Transformer-based sensor failure prediction and classification framework for UAVs
EXPERT SYSTEMS WITH APPLICATIONS(2024)
摘要
Unmanned aerial vehicles (UAVs) heavily rely on sensors to perceive their environment, ensuring safe flight operations. However, sensor failures can occur unexpectedly, jeopardizing flight safety. To address this issue, we propose an innovative real-time solution for diagnosing and preventing sensor failures during autonomous flights. Our study is motivated by the lack of sensor failure datasets for UAVs. We contribute by generating the first comprehensive UAV sensor failures dataset, encompassing 70 autonomous flight data instances with over 7 h of flight time. Our Biomisa Arducopter Sensory Critique (BASiC) dataset includes various sensor failure scenarios, such as GPS, remote control, accelerometer, gyroscope, compass, and barometer. In this paper, we introduce an attention -based novel framework leveraging transformer neural network architecture for UAV sensor failure prediction and classification. The proposed framework analyzes our multivariate spatio-temporal BASiC dataset using transformers. We conducted ablation studies to evaluate transformer performance under different hyperparameter settings, demonstrating the effects of parameter tuning. Our framework achieved an average accuracy of 86% in predicting sensor failures, anticipating them 1 min and 2 s before occurrencea 30 -second improvement over the established LSTM-based (long short-term memory) approach. Additionally, our framework attained a 94% accuracy in classifying sensor failures. This performance evaluation highlights the potential of our optimized framework for integration in mission -critical UAVs, enabling real-time sensor failure prediction and classification.
更多查看译文
关键词
Sensors dataset,Autonomous flights,Attention,Deep learning,Transformer neural network,Failure prediction,Failure classification,UAV
AI 理解论文
溯源树
样例
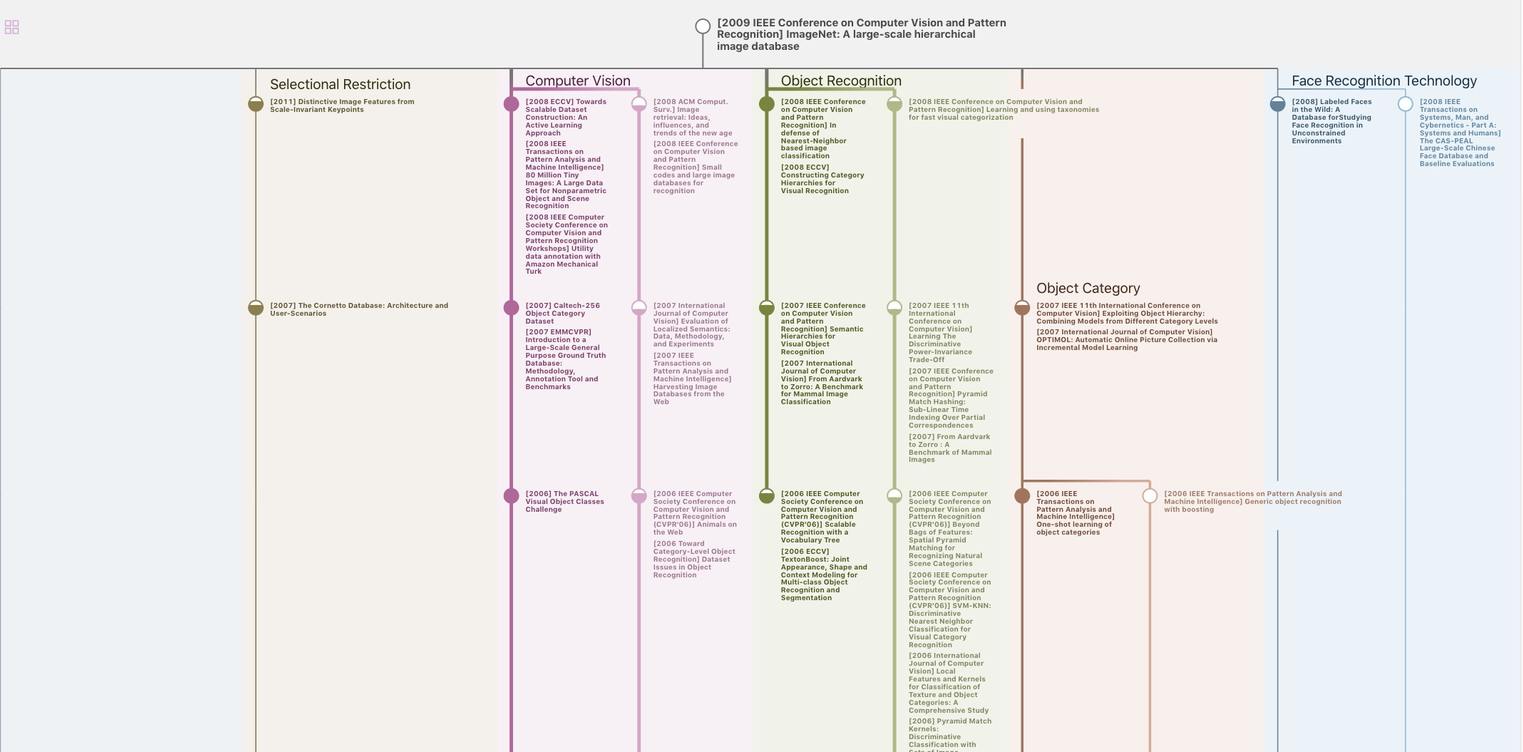
生成溯源树,研究论文发展脉络
Chat Paper
正在生成论文摘要