Granulation-based long-term interval prediction considering spatial-temporal correlations for gas demand prediction in the steel industry
EXPERT SYSTEMS WITH APPLICATIONS(2024)
摘要
In steel enterprises, the long-term interval prediction of downstream gas demand plays an increasingly significant role in the scheduling decision-making of gas supply networks. Accurate long-term interval prediction remains a challenge owing to the intermittent and fluctuating characteristics of ironmaking and steelmaking. In this paper, a novel granulation-based interval prediction model (STGLUBE) is developed, which considers various data granule features and spatial-temporal correlated features. Granular evaluation indices and a corresponding training method are designed for STGLUBE training. A hybrid long-term interval prediction method integrated with a multiscale decomposition and error correction mechanism is further proposed to satisfy the actual requirements. In this method, an empirical wavelet transform is used to extract simplified modes from the original gas demand. The proposed STGLUBE is implemented in the primary mode, whereas the point predictions are performed in other modes. In addition, an error correction mechanism is introduced to enhance prediction accuracy. The effectiveness of the proposed method is verified by conducting two industrial case studies, and the results illustrate that the proposed method outperforms the existing widely used methods.
更多查看译文
关键词
Gas supply network,Long-term interval prediction,Granulation -based prediction,Spatial -temporal correlation
AI 理解论文
溯源树
样例
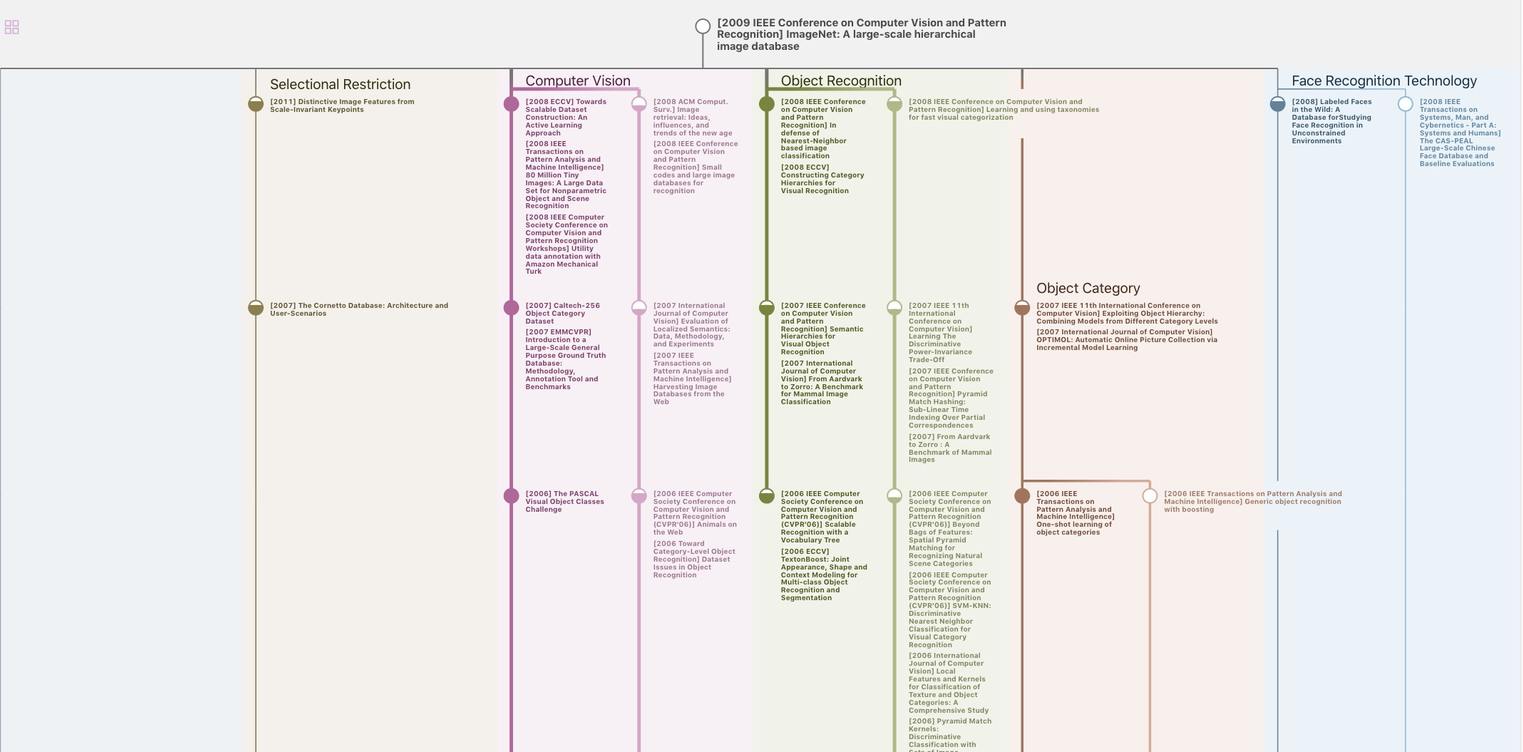
生成溯源树,研究论文发展脉络
Chat Paper
正在生成论文摘要