Safety: A spatial and feature mixed outlier detection method for big trajectory data
INFORMATION PROCESSING & MANAGEMENT(2024)
摘要
Trajectories, as sequential data records generated by continuously collecting sample points from positioning sensors, have the capability to effectively depict the motion patterns of mobile entities. The primary objective of trajectory outlier detection is to identify entities that exhibit aberrant behavior. However, outlier detection for massive trajectories still faces challenges in terms of computational power and algorithms. Specifically: 1. (Computational Power): Trajectory outlier detection, as a computationally intensive application, has encountered significant obstacles in traditional single -machine computing environments. 2. (Algorithmic Challenges): The accuracy of trajectory outlier detection is influenced by various factors. For instance, vehicle trajectories are constrained by the road network structure, which can lead to spatial similarities between abnormal and normal trajectories. To address the above issues, we propose a spatial and feature mixed outlier detection method for big trajectory data, named Safety. Our approach tackles the Computational Power challenge by designing data structures that facilitate trajectory load distribution and result correctness assurance within a distributed parallel processing engine, making it compatible with parallel processing architectures. Regarding Algorithmic Challenges, Safety utilizes feature -based detection within clusters to identify finer -grained feature anomalies, thus improving result accuracy. Extensive experiments on Beijing and Chengdu datasets show that the proposed Safety consistently outperforms existing baselines. In the distributed environment, Safety reduces latency by 29.3% and 27.94% on these two datasets, respectively. In terms of F -measure, Safety has achieved an improvement of 29.55% compared to the baseline on the Beijing dataset.
更多查看译文
关键词
Spatio-temporal data management,Distributed stream processing,Outlier information detection,Trajectory analysis
AI 理解论文
溯源树
样例
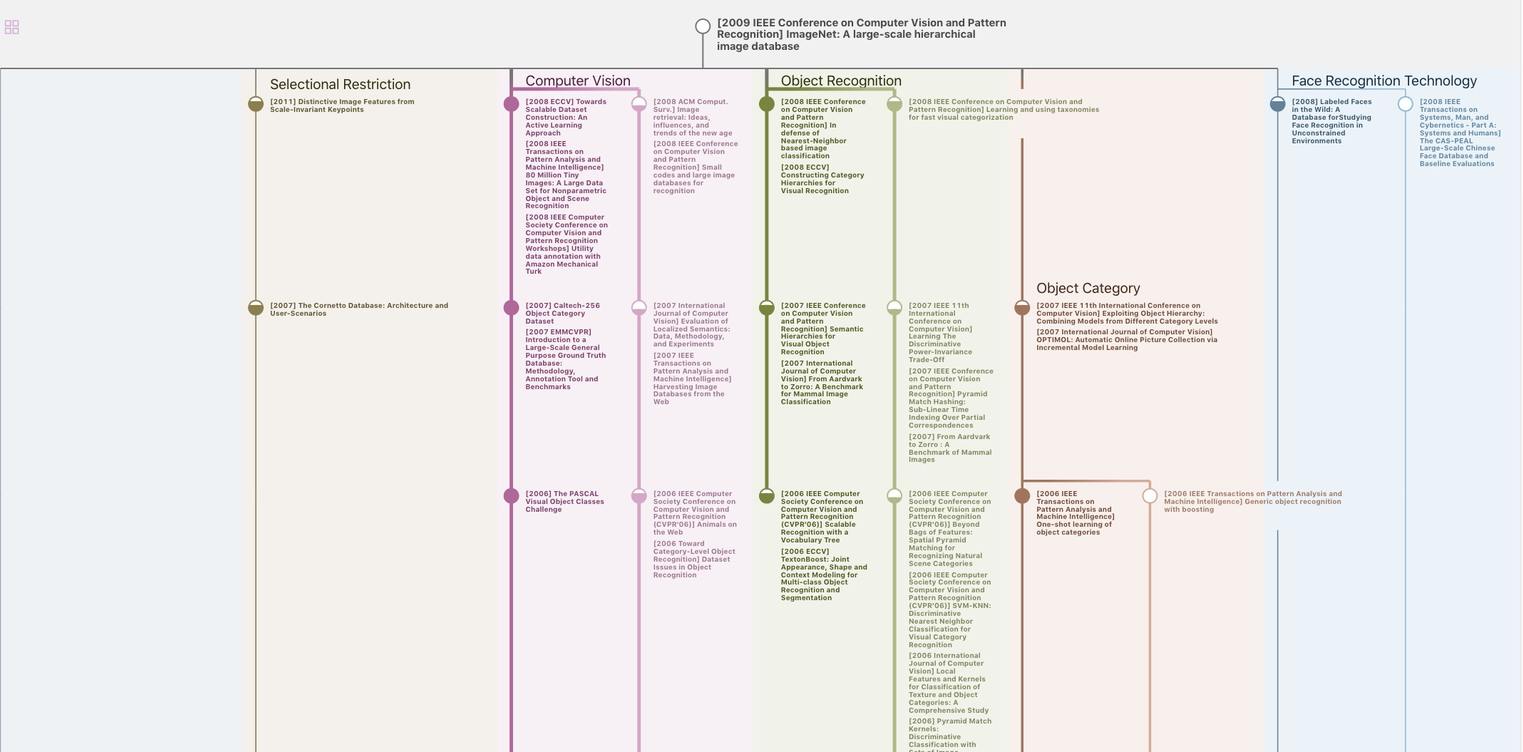
生成溯源树,研究论文发展脉络
Chat Paper
正在生成论文摘要