Enhancing mineral prospectivity mapping with geospatial artificial intelligence: A geographically neural network-weighted logistic regression approach
International Journal of Applied Earth Observation and Geoinformation(2024)
摘要
Accurate prediction of mineral resources is imperative to meet the energy demands of modern society. Nonetheless, this task is often difficult due to estimation bias and limited interpretability of conventional statistical techniques and machine learning methods. To address these shortcomings, we propose a novel geospatial artificial intelligence approach, denoted as geographically neural network-weighted logistic regression, for mineral prospectivity mapping. This model integrates spatial patterns and neural networks, combined with the Shapley additive explanations theory to achieve accurate forecasts and provide explainable insight into mineralization within intricate spatial contexts. In a gold prospecting experiment conducted in Nova Scotia, our model outperformed other state-of-the-art models with a 5% to 16% increase in the area under the receiver operating characteristic curve metric. The presented framework further provided intuitive quantifications of the impact of geological factors on the gold mineralization in spatial settings. The innovative approach promotes novel phenomenon detection and exhibits robust capabilities and universality for classification problems within complex spatial scenarios.
更多查看译文
关键词
Mineral prospectivity mapping,Geospatial artificial intelligence (GeoAI),Spatial heterogeneity,Explainability,Machine learning
AI 理解论文
溯源树
样例
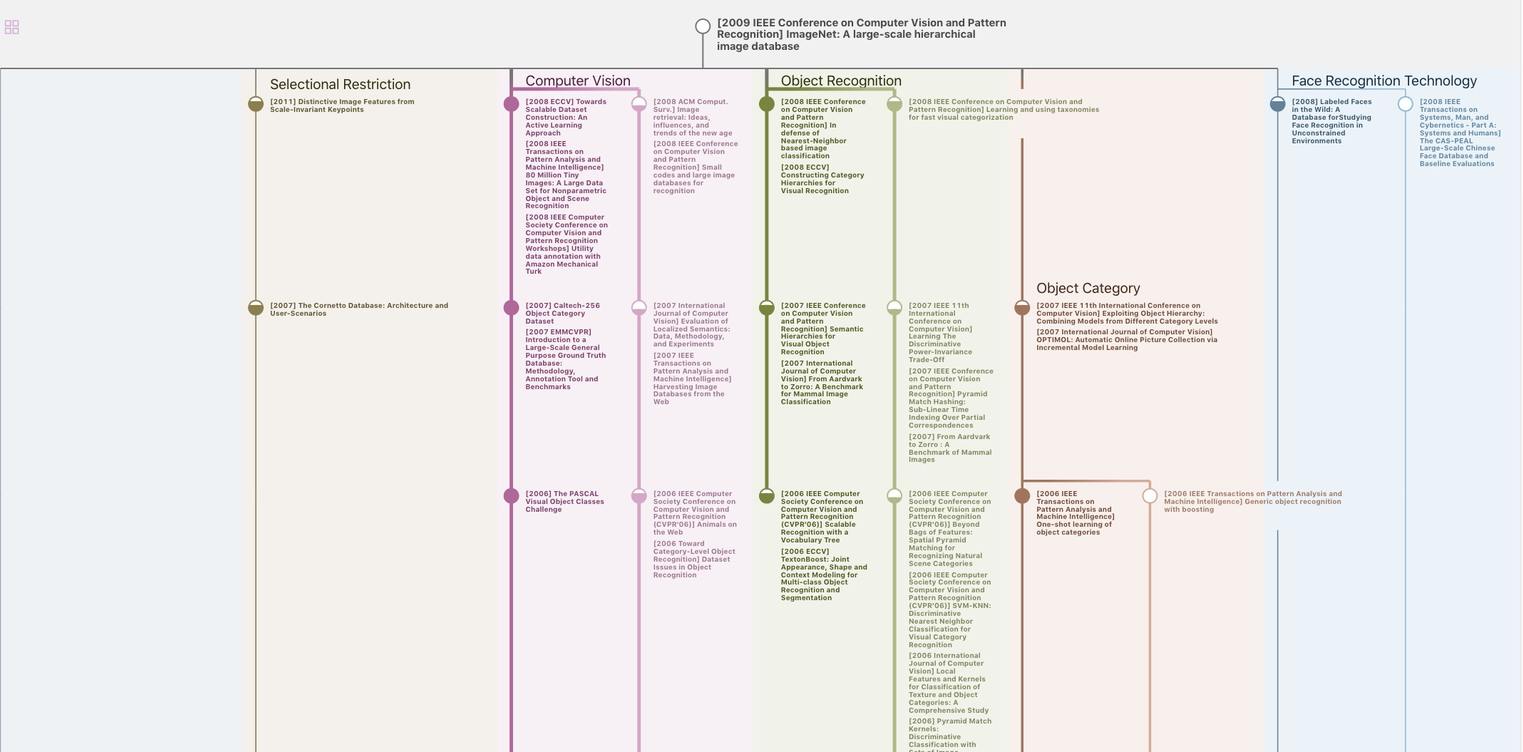
生成溯源树,研究论文发展脉络
Chat Paper
正在生成论文摘要