A novel crude oil price forecasting model using decomposition and deep learning networks
ENGINEERING APPLICATIONS OF ARTIFICIAL INTELLIGENCE(2024)
摘要
Crude oil prices are susceptible to a variety of elements, including military, political, financial, and diplomatic forces, resulting in price fluctuations that are random, abrupt, and chaotic. Based on the chaotic nature of crude oil price series, this paper proposes a model for predicting crude oil prices that incorporates variational modal decomposition algorithm (VMD), phase space reconstruction technique (PSR), convolutional neural network (CNN), and bidirectional long and short -term memory network (BiLSTM). Specifically, the noises in the original data are removed using VMD. Next, the crude oil price is reconstructed using PSR. Then the reconstructed and denoised phase space is fed into the CNN-BiLSTM model for multi -step predictions. The empirical results show that the proposed model achieves the lowest MAPEs as 0.662%, 2.283%, 3.829%, and 4.44% and the lowest MSEs as 0.335, 3.942, 10.047 and 13.681. The Diebold Mariano (DM) test also demonstrates the proposed model's superiority in the domain of crude oil price forecasting.
更多查看译文
关键词
Deep learning,Phase space reconfiguration,Variational model decomposition,Crude oil prices
AI 理解论文
溯源树
样例
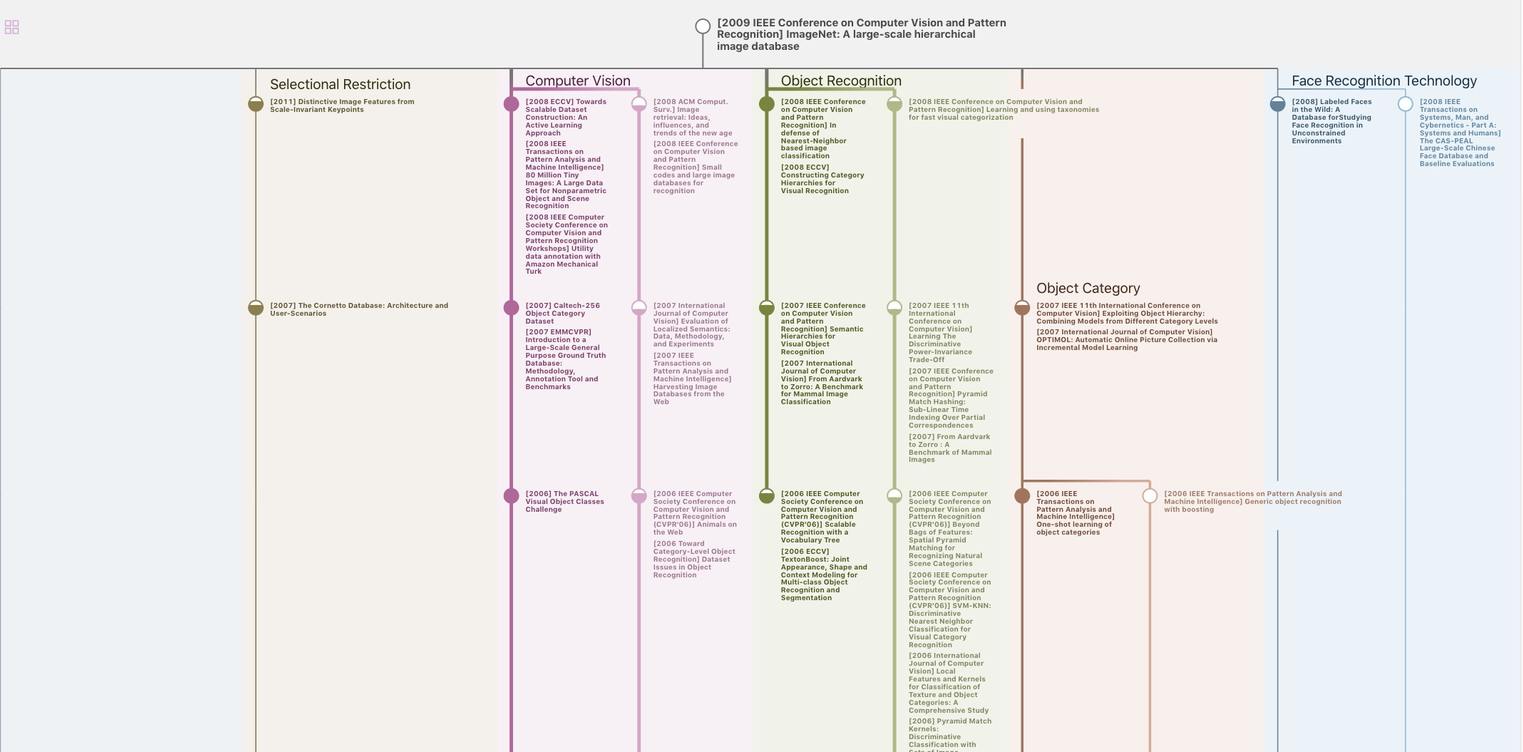
生成溯源树,研究论文发展脉络
Chat Paper
正在生成论文摘要