CVAD-GAN: Constrained video anomaly detection via generative adversarial network
Image and Vision Computing(2024)
摘要
Automatic detection of abnormal behavior in video sequences is a fundamental and challenging problem for intelligent video surveillance systems. However, the existing state-of-the-art Video Anomaly Detection (VAD) methods are computationally expensive and lack the desired robustness in real-world scenarios. The contemporary VAD methods cannot detect the fundamental features absent during training, which usually results in a high false positive rate while testing. To this end, we propose a Constrained Generative Adversarial Network (CVAD-GAN) for real-time VAD. Adding white Gaussian noise to the input video frame with constrained latent space of CVAD-GAN improves its fine-grained features learning from the normal video frames. Also, the dilated convolution layers and skip-connection preserve the information across layers to understand the broader context of complex video scenes in real-time. Our proposed approach achieves a higher Area Under Curve (AUC) score and a lower Equal Error Rate (EER) with enhanced computational efficiency than the existing state-of-the-art VAD methods. CVAD-GAN achieves an AUC and EER score of 98.0% and 6.0% on UCSD Peds1, 97.8% and 7.0% on UCSD Peds2, 94.0% and 8.1% on CUHK Avenue, and 76.2% and 21.7% on ShanghaiTech dataset, respectively. Also, it detects 63 and 19 abnormal events, with false alarms of 3 and 1, respectively, on the Subway-Entry and Subway-Exit datasets. The source code to replicate the results of the proposed CVAD-GAN is available at https://github.com/Rituraj-ksi/CVAD-GAN.
更多查看译文
关键词
Video anomaly detection,Adversarial learning,Surveillance video,Generative adversarial network (GAN)
AI 理解论文
溯源树
样例
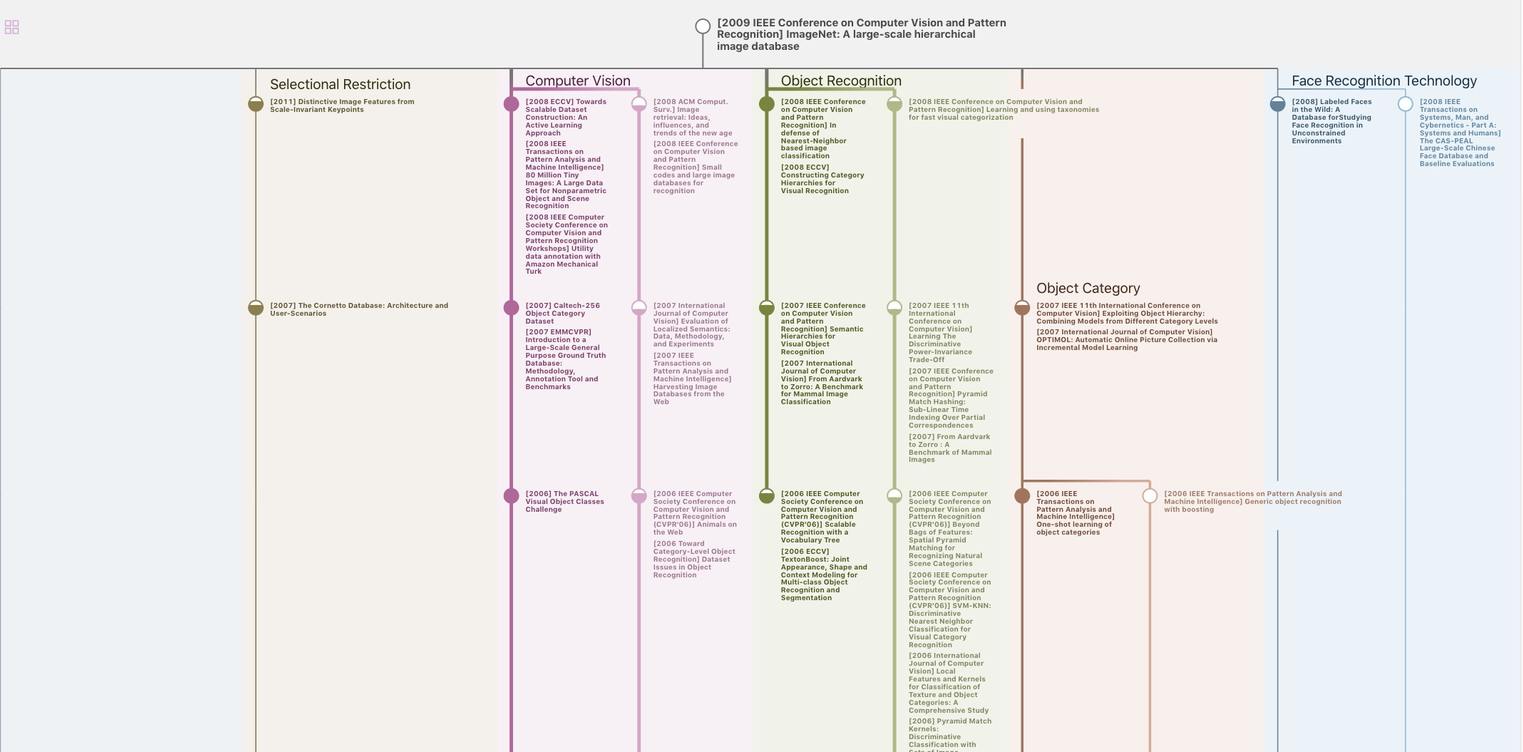
生成溯源树,研究论文发展脉络
Chat Paper
正在生成论文摘要