A multi-scale information fusion-based multiple correlations for unsupervised attribute selection
INFORMATION FUSION(2024)
摘要
With the continuous evolution of artificial intelligence and sensor technology, there is a growing accumulation of unlabeled data. Uncovering valuable insights from this data has become a crucial task, propelling the ongoing development of unsupervised knowledge discovery techniques. Unsupervised attribute selection plays a pivotal role in data preprocessing for knowledge discovery, aiming to extract relevant attributes from the dataset without relying on prior labeling or category information. This article introduces an unsupervised attribute selection method that integrates multi-scale information while considering multiple correlations. Initially, a multi-scale fuzzy similarity relation information system (MsFSRIS) is established by integrating multi-scale information with fuzzy relations. This system unveils fuzzy similarity relations stemming from both numerical and categorical attributes, while also integrating information across multiple scales. Within this MsFSRIS, information measures of fuzzy multi-scale are defined, encompassing multiple correlations related to multi-scale fuzzy relevance, multi-scale fuzzy redundancy, multi-scale fuzzy complementarity, and multi-scale fuzzy interaction during the attribute selection process. Furthermore, we propose an unsupervised attribute selection algorithm (UAS_MsIFMC) that fuses information from multiple scales and considers multiple interactions among selected, unselected, and candidate attributes, enhancing the identification of valuable attributes. Finally, the experimental results demonstrate the superiority of the proposed UAS_MsIFMC algorithm, presenting a promising solution for unsupervised attribute selection methods.
更多查看译文
关键词
Multi-scale information fusion,Fuzzy similarity relation information system,Information measures,Multiple correlations,Unsupervised attribute selection
AI 理解论文
溯源树
样例
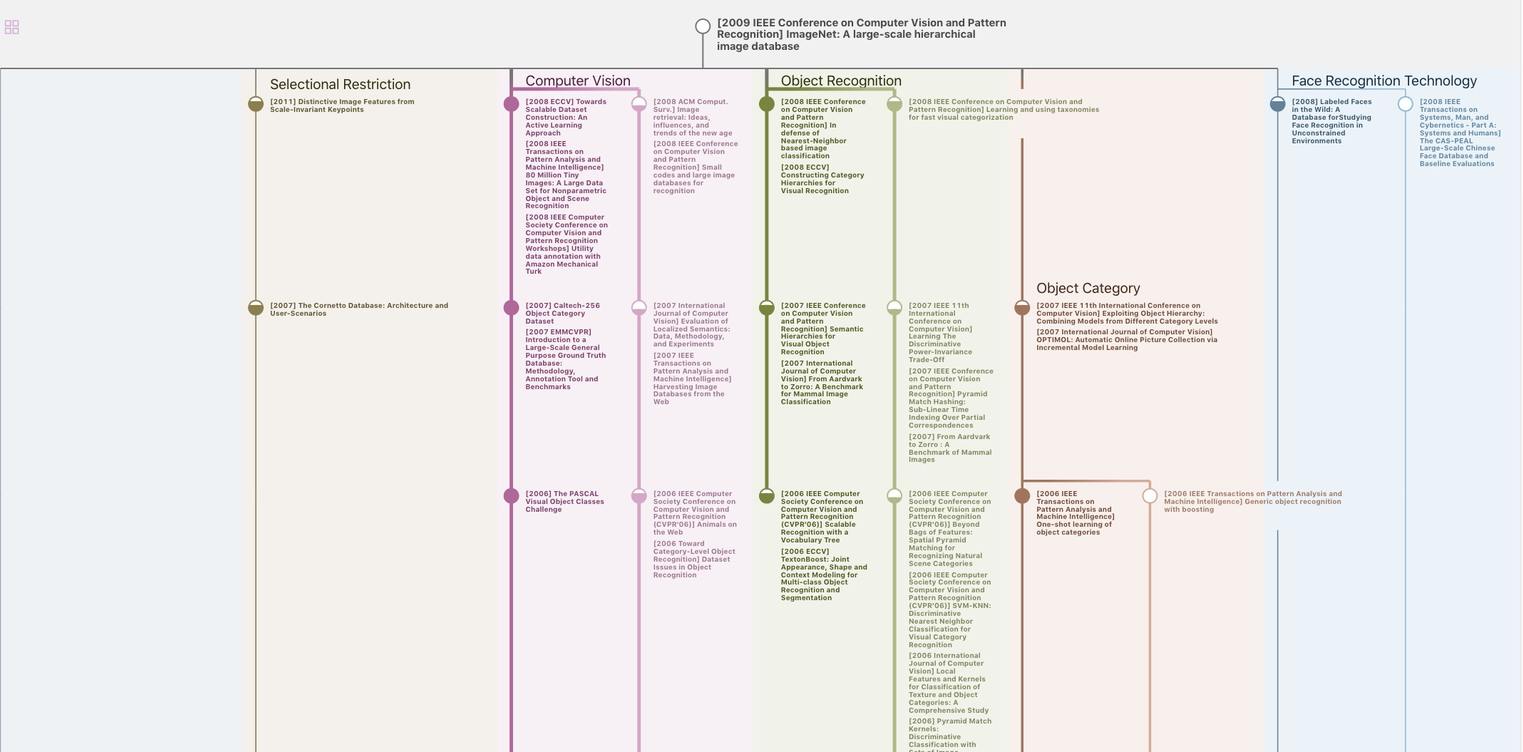
生成溯源树,研究论文发展脉络
Chat Paper
正在生成论文摘要