence threshold. Third, regional similarity is defined based on RCPs over regions to merge similar sub-regions. Finally, the proposed method is compared with a state-of-the-art method on both synthetic and real datasets. The experimental results show that the proposed method can not only effectively solve the issues of existing methods to make the divided sub-regions more closely matched with the real spatial distribution, but also quickly obtain new mining results when changing the prevalence threshold.
更多查看译文
关键词
Spatial data mining,Regional co -location pattern,Multi -density clustering,Maximal clique
AI 理解论文
溯源树
样例
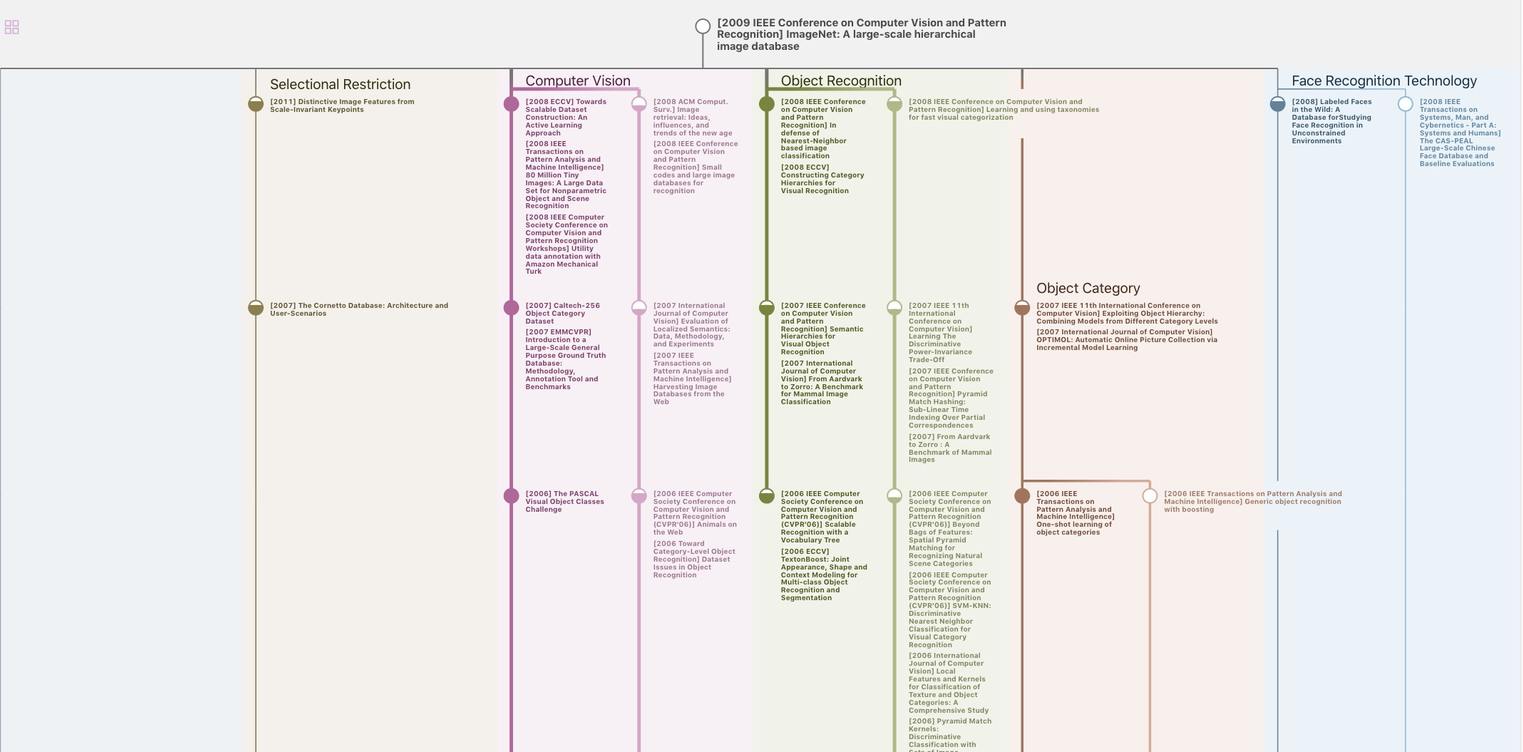
生成溯源树,研究论文发展脉络
Chat Paper
正在生成论文摘要