Fast and robust clustering of general-shaped structures with tk-merge
INTERNATIONAL JOURNAL OF APPROXIMATE REASONING(2024)
摘要
In real -world applications, the group of provenance of data can be inherently uncertain, the data values can be imprecise and some of them can be wrong. We handle uncertain, imprecise and noisy data in clustering problems with general -shaped structures. We do it under very weak parametric assumptions with a two-step hybrid robust clustering algorithm based on trimmed k -means and hierarchical agglomeration. The algorithm has low computational complexity and effectively identifies the clusters also in presence of data contamination. We also present natural generalizations of the approach as well as an adaptive procedure to estimate the amount of contamination in a data -driven fashion. Our proposal outperforms state-of-the-art robust, model -based methods in our numerical simulations and real -world applications related to color quantization for image analysis, human mobility patterns based on GPS data, biomedical images of diabetic retinopathy, and weather data.
更多查看译文
关键词
Robust statistics,Model-based clustering,Hierarchical clustering
AI 理解论文
溯源树
样例
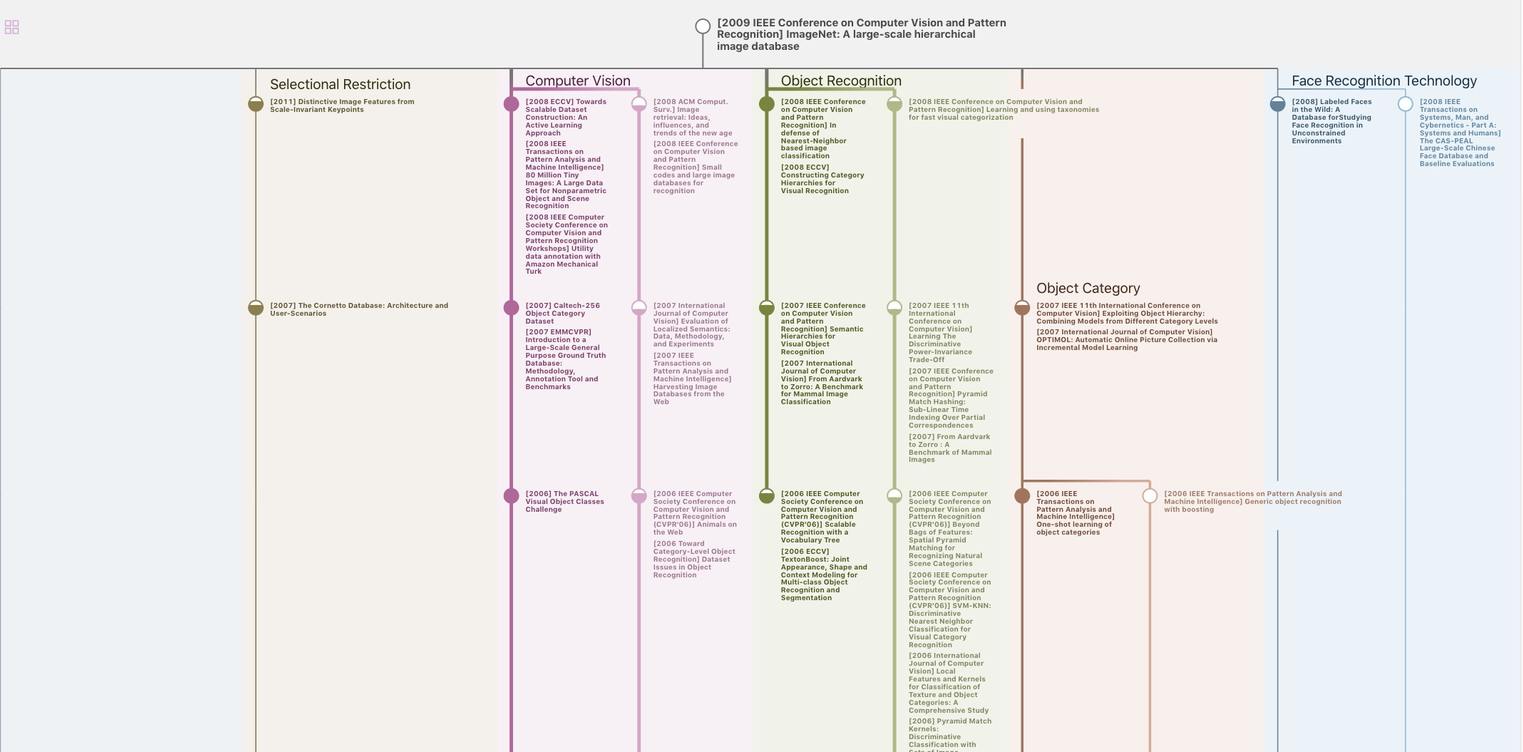
生成溯源树,研究论文发展脉络
Chat Paper
正在生成论文摘要